Cnn-Lstm Neural Networks For Anomalous Database Intrusion Detection In Rbac-Administered Model
NEURAL INFORMATION PROCESSING (ICONIP 2019), PT IV(2019)
摘要
The relational database is designed to store and process large amount of information such as business records and personal data. There are many policies and access control techniques for database security, but they are not sufficient for detecting insider attacks. In order to detect threats for the database application, it is necessary to adopt role-based access control (RBAC) and classify the roles according to the authority of each user. In this paper, we propose a method of classifying user's role and authority using the CNN-LSTM neural networks by extracting features from SQL queries. In the anomaly detection method, CNN automatically extracts important features from database query and LSTM models the temporal information of the SQL sequence. The class activation map also identifies the SQL query features that affect the classification. Experiments with the TPC-E scenario-based benchmark query dataset show that the CNN-LSTM neural networks surpass other state-of-the-art machine learning methods, achieving an overall accuracy of 93.3% and recall of 88.7%. We also identify the characteristics of misclassification data through statistical analysis.
更多查看译文
关键词
Deep learning, Convolutional neural network, Long short-term memory, Database security, Access control
AI 理解论文
溯源树
样例
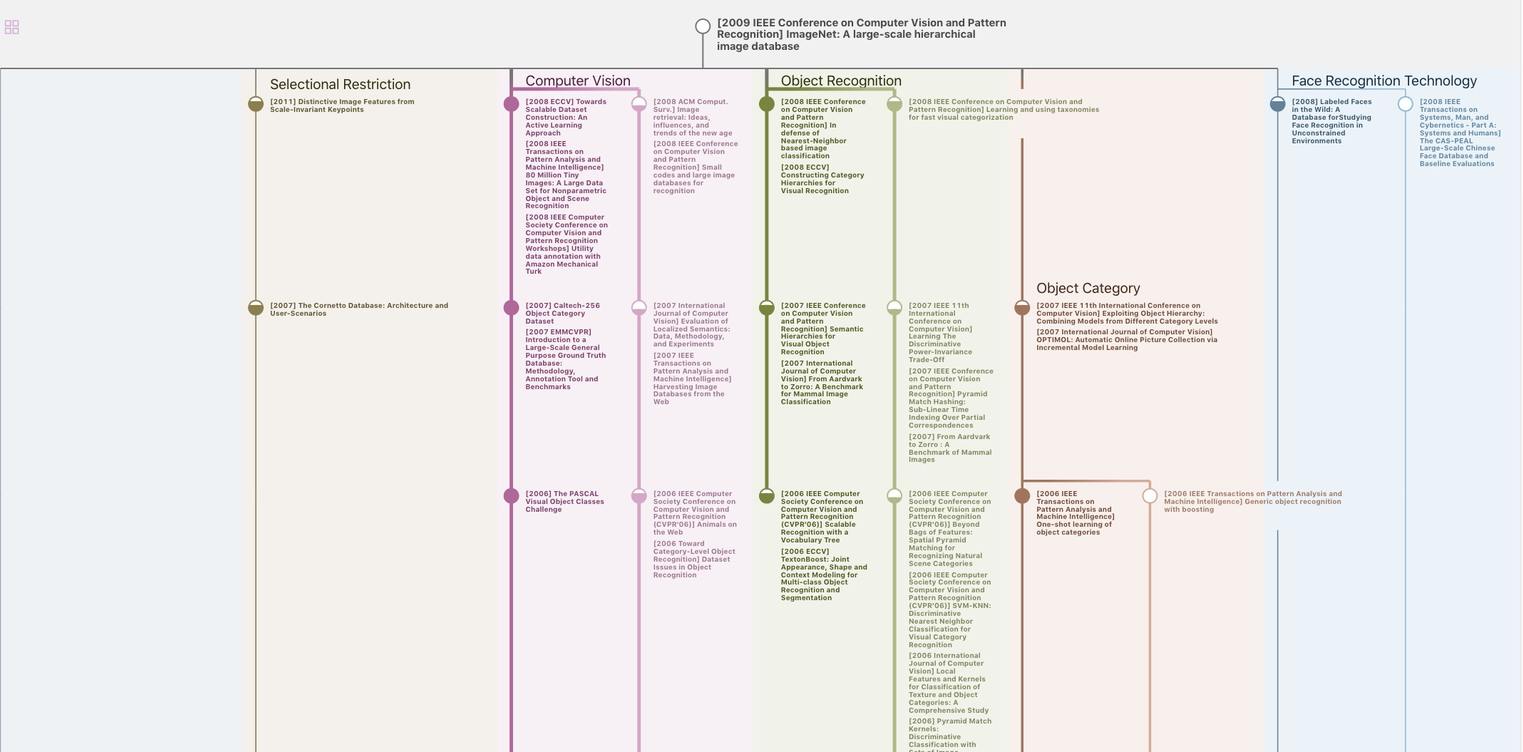
生成溯源树,研究论文发展脉络
Chat Paper
正在生成论文摘要