A Hidden Feature Selection Method Based On L(2,0)-Norm Regularization For Training Single-Hidden-Layer Neural Networks
2019 IEEE SYMPOSIUM SERIES ON COMPUTATIONAL INTELLIGENCE (IEEE SSCI 2019)(2019)
摘要
Feature selection is an important data preprocessing for machine learning. It can improve the performance of machine learning algorithms by removing redundant and noisy features. Among all the methods, those based on l(1)-norms or l(2,1)-norms have received considerable attention due to their good performance. However, these methods cannot produce exact row sparsity to the weight matrix, so the number of selected features cannot be determined automatically without using a threshold. To this end, this paper proposes a feature selection method incorporating the l(2,0)-norm, which can guarantee exact row sparsity of weight matrix. A method based on iterative hard thresholding (IHT) algorithm is also proposed to solve the l(2,0)-norm regularized least square problem. For fully using the role of row-sparsity induced by the l(2,0)-norm, this method acts as network pruning for single-hidden-layer neural networks. This method is conducted on the hidden features and it can achieve node-level pruning rather than the connection-level pruning. The experimental results in several public data sets and three image recognition data sets have shown that this method can not only effectively prune the useless hidden nodes, but also obtain better performance.
更多查看译文
关键词
Feature selection, l(2,0)-norm, Iterative hard thresholding (IHT) algorithm
AI 理解论文
溯源树
样例
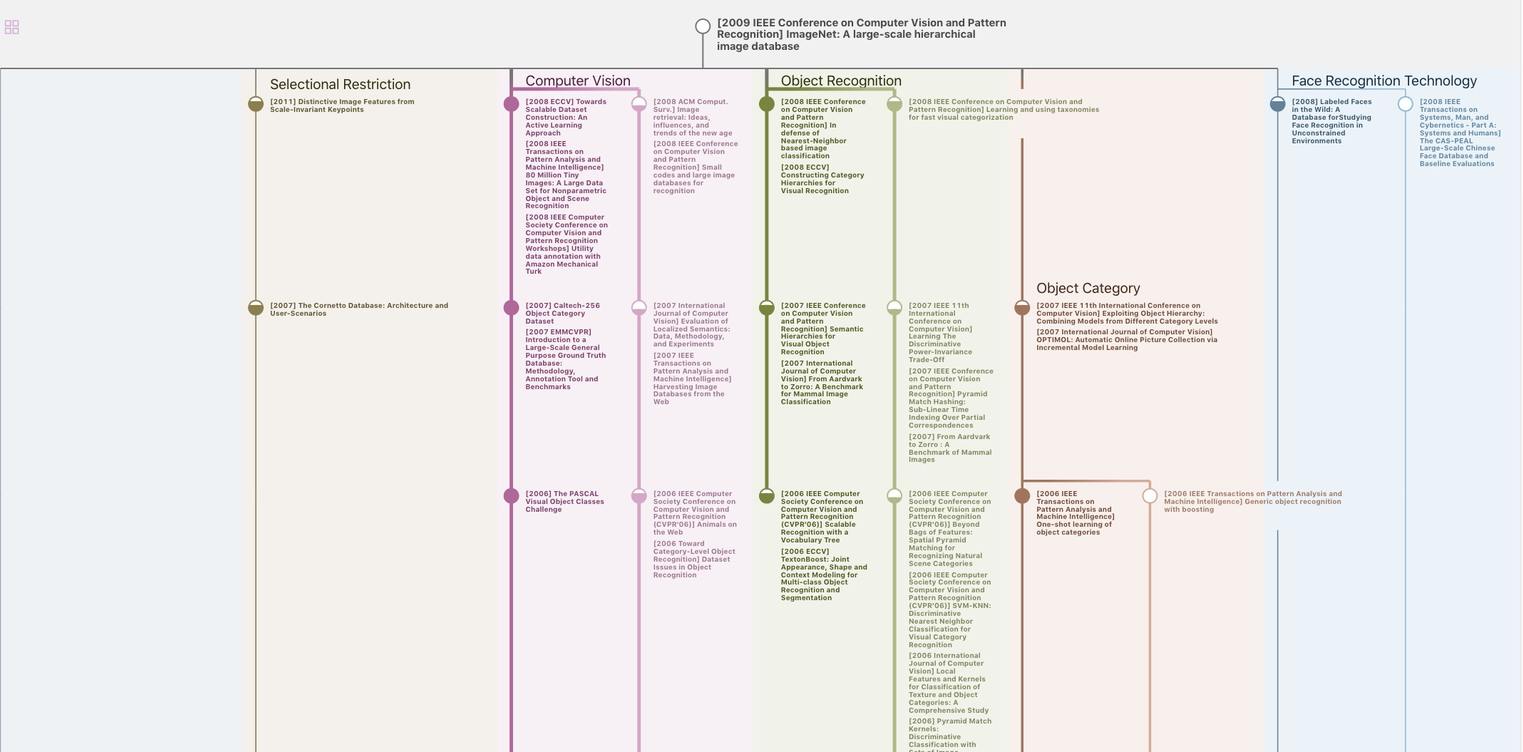
生成溯源树,研究论文发展脉络
Chat Paper
正在生成论文摘要