Supervised Categorical Metric Learning With Schatten p-Norms
IEEE TRANSACTIONS ON CYBERNETICS(2022)
摘要
Metric learning has been successful in learning new metrics adapted to numerical datasets. However, its development of categorical data still needs further exploration. In this article, we propose a method, called CPML for categorical projected metric learning, which tries to efficiently (i.e., less computational time and better prediction accuracy) address the problem of metric learning in categorical data. We make use of the value distance metric to represent our data and propose new distances based on this representation. We then show how to efficiently learn new metrics. We also generalize several previous regularizers through the Schatten p-norm and provide a generalization bound for it that complements the standard generalization bound for metric learning. The experimental results show that our method provides state-of-the-art results while being faster.
更多查看译文
关键词
Categorical data,metric learning
AI 理解论文
溯源树
样例
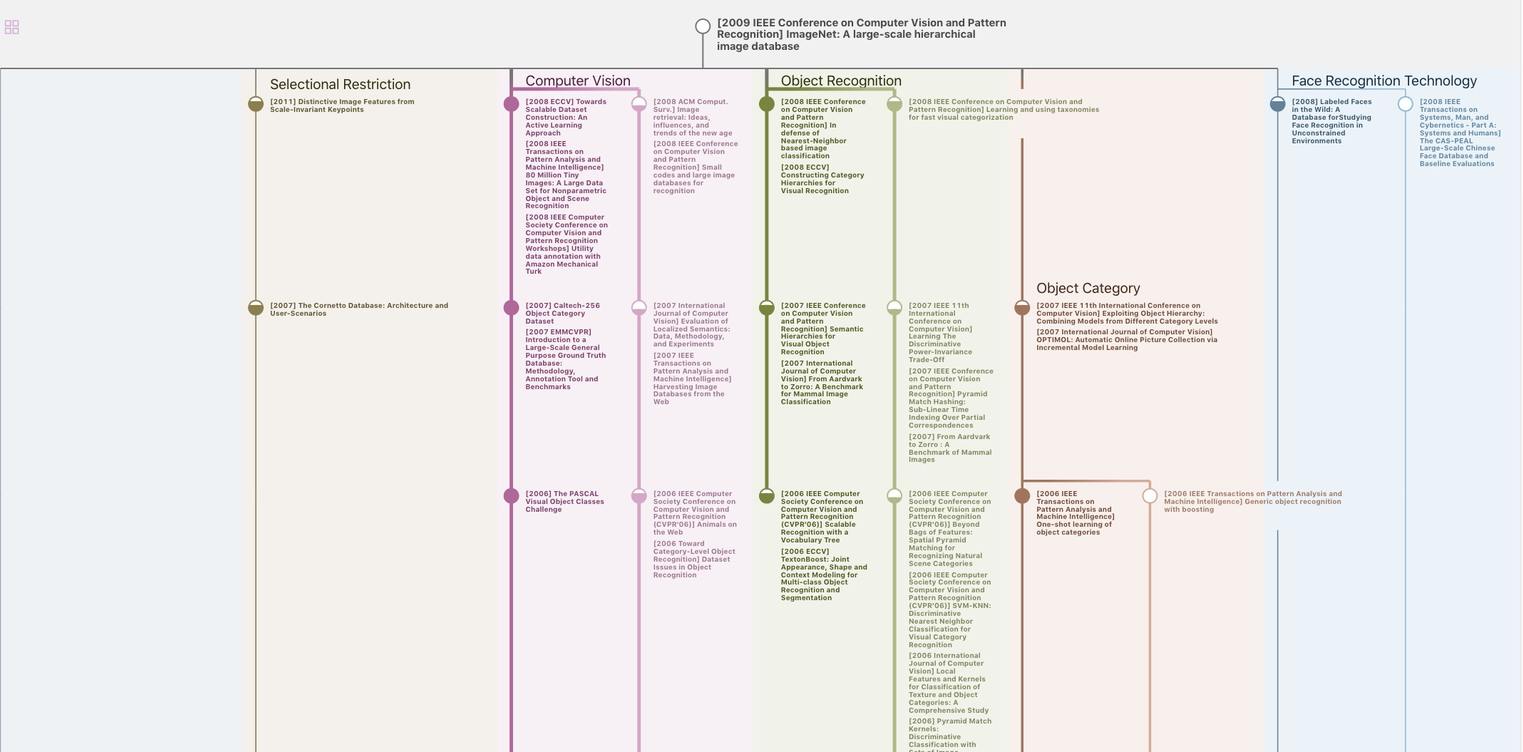
生成溯源树,研究论文发展脉络
Chat Paper
正在生成论文摘要