Generalized Pareto Model Based On Particle Swarm Optimization For Anomaly Detection
IEEE ACCESS(2019)
摘要
Anomaly detection of time series has been widely used in various fields. Most detection methods depend either on assumptions about data distribution or manual threshold setting. If the assumption is incorrect, the effectiveness of detection technology will be greatly reduced. To deal with this problem, we propose a maximum likelihood estimation method based on particle swarm optimization for generalized Pareto model to detect outliers of time series, which can be called Generalized Pareto Model Based on Particle Swarm Optimization (GPMPSO). Because the generalized Pareto model is multidimensional, we introduce a comprehensive learning strategy to improve search ability of particle swarm algorithm. Due to the multiple peaks of the log-likelihood function of generalized Pareto model, we apply dynamic neighbors to reduce the possibility of particle swarm optimization falling into local optimum. Moreover, we propose a new processing model Big Drift Streaming Peak Over Threshold (BDSPOT) to enhance the capability of the data stream processor. Our algorithm is tested on various real-world datasets which demonstrate its very competitive performance.
更多查看译文
关键词
Anomaly detection, generalized pareto distribution, particle swarm optimization, time series
AI 理解论文
溯源树
样例
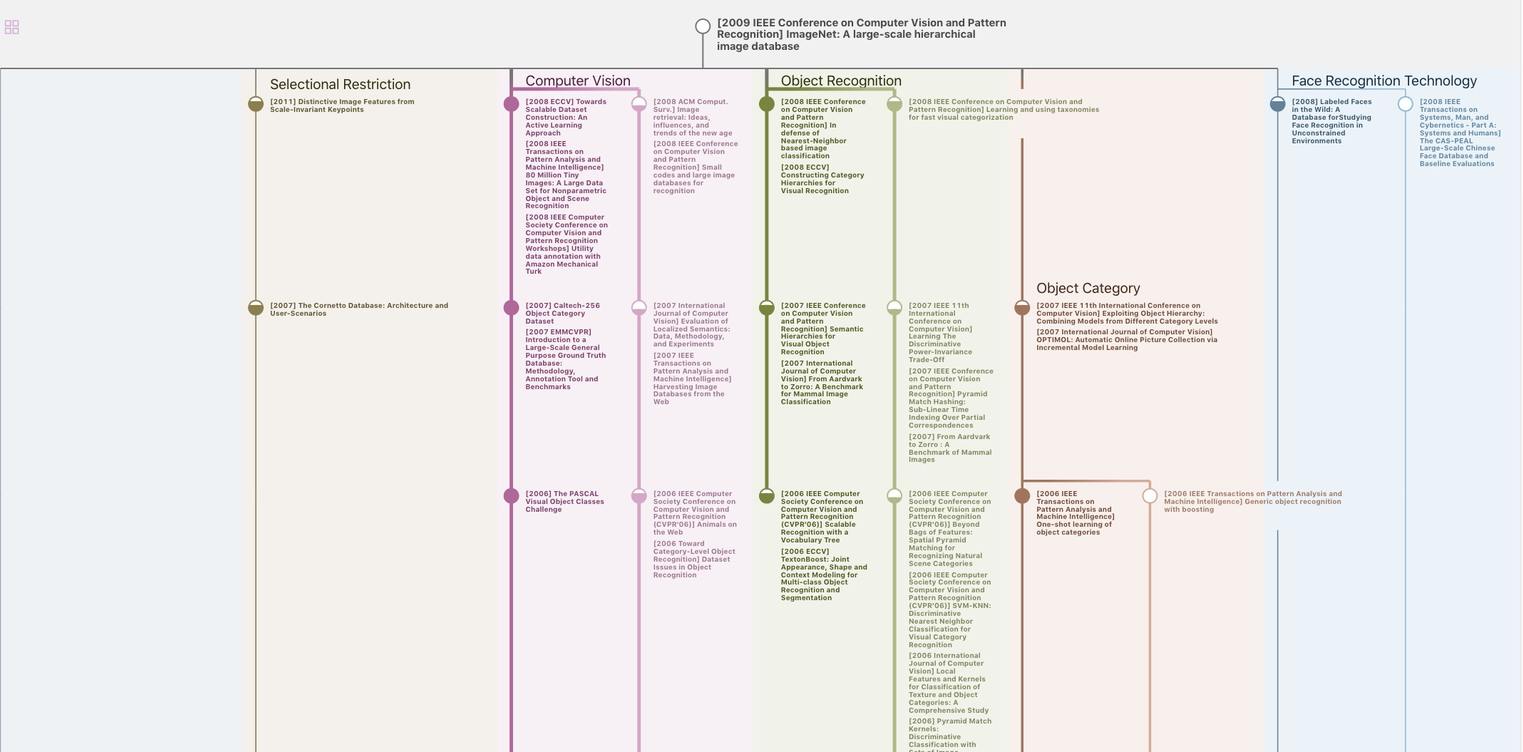
生成溯源树,研究论文发展脉络
Chat Paper
正在生成论文摘要