Agent-Based Model Characterization Using Natural Language Processing
2019 Winter Simulation Conference (WSC)(2019)
摘要
This paper reports on Natural Language Processing (NLP) as a technique to analyze phenomena towards specifying agent-based models (ABM). The objective of the ABM NLP Analyzer is to facilitate non-simulationists to actively engage in the learning and collaborative designing of ABMs. The NLP model identifies candidate agents, candidate agent attributes, and candidate rules all of which non-simulationists can later evaluate for feasibility. IBM's Watson Natural Language Understanding (NLU) and Knowledge Studio were used in order to annotate, evaluate, extract agents, agent attributes, and agent rules from unstructured descriptions of phenomena. The software, and related agent-attribute-rule characterization, provides insight into a simple but useful means of conceptualizing and specifying baseline ABMs. Further, it emphasizes on how to approach the design of ABMs without the use of NLP by focusing on the identification of agent, attributes and rules.
更多查看译文
关键词
agent-based model characterization,natural language processing,agent-based models,ABM NLP Analyzer,NLP model,IBM Watson natural language understanding,agent-attribute-rule characterization
AI 理解论文
溯源树
样例
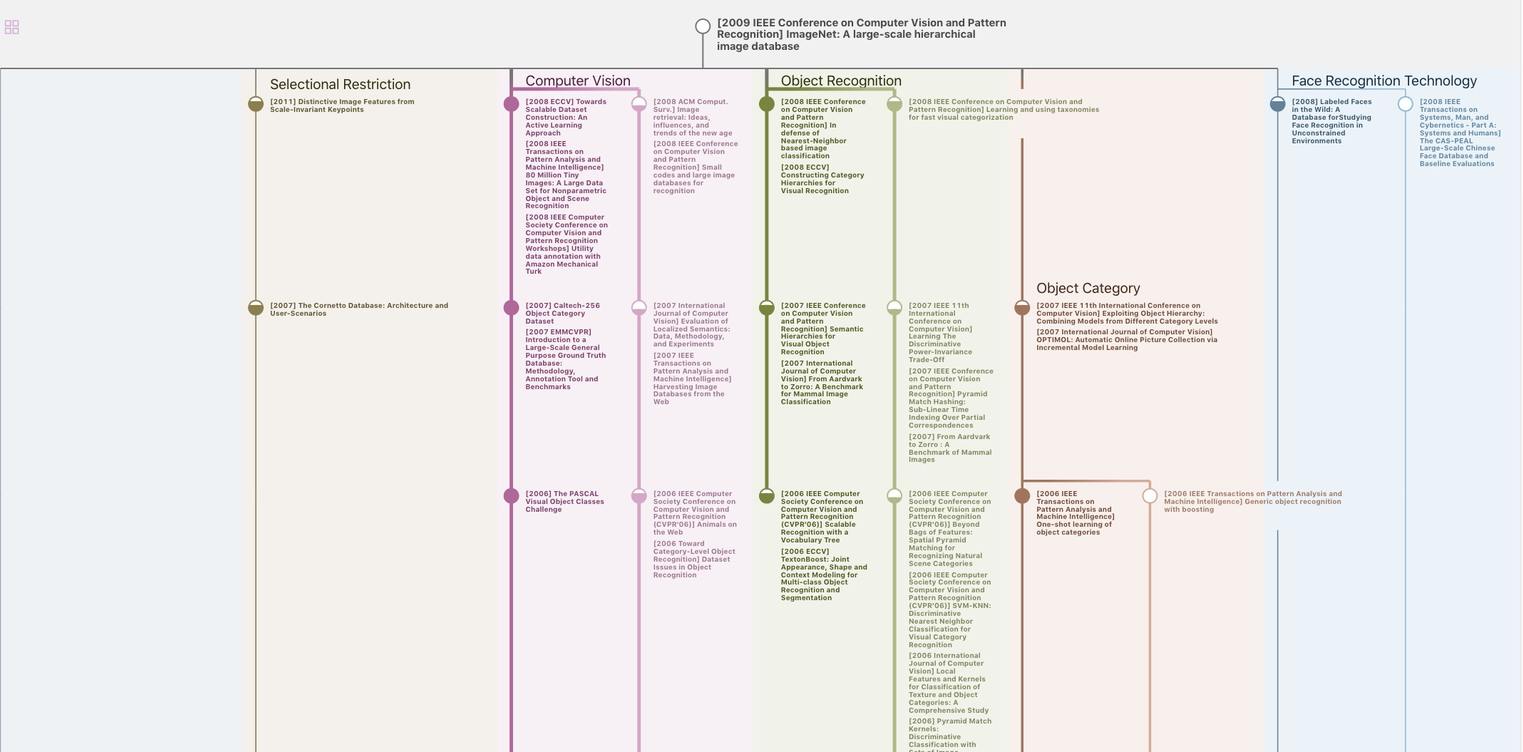
生成溯源树,研究论文发展脉络
Chat Paper
正在生成论文摘要