A sparse linear regression model for incomplete datasets
Pattern Analysis and Applications(2019)
摘要
Incomplete data are often neglected when designing machine learning methods. A popular strategy adopted by practitioners to circumvent this consists of taking a preprocessing step to fill the missing components. These preprocessing algorithms are designed independently of the machine learning method that will be applied subsequently, which may lead to sub-optimal results. An alternative solution is to redesign classical machine learning methods to handle missing data directly. In this paper, we propose a variant of the forward stagewise regression (FSR) algorithm for incomplete data. The original FSR is an iterative procedure to estimate parameters of sparse linear models. The proposed method, named forward stagewise regression for incomplete datasets with GMM (FSIG), models the missing components as random variables following a Gaussian mixture distribution. In FSIG, the main steps of FSR are adapted to deaç with the intrinsic uncertainty of incomplete samples. The performance of FSIG was evaluated in an extensive set of experiments, and our model was able to outperform classical methods in most of the tested cases.
更多查看译文
关键词
Forward stagewise regression,Missing data,Gaussian mixtures
AI 理解论文
溯源树
样例
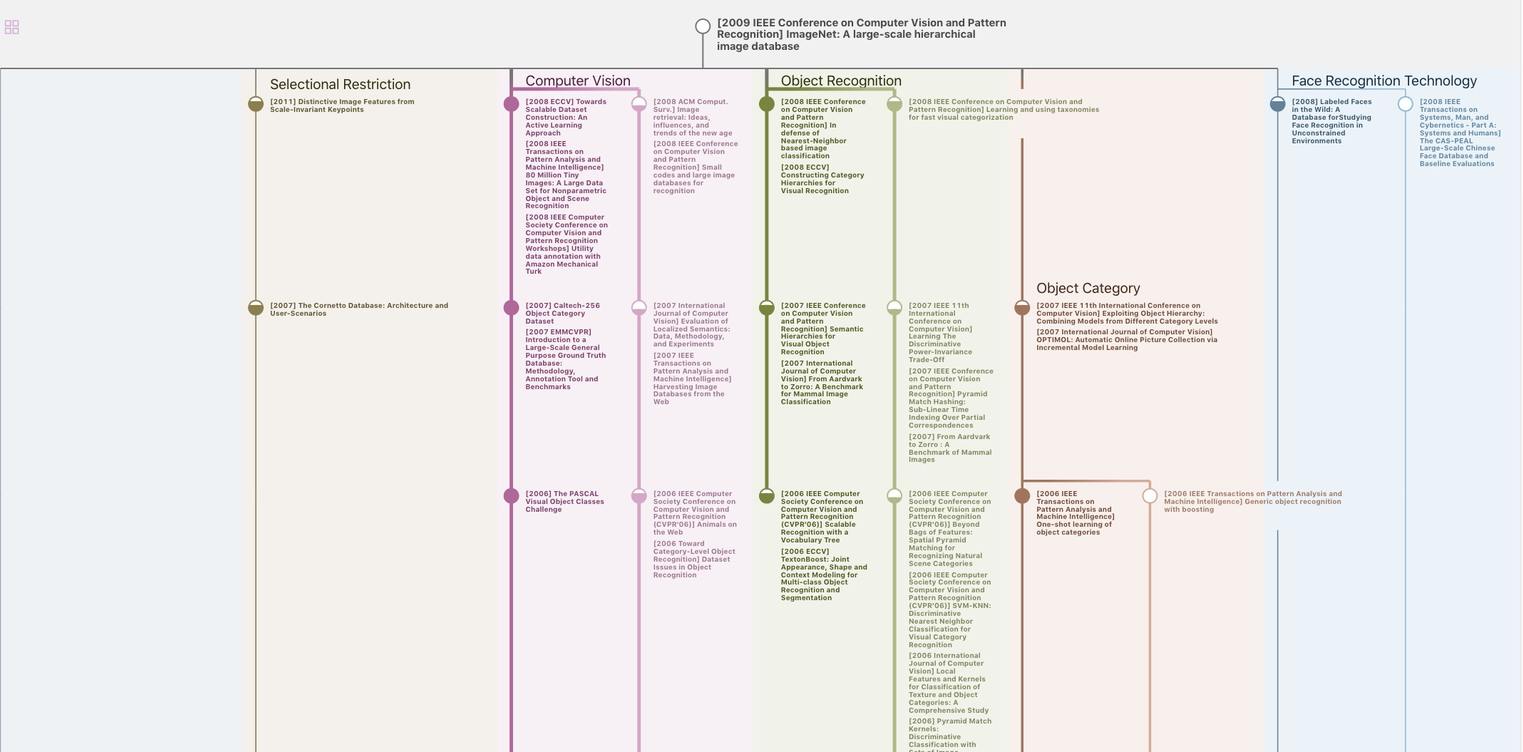
生成溯源树,研究论文发展脉络
Chat Paper
正在生成论文摘要