Variational Inference Of Finite Asymmetric Gaussian Mixture Models
2019 IEEE SYMPOSIUM SERIES ON COMPUTATIONAL INTELLIGENCE (IEEE SSCI 2019)(2019)
摘要
Mixture models are a popular unsupervised learning technique useful for discovering homogeneous clusters in unlabeled data. A key research problem lies in the accurate and efficient determination of their associated parameters. Variational inference has recently risen as a prominent parameter learning approach. Hence, in this research, we propose a variational Bayes learning framework for asymmetric Gaussian mixture model. Unlike Gaussian mixture models, these models incorporate the asymmetric shape of data and are adaptive to different conditions in real-word image processing domains. Experimental results show the merit of the proposed approach.
更多查看译文
关键词
Mixture Model, Variational Bayes Inference, Asymmetric Gaussian Distribution, Background Subtraction
AI 理解论文
溯源树
样例
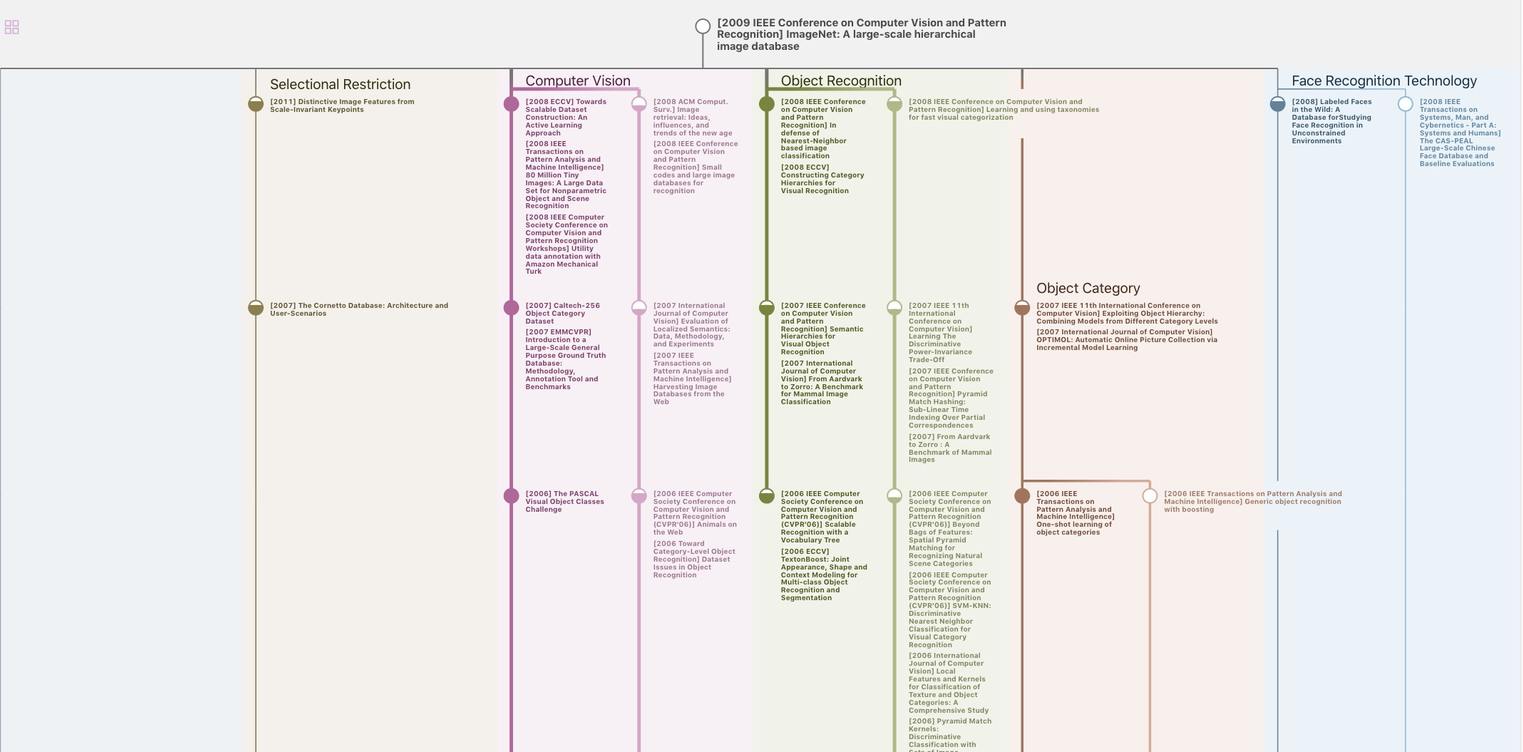
生成溯源树,研究论文发展脉络
Chat Paper
正在生成论文摘要