Joint Framework For Image Fusion A Super-Resolution Via Multicomponent Analysis And Residual Compensation
IEEE ACCESS(2019)
摘要
To solve the problems in two-step processing of image fusion and Super-Resolution Reconstruction (SRR), we propose a joint framework of image Fusion and Super-Resolution (FSR) based on multicomponent analysis and residual compensation. Inspired by the idea of multicomponent analysis, we design a new structure-texture decomposition model to realize multicomponent dictionary learning for the above task. To depict the relationship between low-resolution image and its corresponding high-resolution image, the correlation of their sparse coding coefficients is introduced in the model. To compensate the information loss during the SRR, we propose a reconstruction residue compensation mechanism, in which the reconstruction residual error is compensated into the initial result of FSR to improve the quality of the final processing result. In addition, we propose different fusion algorithms for structure and texture components. For structure components, the fusion rule with the maximum absolute value is adopted, and for texture components, we design a new saliency measure to construct fusion results. Experiment results show that the proposed method can well retain the brightness and detail information in the original image, and is superior to other methods in subjective and objective evaluation.
更多查看译文
关键词
Multicomponent analysis, sparse representation, image fusion, super-resolution reconstruction, residual compensation
AI 理解论文
溯源树
样例
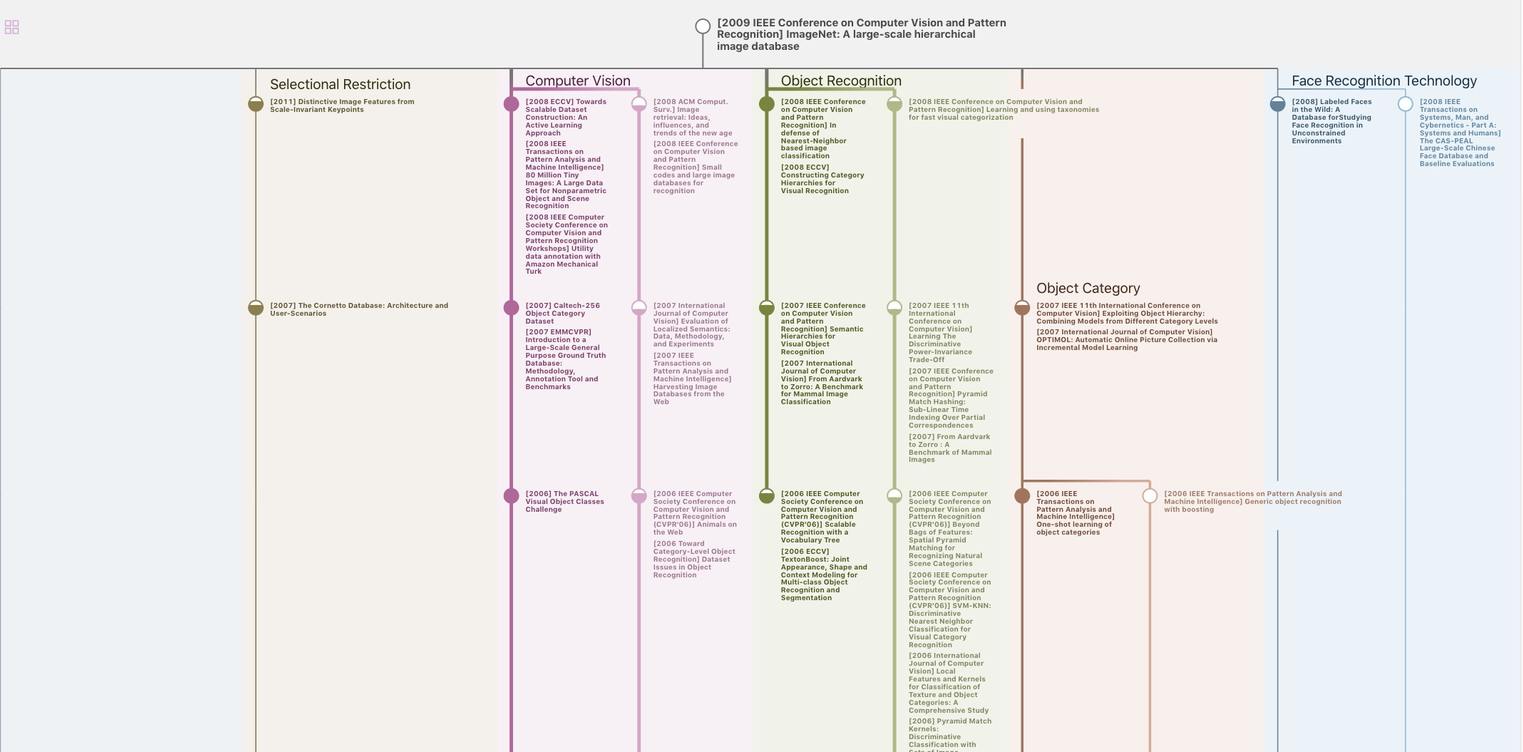
生成溯源树,研究论文发展脉络
Chat Paper
正在生成论文摘要