ARMA Nets: Expanding Receptive Field for Dense Prediction
NIPS 2020(2020)
摘要
Global information is essential for dense prediction problems, whose goal is to compute a discrete or continuous label for each pixel in the images. Traditional convolutional layers in neural networks, originally designed for image classification, are restrictive in these problems since their receptive fields are limited by the filter size. In this work, we propose autoregressive moving-average (ARMA) layer, a novel module in neural networks to allow explicit dependencies of output neurons, which significantly expands the receptive field with minimal extra parameters. We show experimentally that the effective receptive field of neural networks with ARMA layers expands as autoregressive coefficients become larger. In addition, we demonstrate that neural networks with ARMA layers substantially improve the performance of challenging pixel-level video prediction tasks as our model enlarges the effective receptive field.
更多查看译文
关键词
dense prediction,receptive field,nets,expanding
AI 理解论文
溯源树
样例
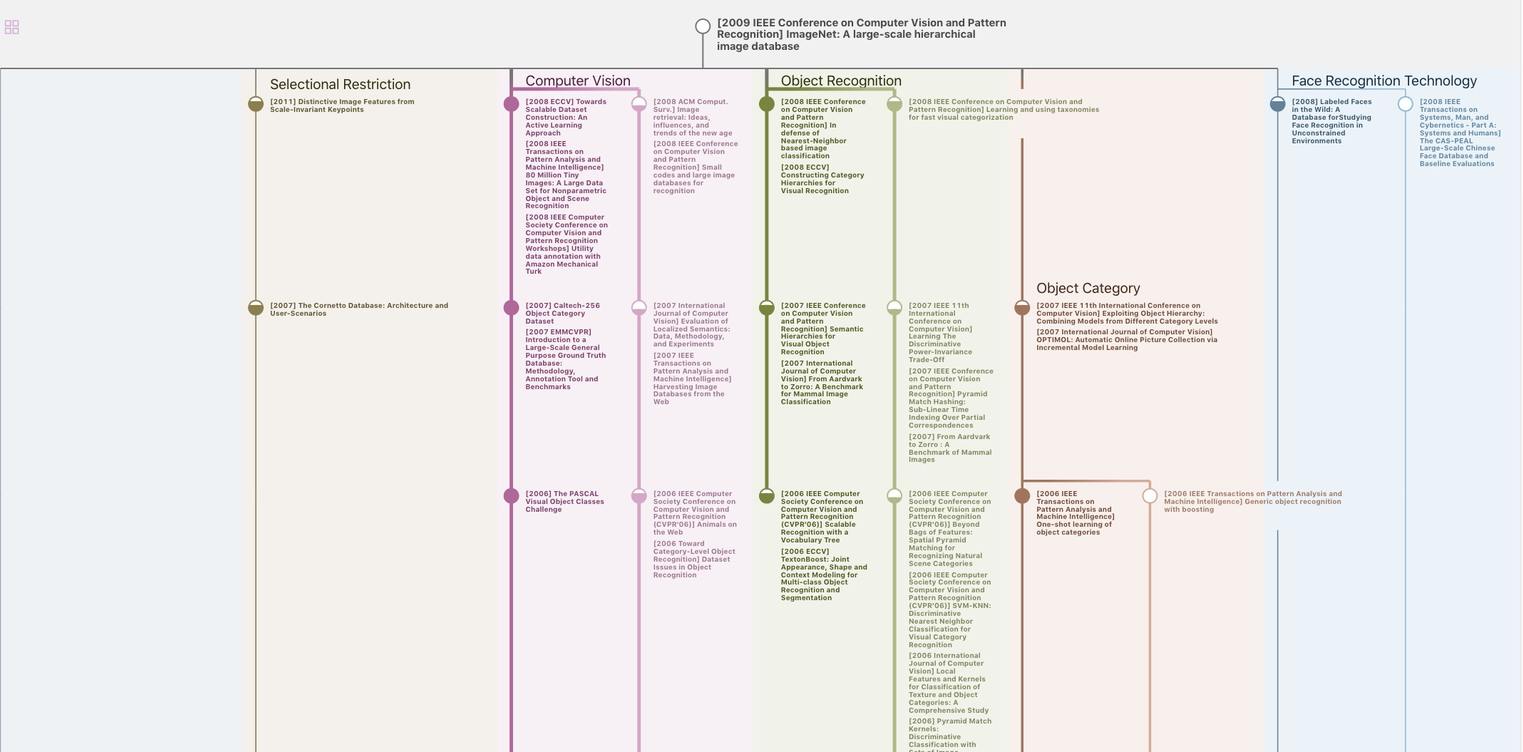
生成溯源树,研究论文发展脉络
Chat Paper
正在生成论文摘要