Projection Based Constrained Policy Optimization
international conference on learning representations(2020)
摘要
In this paper, we consider the problem of learning control policies that optimize areward function while satisfying constraints due to considerations of safety, fairness, or other costs. We propose a new algorithm - Projection Based ConstrainedPolicy Optimization (PCPO), an iterative method for optimizing policies in a two-step process - the first step performs an unconstrained update while the secondstep reconciles the constraint violation by projection the policy back onto the constraint set. We theoretically analyze PCPO and provide a lower bound on rewardimprovement, as well as an upper bound on constraint violation for each policy update. We further characterize the convergence of PCPO with projection basedon two different metrics - L2 norm and Kullback-Leibler divergence. Our empirical results over several control tasks demonstrate that our algorithm achievessuperior performance, averaging more than 3.5 times less constraint violation andaround 15% higher reward compared to state-of-the-art methods.
更多查看译文
关键词
Reinforcement learning with constraints, Safe reinforcement learning
AI 理解论文
溯源树
样例
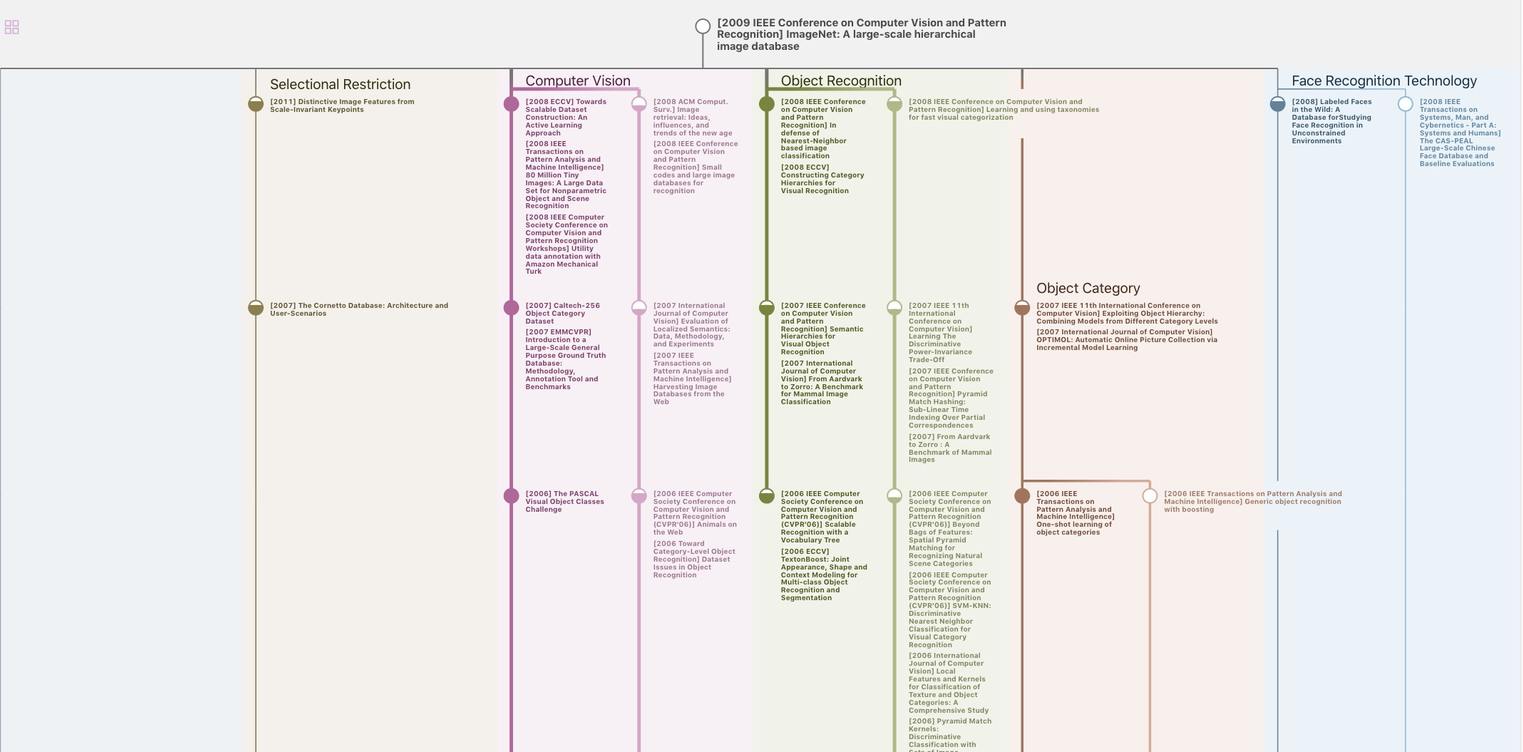
生成溯源树,研究论文发展脉络
Chat Paper
正在生成论文摘要