SWARM: Adaptive Load Balancing in Distributed Streaming Systems for Big Spatial Data
ACM Transactions on Spatial Algorithms and Systems(2021)
摘要
AbstractThe proliferation of GPS-enabled devices has led to the development of numerous location-based services. These services need to process massive amounts of streamed spatial data in real-time. The current scale of spatial data cannot be handled using centralized systems. This has led to the development of distributed spatial streaming systems. Existing systems are using static spatial partitioning to distribute the workload. In contrast, the real-time streamed spatial data follows non-uniform spatial distributions that are continuously changing over time. Distributed spatial streaming systems need to react to the changes in the distribution of spatial data and queries. This article introduces SWARM, a lightweight adaptivity protocol that continuously monitors the data and query workloads across the distributed processes of the spatial data streaming system and redistributes and rebalances the workloads as soon as performance bottlenecks get detected. SWARM is able to handle multiple query-execution and data-persistence models. A distributed streaming system can directly use SWARM to adaptively rebalance the system’s workload among its machines with minimal changes to the original code of the underlying spatial application. Extensive experimental evaluation using real and synthetic datasets illustrate that, on average, SWARM achieves 2\(\) improvement in throughput over a static grid partitioning that is determined based on observing a limited history of the data and query workloads. Moreover, SWARM reduces execution latency on average 4\(\) compared with the other technique.
更多查看译文
关键词
Load balancing, distributed streaming systems, spatial stream processing, cluster utilization, spatial continuous queries
AI 理解论文
溯源树
样例
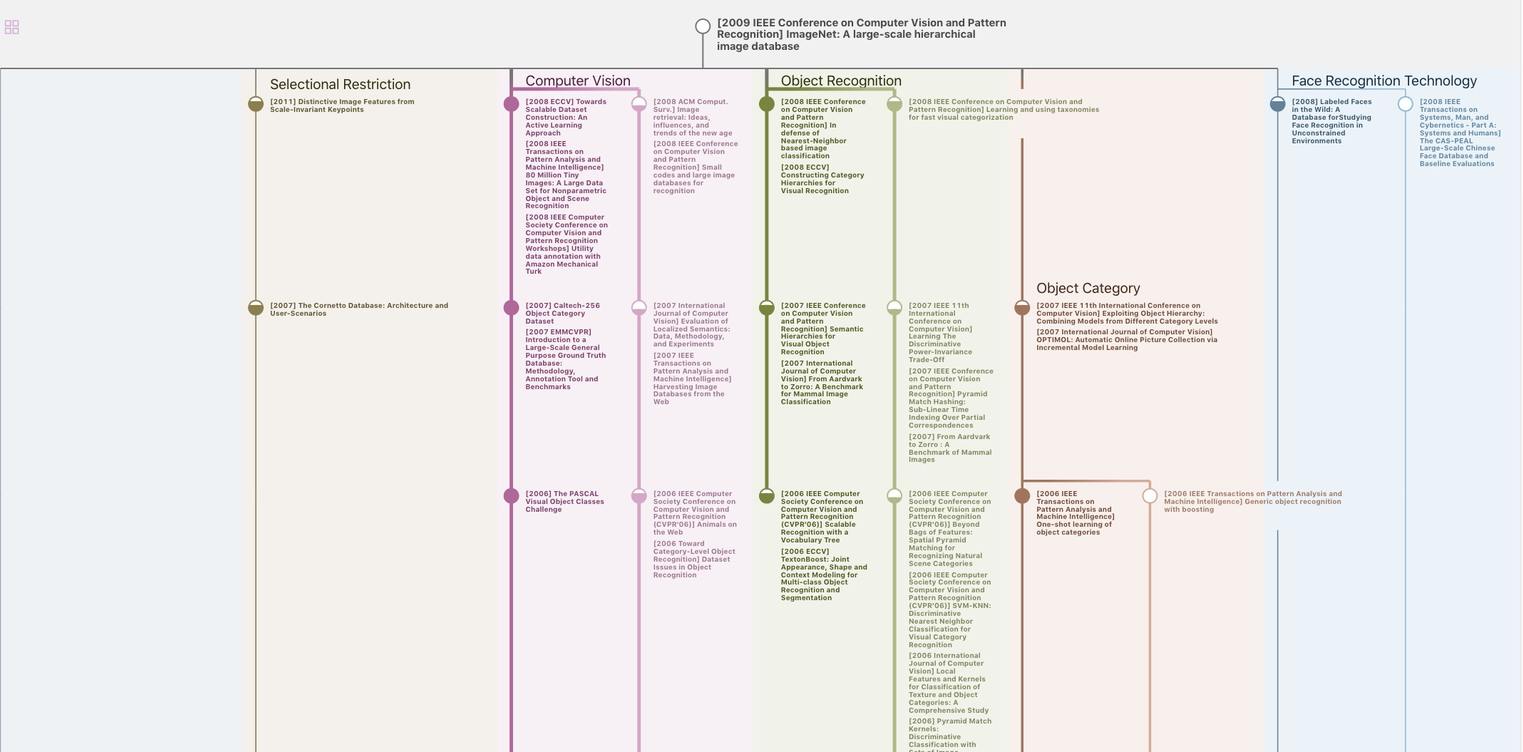
生成溯源树,研究论文发展脉络
Chat Paper
正在生成论文摘要