sBSNN: Stochastic-Bits Enabled Binary Spiking Neural Network with On-Chip Learning for Energy Efficient Neuromorphic Computing at the Edge
IEEE Transactions on Circuits and Systems I: Regular Papers(2020)
摘要
In this work, we propose stochastic Binary Spiking Neural Network (sBSNN) composed of stochastic spiking neurons and binary synapses (stochastic only during training) that computes probabilistically with one-bit precision for power-efficient and memory-compressed neuromorphic computing. We present an energy-efficient implementation of the proposed sBSNN using
‘stochastic bit’
as the core computational primitive to realize the stochastic neurons and synapses, which are fabricated in 90nm CMOS process, to achieve efficient on-chip training and inference for image recognition tasks. The measured data shows that the
‘stochastic bit’
can be programmed to mimic spiking neurons, and stochastic Spike Timing Dependent Plasticity (or sSTDP) rule for training the binary synaptic weights without expensive random number generators. Our results indicate that the proposed sBSNN realization offers possibility of up to
$32\times $
neuronal and synaptic memory compression compared to full precision (32-bit) SNN and energy efficiency of 89.49 TOPS/Watt for two-layer fully-connected SNN.
更多查看译文
关键词
Neurons,Synapses,Stochastic processes,Training,System-on-chip,Probabilistic logic,Timing
AI 理解论文
溯源树
样例
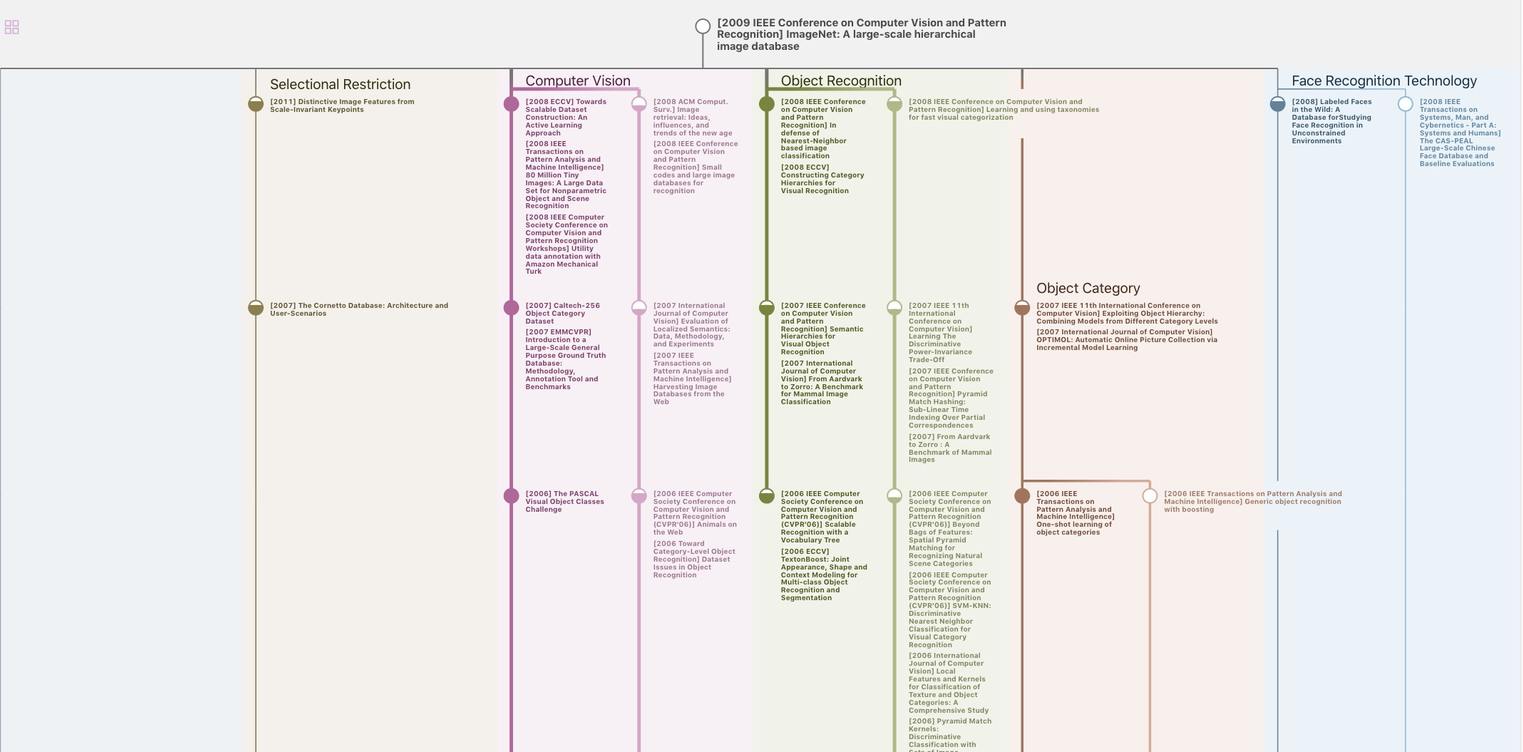
生成溯源树,研究论文发展脉络
Chat Paper
正在生成论文摘要