A Multi-View Unified Feature Learning Network For Eeg Epileptic Seizure Detection
2019 IEEE SYMPOSIUM SERIES ON COMPUTATIONAL INTELLIGENCE (IEEE SSCI 2019)(2019)
摘要
Electroencephalogram (EEG) signal is a valuable ancillary test to aide for the diagnosis of neurological disorders, while multi-channel EEG signal is commonly used for seizure epilepsy detection recently. However, accumulation of multivariate EEG signals is not sufficient to unleash the power of multi-view information. Therefore, in this paper we propose a novel multi-view learning framework with a unified multidomain feature learning method for seizure epilepsy detection. We first apply a patient-specific channel selection mechanism to pick up seizure-relevant EEG channels and construct a multiview framework by adopting discrete wavelet transform (DWT) method to generalize selected five-channel signals into five sets of sub-band frequency components corresponding to five views, which enhances intra-view coherence and cross-view complementary efficiently. Moreover, a unified feature learning method within each view is proposed with common spatial patterns (CSP) for statistical and morphological features and a semi-supervised maximum mean discrepancy autoencoder (semi-MMDAE) for cross-channel time-frequency domain representations extraction. Instead of extracting sub-feature sets separately, unified feature learning procedure prevents overfitting problem and reduces redundant features efficiently. Experimental results compared with state-of-the-art studies on Children's Hospital Boston-Massachusetts Institute of Technology (CHB-MIT) Scalp EEG Database validate superiority of the proposed approach, demonstrating the effectiveness of the pro-posed method in the detection of epileptic seizures.
更多查看译文
关键词
electroencephalogram, multi-view learning, deep learning, seizure detection
AI 理解论文
溯源树
样例
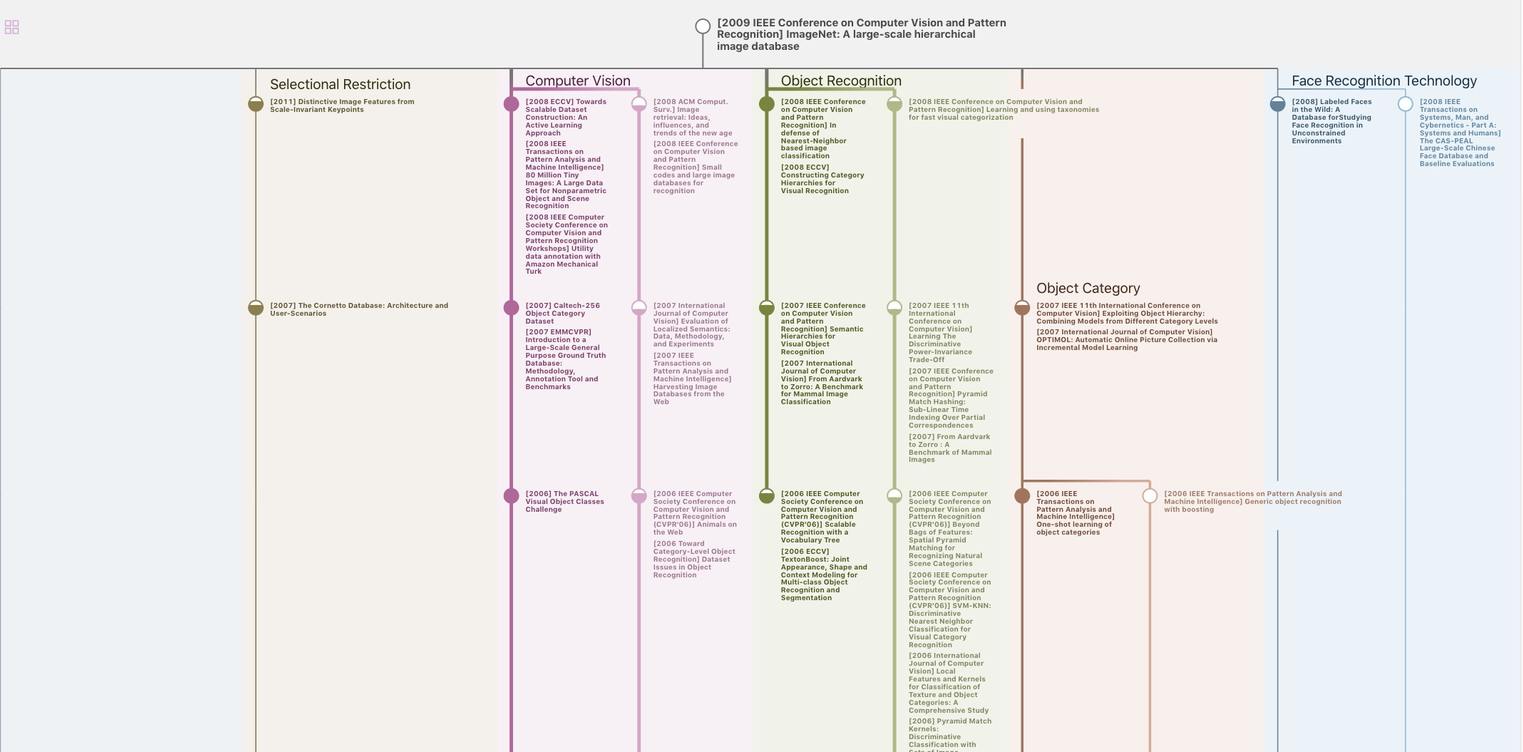
生成溯源树,研究论文发展脉络
Chat Paper
正在生成论文摘要