Interactive Semantic Features Selection From Reviews For Recommendation
NEURAL INFORMATION PROCESSING, ICONIP 2019, PT V(2019)
摘要
In recent years, reviews information has been effectively utilized by deep learning to improve the performance of the recommendation system and alleviate the problems of sparse data and cold start. However, there is much redundant information in the reviews that has a negative effect on the performance of the recommender system, which is ignored by most existing methods. In this paper, the Interactive Semantic Features Selection (ISFS) method is proposed to more effectively select the useful information from reviews based on attention mechanisms. Specifically, each word in reviews is interactively assigned a different weight according to the value of the semantic information it contains. Experiment results on real-world datasets show that ISFS outperforms baseline recommender systems on rating prediction tasks.
更多查看译文
关键词
Recommender system, Deep learning, Attention mechanism, Long-Short Term Memory, Rating prediction
AI 理解论文
溯源树
样例
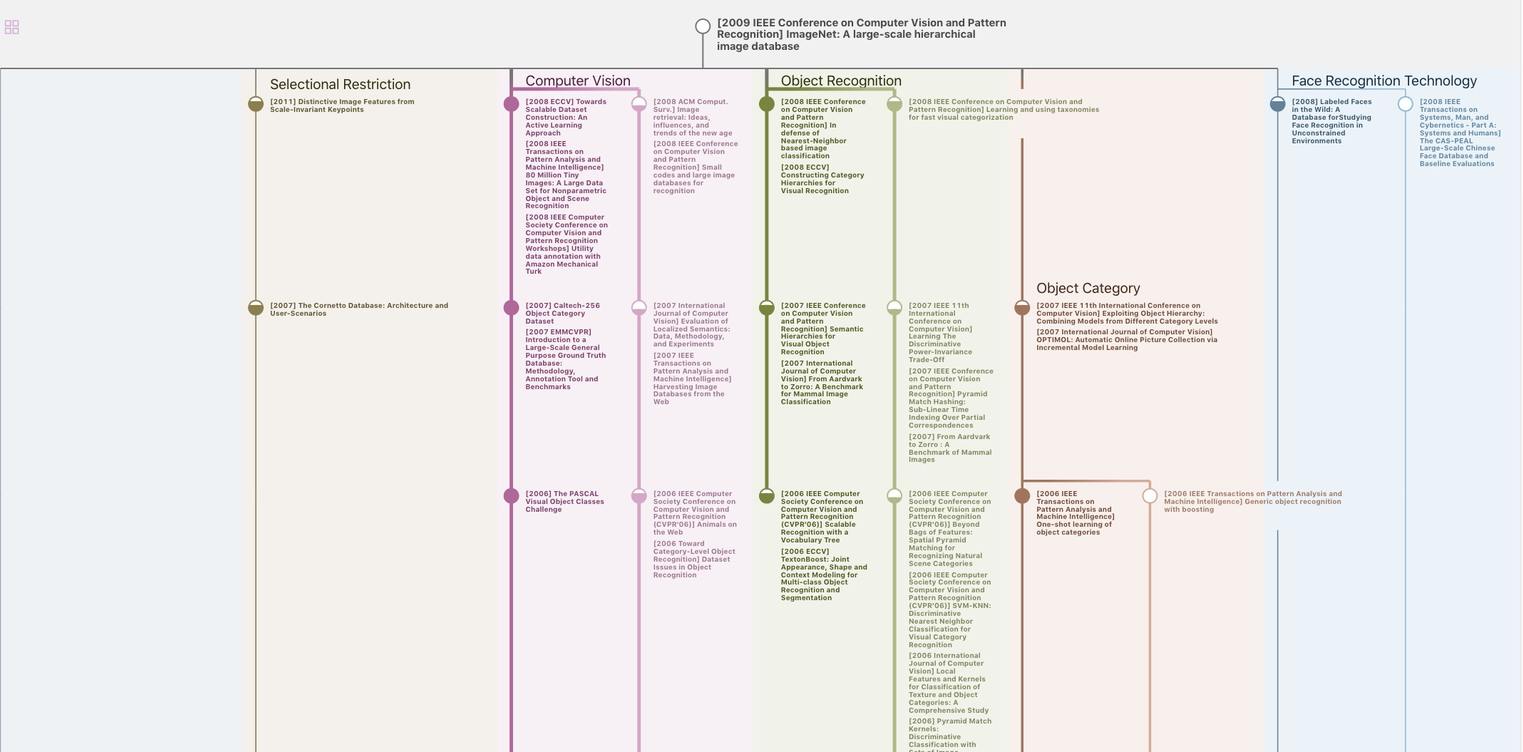
生成溯源树,研究论文发展脉络
Chat Paper
正在生成论文摘要