Performance Analysis Of Spiking Rbm With Measurement-Based Phase Change Memory Model
NEURAL INFORMATION PROCESSING, ICONIP 2019, PT V(2019)
摘要
Phase change memory (PCM) is arguably one of the most promising non-volatile memories which can be used in neuromorphic applications. When we use PCM as analog synaptic elements, non-ideality impact should be carefully taken into account. In this paper, we investigate the impact of such nonideality items as nonlinear weight update and resistance drift on training accuracy in a spiking restricted Boltzmann machine (RBM). In addition to ideal PCM model, actual measurement-based PCM characteristics is used for this study. The resistance drift affects training accuracy, especially in a widely distributed read interval time caused by spatial and temporal sparse spike activities during training in spiking RBM. Our simulation results show that the training accuracy worsens with the increase of the weight-update nonlinearity or the resistance-drift coefficient. However, the results also suggest we can expect more than 8.95% improvement in training accuracy. This potential improvement will be possible if we use linearity-improved confined PCM cells whose median resistance drift coefficient is 0.005, compared to the resistance drift coefficient of more than 0.02 in typical existing PCM cells.
更多查看译文
关键词
Spiking neural network, Phase change memory, Resistance drift
AI 理解论文
溯源树
样例
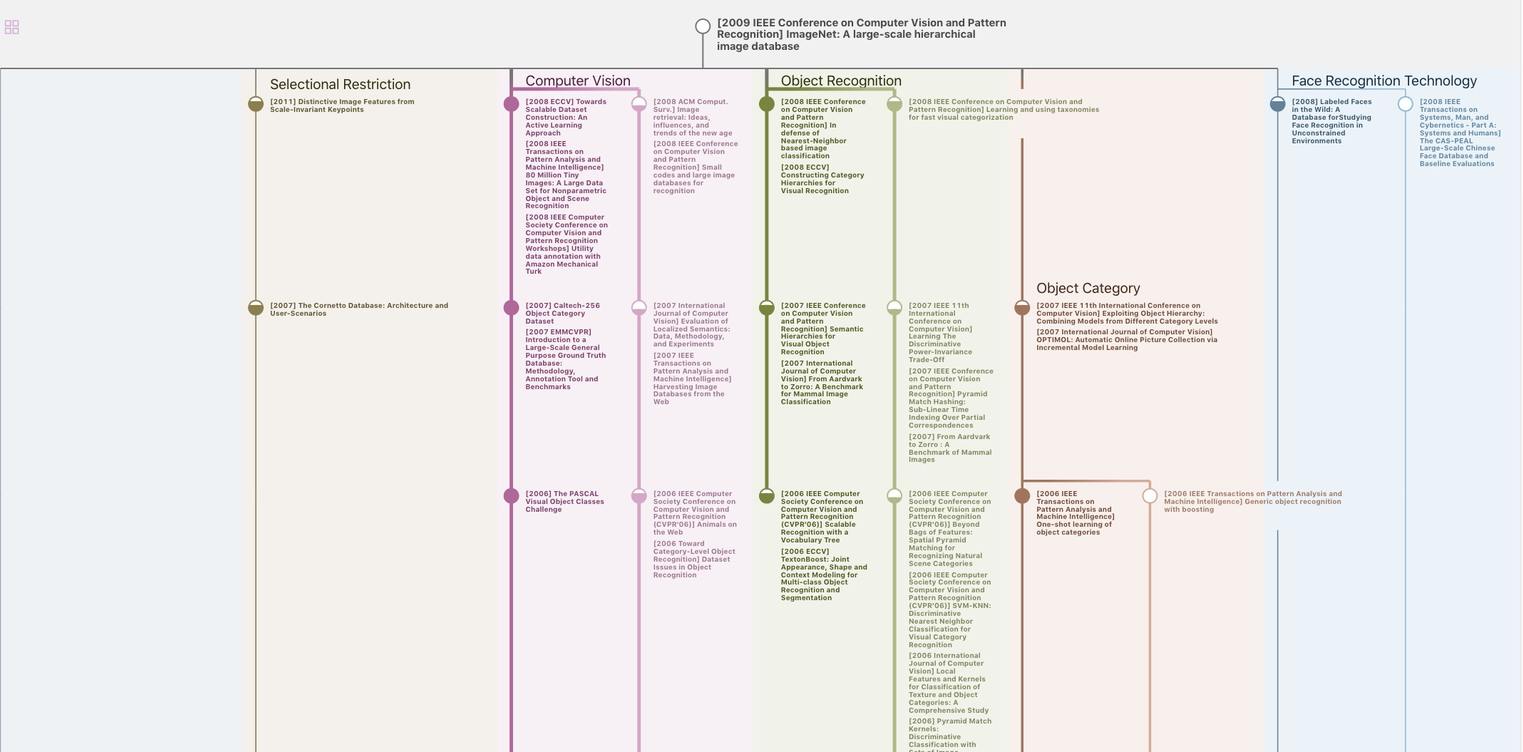
生成溯源树,研究论文发展脉络
Chat Paper
正在生成论文摘要