Multi-Cycle-Consistent Adversarial Networks for CT Image Denoising
2020 IEEE 17TH INTERNATIONAL SYMPOSIUM ON BIOMEDICAL IMAGING (ISBI 2020)(2020)
摘要
CT image denoising can be treated as an image-to-image translation task where the goal is to learn the transform between a source domain $X$ (noisy images) and a target domain $Y$ (clean images). Recently, cycle-consistent adversarial denoising network (CCADN) has achieved state-of-the-art results by enforcing cycle-consistent loss without the need of paired training data. Our detailed analysis of CCADN raises a number of interesting questions. For example, if the noise is large leading to significant difference between domain $X$ and domain $Y$, can we bridge $X$ and $Y$ with an intermediate domain $Z$ such that both the denoising process between $X$ and $Z$ and that between $Z$ and $Y$ are easier to learn? As such intermediate domains lead to multiple cycles, how do we best enforce cycle-consistency? Driven by these questions, we propose a multi-cycle-consistent adversarial network (MCCAN) that builds intermediate domains and enforces both local and global cycle-consistency. The global cycle-consistency couples all generators together to model the whole denoising process, while the local cycle-consistency imposes effective supervision on the process between adjacent domains. Experiments show that both local and global cycle-consistency are important for the success of MCCAN, which outperforms the state-of-the-art.
更多查看译文
关键词
Machine learning, Image enhancement/restoration (noise and artifact reduction), Computed tomography (CT), Multi-cycle-consistency
AI 理解论文
溯源树
样例
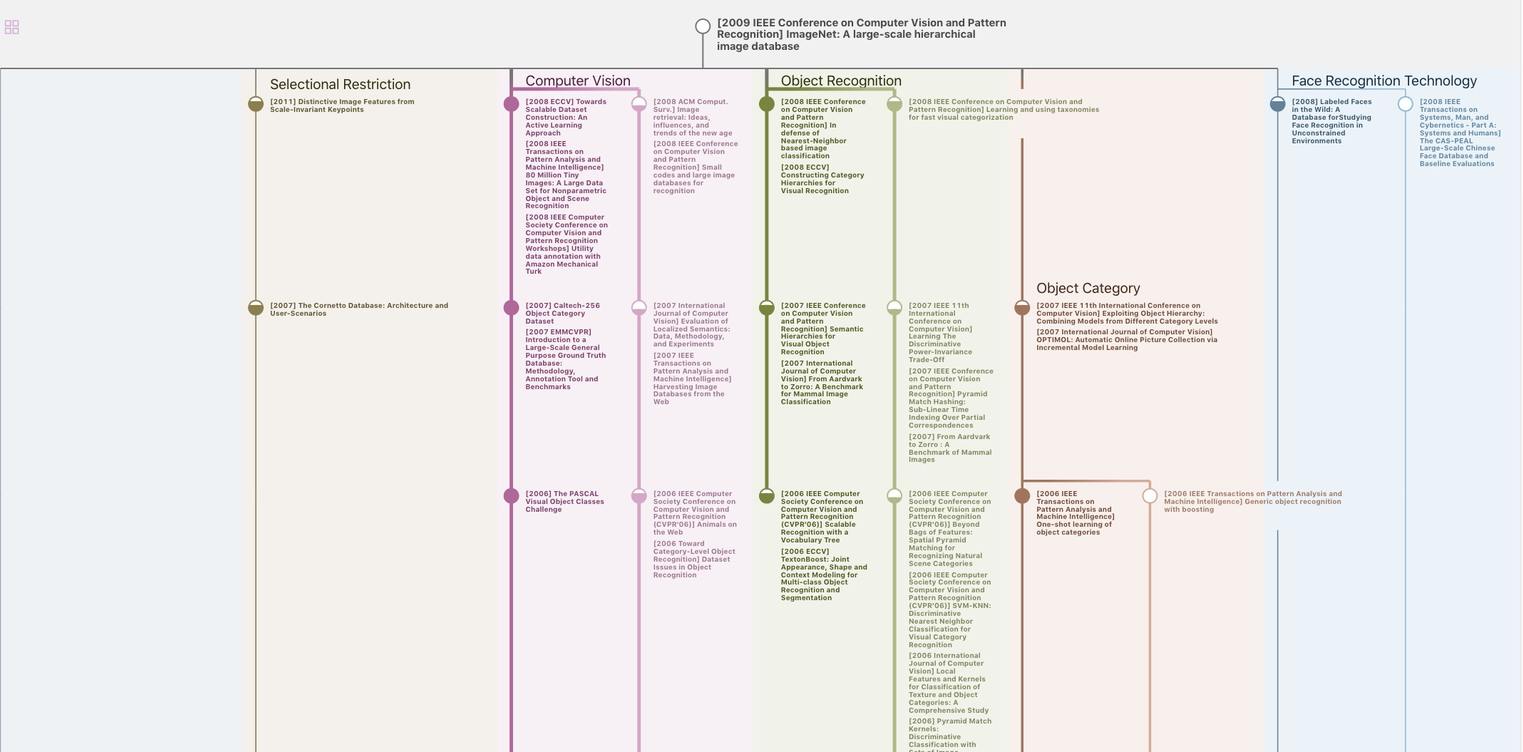
生成溯源树,研究论文发展脉络
Chat Paper
正在生成论文摘要