Feature Significance In Wide Neural Networks
2019 IEEE SYMPOSIUM SERIES ON COMPUTATIONAL INTELLIGENCE (IEEE SSCI 2019)(2019)
摘要
Wide neural networks were recently proposed as a less costly alternative to deep neural networks. In this paper, we analyze the properties of wide neural networks regarding feature selection and their significance. We compared the random selection of weights in the hidden layer to the selection based on radial basis functions. Wide neural networks were also compared with fully connected cascade networks. Feature significance was introduced as a measure to compare various feature selection techniques. Another performance measure introduced in this paper - incremental feature significance - determines the level of improvement that results from selecting only some features, which were added to the existing features, rather than replacing one set of features with another. In both cases, we can also estimate the number of features saved by replacing the original features with the selected ones for which recognition levels improve. This approach can be applied to wide networks that use different feature selection methods than those that are analyzed in this paper; like a k-nearest neighbor, an autoencoder etc.
更多查看译文
关键词
Broad neural networks, feature significance, incremental feature significance
AI 理解论文
溯源树
样例
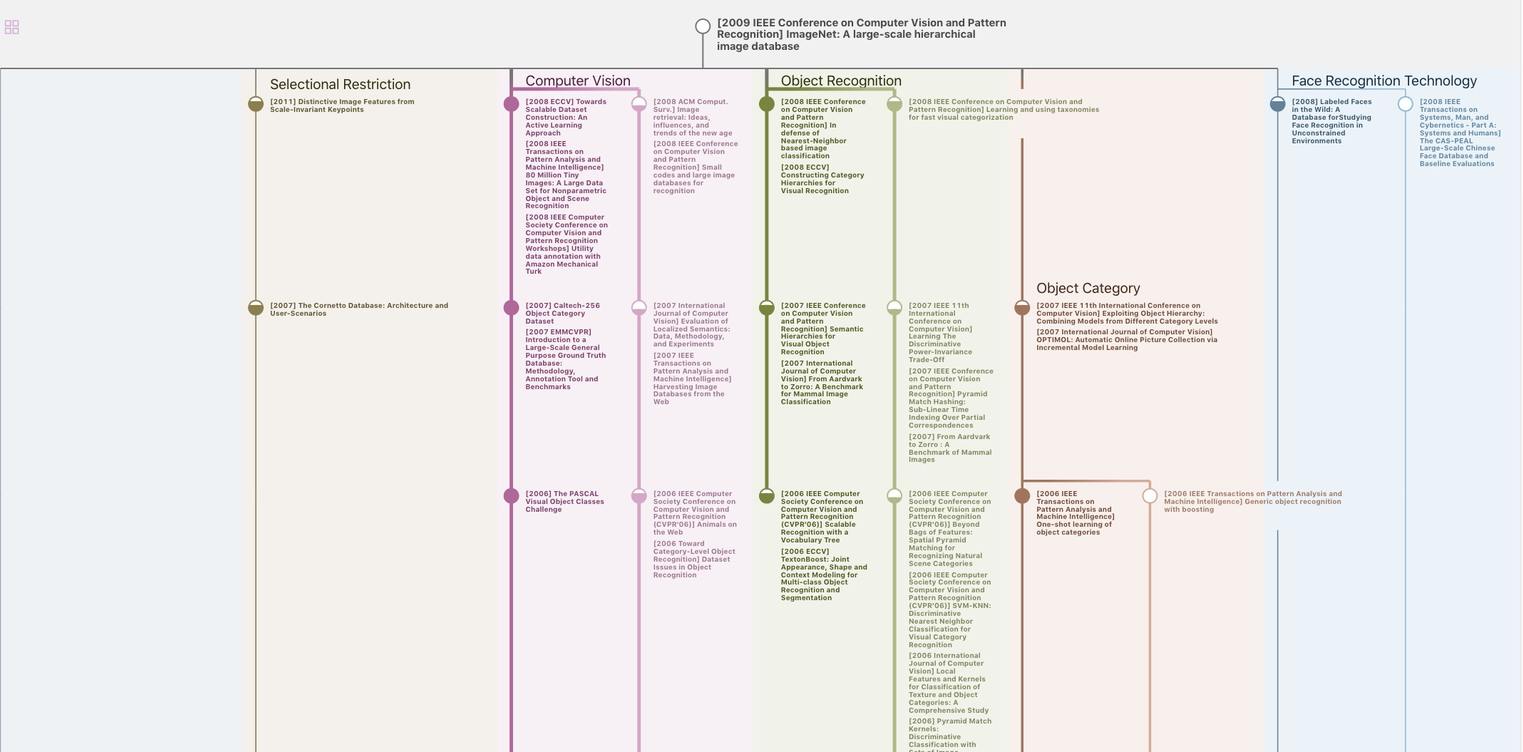
生成溯源树,研究论文发展脉络
Chat Paper
正在生成论文摘要