Learnable Gabor Convolutional Networks
NEURAL INFORMATION PROCESSING (ICONIP 2019), PT IV(2019)
摘要
Commonly used convolutional operation does not have the ability to learn invariant information of images. However, some hand-crafted image feature extractors, like Gabor wavelets, are robust to object's scale and orientation transformations. Hence, how to combine Gabor filters with convolutional kernels for image feature extraction is an interesting and urgent issue in recent research of image representation learning using deep convolutional neural networks (DCNNs). In this paper, we propose a new method, named learnable Gabor convolutional networks (LGCNs), to combine the Gabor filters and convolutional kernels together to form the Gabor convolutional filters (GCFs) for invariant information learning of images. The scale and orientation parameters in the Gabor function can be learned simultaneously with other parameters during the networks' training. Experimental results show that LGCNs perform better than the corresponding DCNNs and other related methods in image classification tasks.
更多查看译文
关键词
Gabor wavelets, Deep convolutional neural networks, Invariant information, Gabor convolutional filters, Image classification
AI 理解论文
溯源树
样例
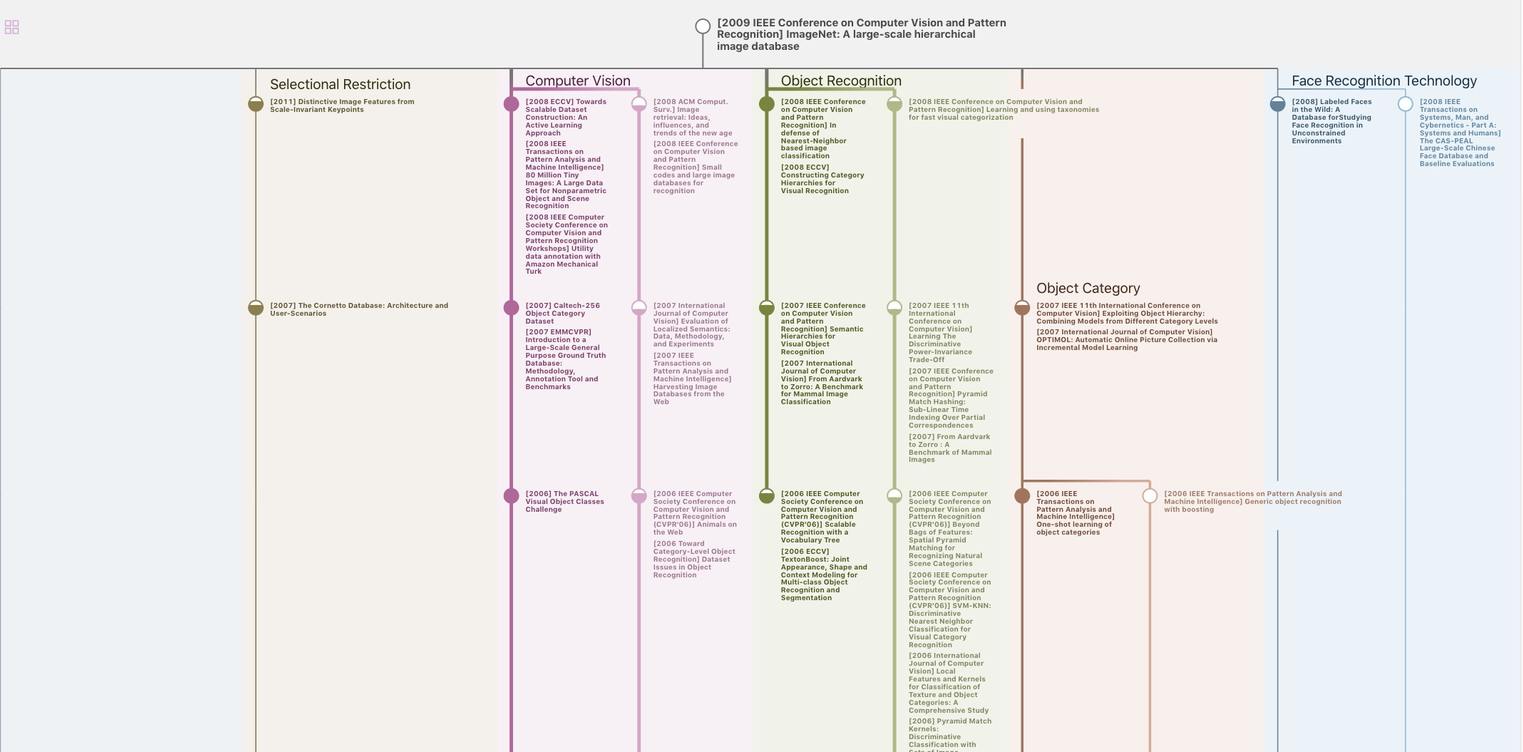
生成溯源树,研究论文发展脉络
Chat Paper
正在生成论文摘要