A Cascade Face Spoofing Detector Based On Face Anti-Spoofing R-Cnn And Improved Retinex Lbp
IEEE ACCESS(2019)
摘要
In consideration of secure and convenient, face gains increasing attention in variety of fields during the past decades. Since human face is most accessible from our daily life and preserves the richest information, face based biometric systems are widely used in person authentication applications. However, face recognition systems are always challenged by face spoofing attacks. Although, researchers have proposed many face spoofing detection methods, which have achieved great performances, we aim to develop a method to counter face spoofing, which combines the face detection stage and face spoofing detection stage together. In this paper, we design face anti-spoofing region-based convolutional neural network (FARCNN), based on improved Faster region-based convolutional neural network (R-CNN) framework. Motivated by face detection, we regard the face spoofing detection as a three-way classification to distinguish real face, fake face and background. We extend the typical Faster R-CNN scheme by optimizing several important strategies, including roi-pooling feature fusion and adding Crystal Loss function to the original multi-task loss function. In addition, an improved Retinex based LBP is presented to handle the different illumination conditions in face spoofing detection. Finally, these two detectors are further cascaded and achieve promising performances on the benchmark databases: CASIA-FASD, REPLAY-ATTACK and OULU-NPU. Besides, for the purpose of verifying the generalization capacity of the proposed cascade detector, we perform experiments on cross-databases and the results testify the effectiveness of our proposed method.
更多查看译文
关键词
Face, Feature extraction, Detectors, Lighting, Image quality, Face detection, Image color analysis, Face spoofing detection, faster R-CNN, crystal loss, Retinex, attention fusion, guided filter
AI 理解论文
溯源树
样例
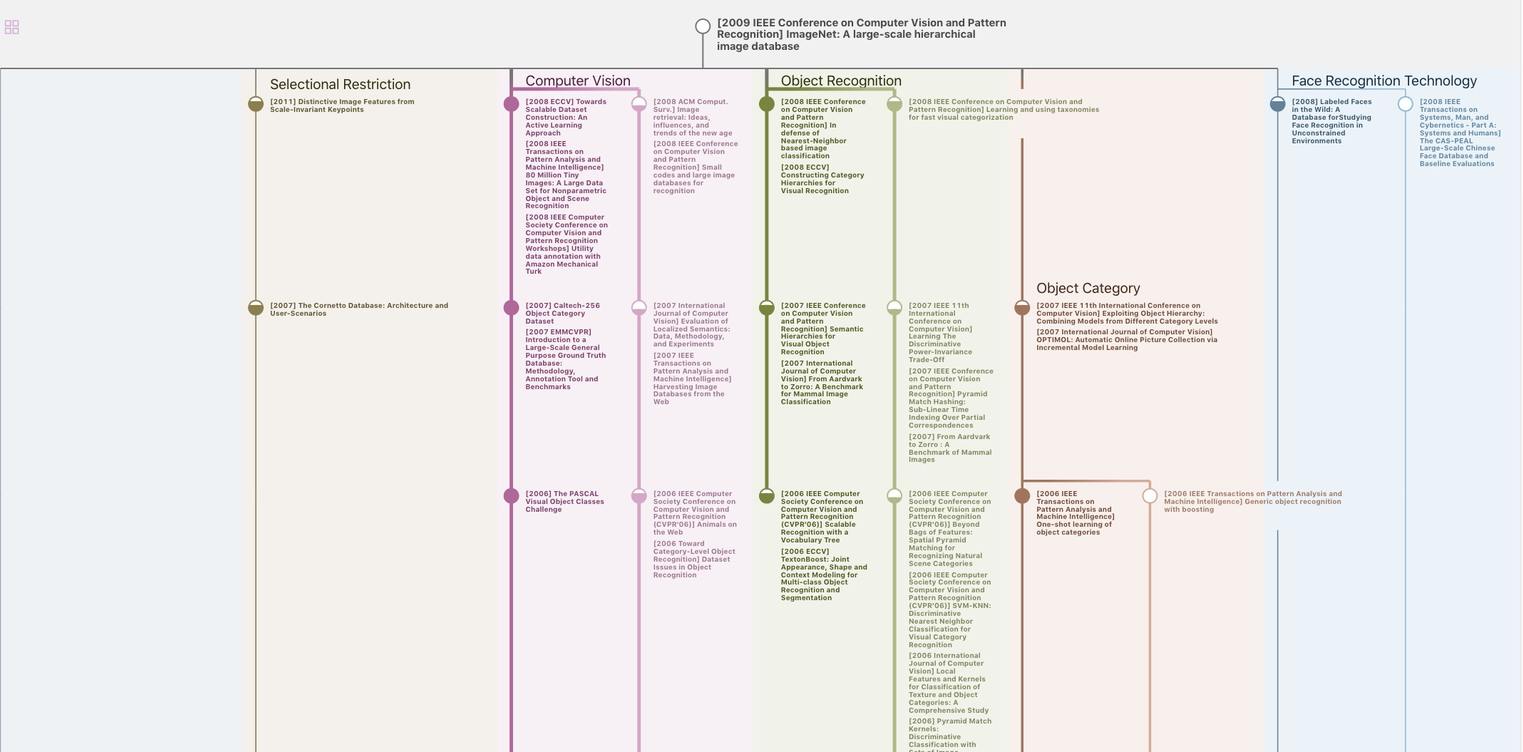
生成溯源树,研究论文发展脉络
Chat Paper
正在生成论文摘要