Neural Inter-Frame Compression For Video Coding
2019 IEEE/CVF INTERNATIONAL CONFERENCE ON COMPUTER VISION (ICCV 2019)(2019)
摘要
While there are many deep learning based approaches for single image compression, the field of end-to-end learned video coding has remained much less explored. Therefore, in this work we present an inter-frame compression approach for neural video coding that can seamlessly build up on different existing neural image codecs. Our end-to-end solution performs temporal prediction by optical flow based motion compensation in pixel space. The key insight is that we can increase both decoding efficiency and reconstruction quality by encoding the required information into a latent representation that directly decodes into motion and blending coefficients. In order to account for remaining prediction errors, residual information between the original image and the interpolated frame is needed. We propose to compute residuals directly in latent space instead of in pixel space as this allows to reuse the same image compression network for both key frames and intermediate frames. Our extended evaluation on different datasets and resolutions shows that the rate-distortion performance of our approach is competitive with existing state-of-the-art codecs.
更多查看译文
关键词
intermediate frames,neural inter-frame compression,deep learning,single image compression,neural video coding,optical flow based motion compensation,reconstruction quality,prediction errors,residual information,interpolated frame,image compression network
AI 理解论文
溯源树
样例
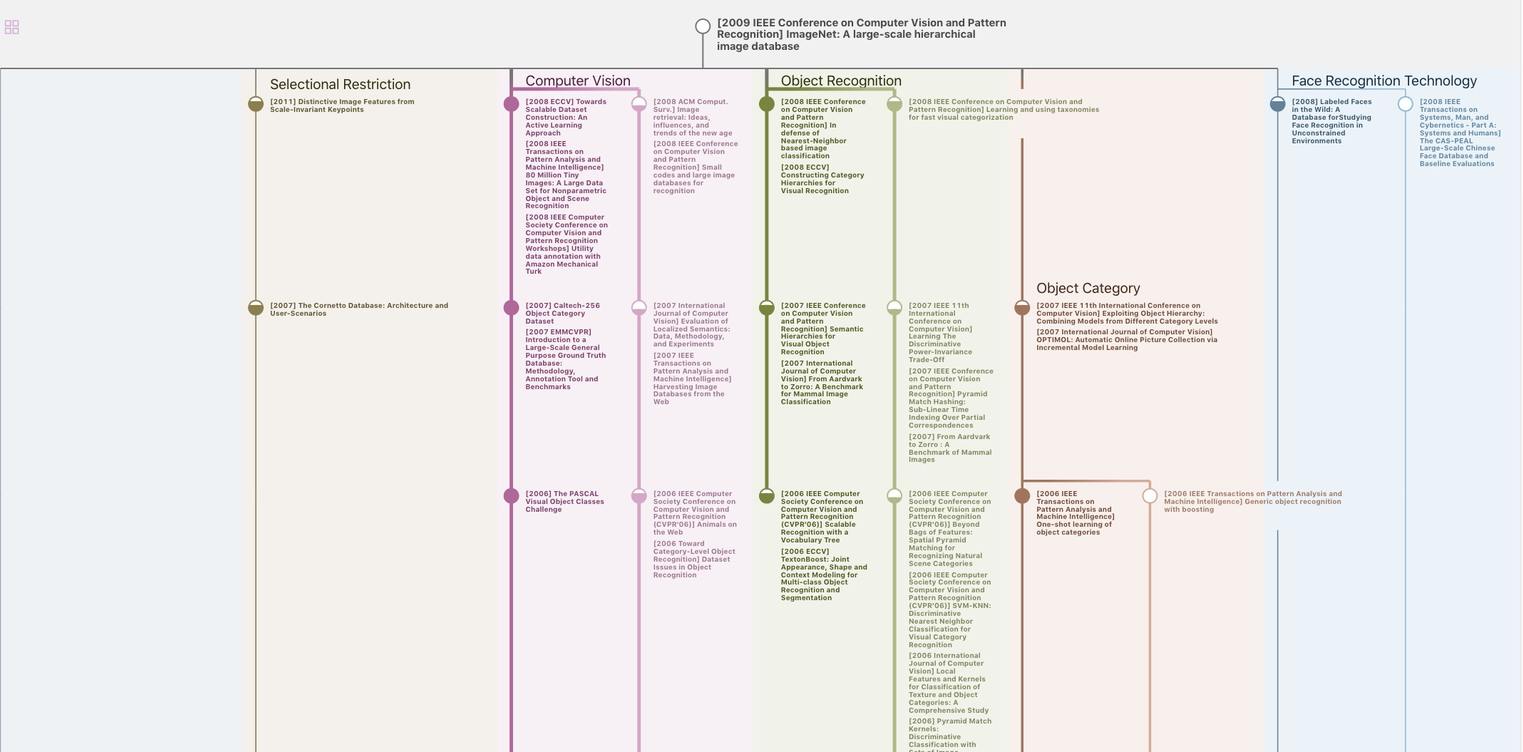
生成溯源树,研究论文发展脉络
Chat Paper
正在生成论文摘要