Correlated Feature Selection with Extended Exclusive Group Lasso
arxiv(2020)
摘要
In many high dimensional classification or regression problems set in a biological context, the complete identification of the set of informative features is often as important as predictive accuracy, since this can provide mechanistic insight and conceptual understanding. Lasso and related algorithms have been widely used since their sparse solutions naturally identify a set of informative features. However, Lasso performs erratically when features are correlated. This limits the use of such algorithms in biological problems, where features such as genes often work together in pathways, leading to sets of highly correlated features. In this paper, we examine the performance of a Lasso derivative, the exclusive group Lasso, in this setting. We propose fast algorithms to solve the exclusive group Lasso, and introduce a solution to the case when the underlying group structure is unknown. The solution combines stability selection with random group allocation and introduction of artificial features. Experiments with both synthetic and real-world data highlight the advantages of this proposed methodology over Lasso in comprehensive selection of informative features.
更多查看译文
关键词
extended exclusive group lasso,selection
AI 理解论文
溯源树
样例
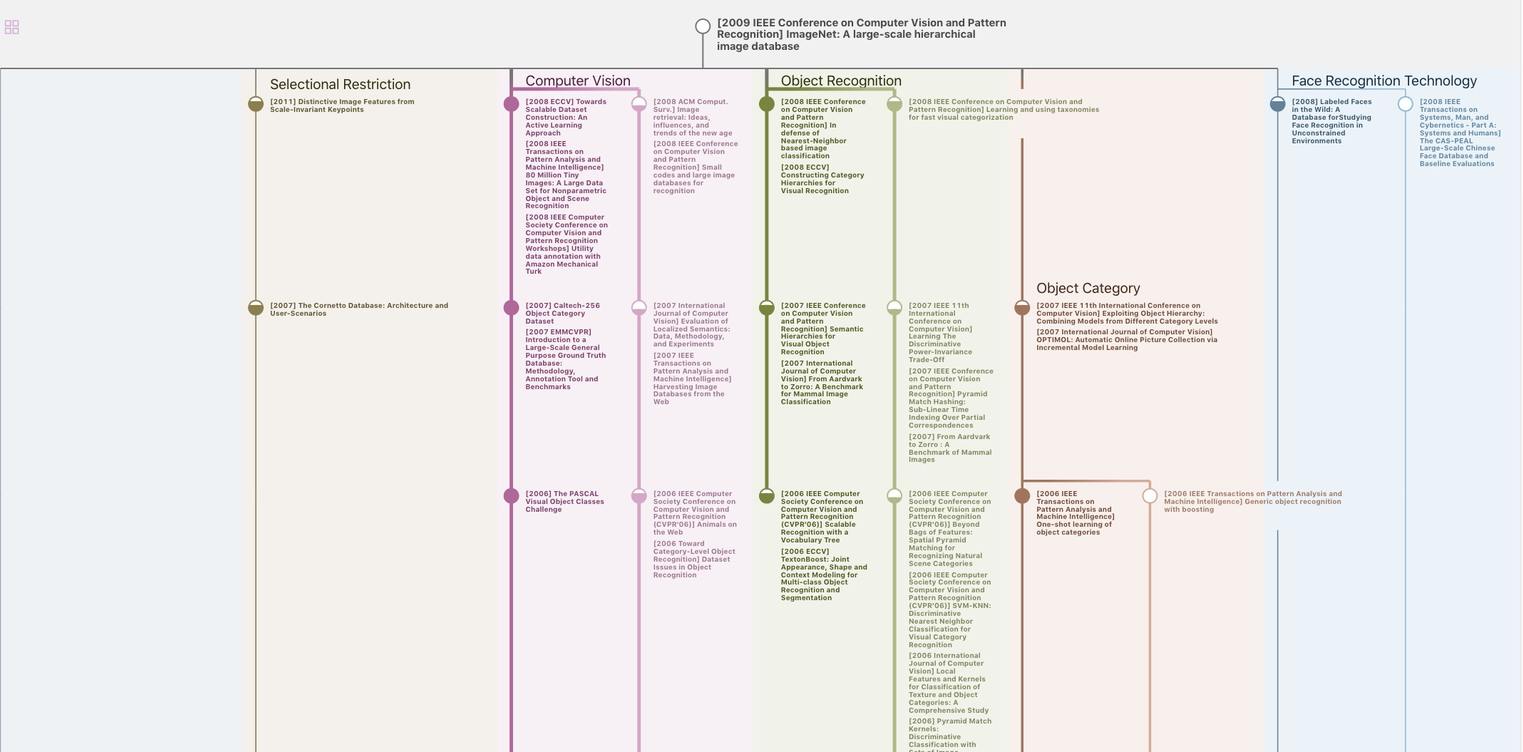
生成溯源树,研究论文发展脉络
Chat Paper
正在生成论文摘要