Self-Supervised Deep Pose Corrections for Robust Visual Odometry
ICRA(2020)
摘要
We present a self-supervised deep pose correction (DPC) network that applies pose corrections to a visual odometry estimator to improve its accuracy. Instead of regressing inter-frame pose changes directly, we build on prior work that uses data-driven learning to regress pose corrections that account for systematic errors due to violations of modelling assumptions. Our self-supervised formulation removes any requirement for six-degrees-of-freedom ground truth and, in contrast to expectations, often improves overall navigation accuracy compared to a supervised approach. Through extensive experiments, we show that our self-supervised DPC network can significantly enhance the performance of classical monocular and stereo odometry estimators and substantially out-performs state-of-the-art learning-only approaches.
更多查看译文
关键词
self-supervised deep pose corrections,robust visual odometry,data-driven learning,six-degrees-of-freedom ground truth,self-supervised DPC network,stereo odometry estimators,pose corrections regression,monocular odometry estimators
AI 理解论文
溯源树
样例
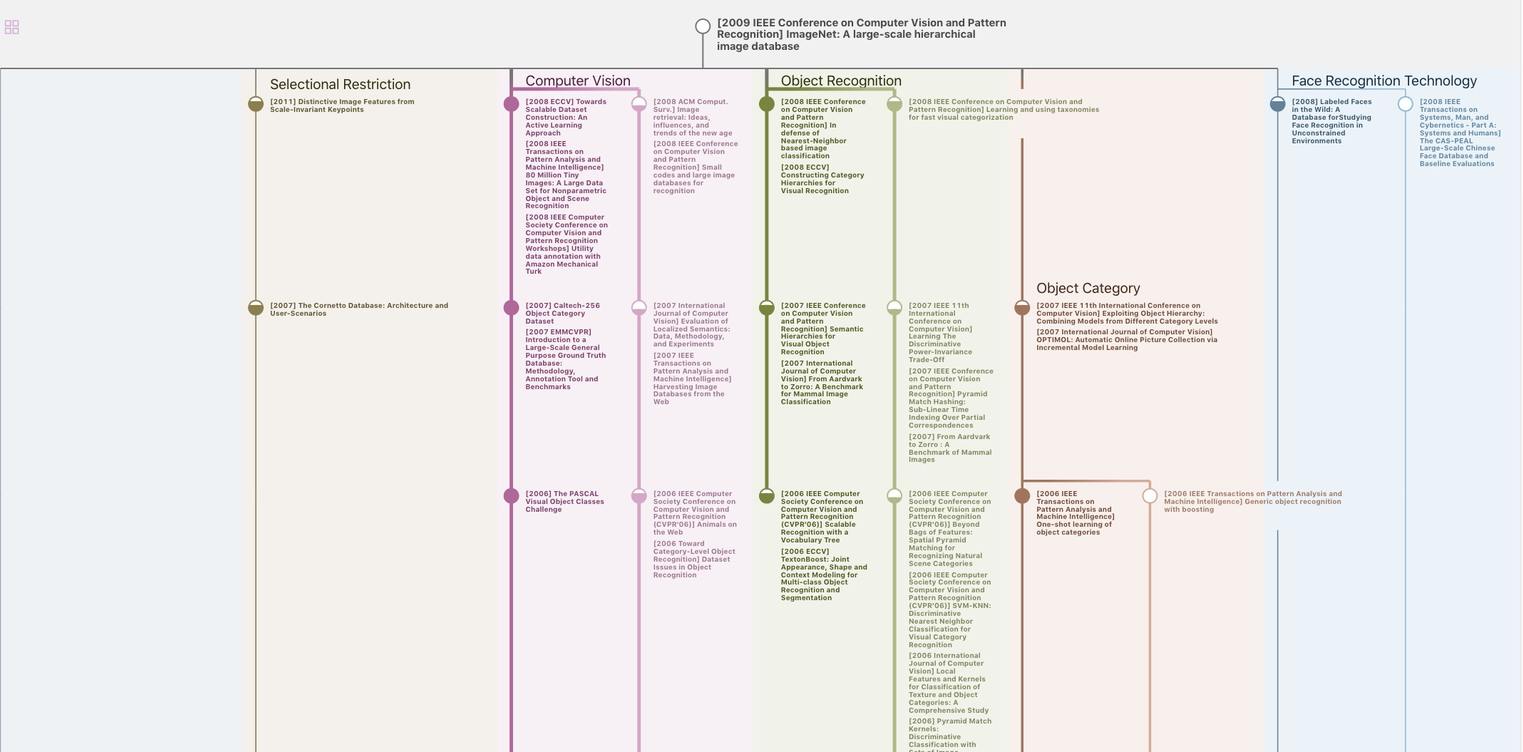
生成溯源树,研究论文发展脉络
Chat Paper
正在生成论文摘要