Graph convolutional neural network for multi-scale feature learning
Computer Vision and Image Understanding(2020)
摘要
Automatic deformable 3D modeling is computationally expensive, especially when considering complex position, orientation and scale variations. We present a volume segmentation framework to utilize local and global regularizations in a data-driven approach. We introduce automated correspondence search to avoid manually labeling landmarks and improve scalability. We propose a novel marginal space learning technique, utilizing multi-resolution pooling to obtain local and contextual features without training numerous detectors or excessively dense patches. Unlike conventional convolutional neural network operators, graph-based operators allow spatially related features to be learned on the irregular domain of the multi-resolution space, and a graph-based convolutional neural network is proposed to learn representations for position and orientation classification. The graph-CNN classifiers are used within a marginal space learning framework to provide efficient and accurate shape pose parameter hypothesis prediction. During segmentation, a global constraint is initially non-iteratively applied, with local and geometric constraints applied iteratively for refinement. Comparison is provided against both classical deformable models and state-of-the-art techniques in the complex problem domain of segmenting aortic root structure from computerized tomography scans. The proposed method shows improvement in both pose parameter estimation and segmentation performance.
更多查看译文
关键词
Deep learning,Graph convolutional neural network,Medical image segmentation,Marginal space learning,Aortic root,Computerized tomography
AI 理解论文
溯源树
样例
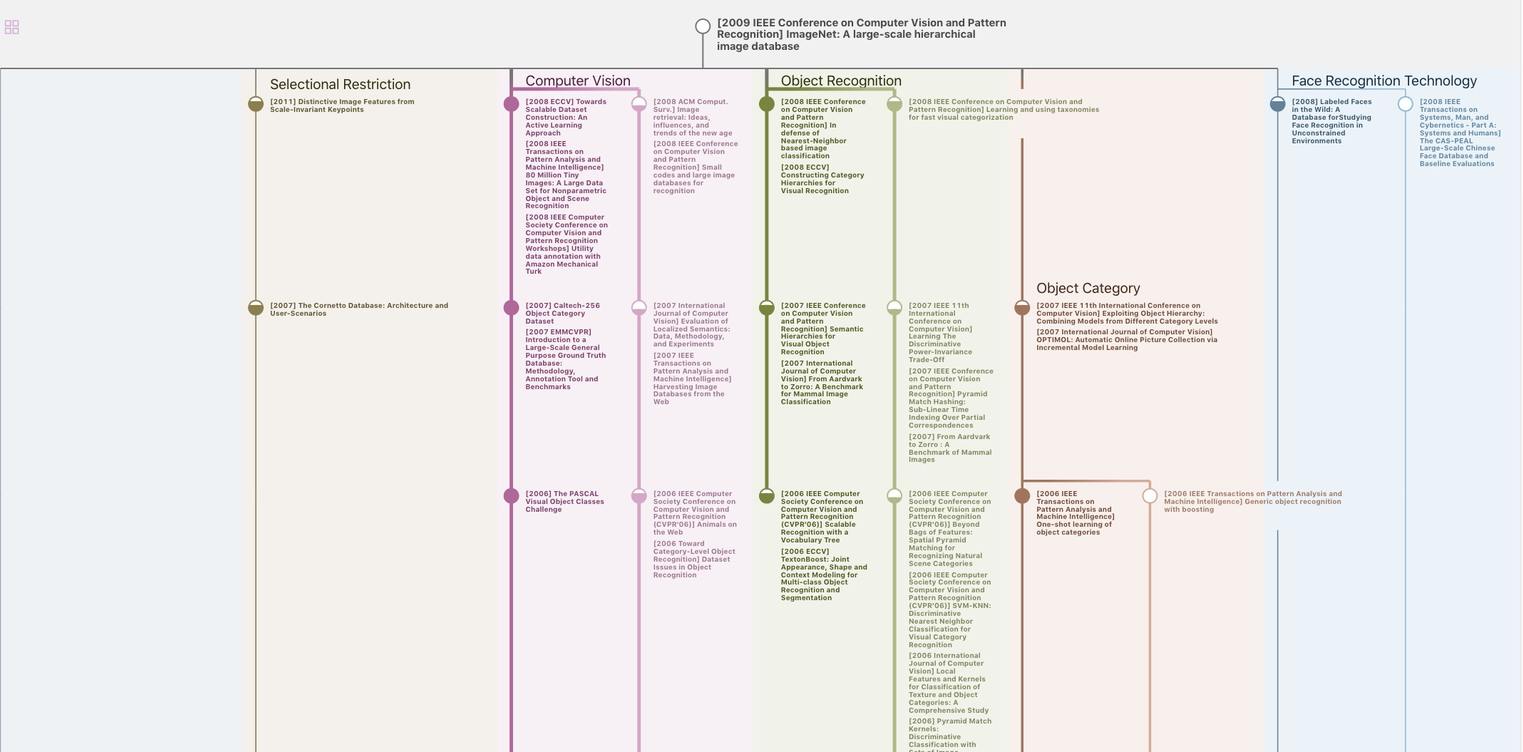
生成溯源树,研究论文发展脉络
Chat Paper
正在生成论文摘要