Improving Speech-Based End-Of-Turn Detection Via Cross-Modal Representation Learning With Punctuated Text Data
2019 IEEE AUTOMATIC SPEECH RECOGNITION AND UNDERSTANDING WORKSHOP (ASRU 2019)(2019)
摘要
This paper presents a novel training method for speech-based end-of-turn detection for which not only manually annotated speech data sets but also punctuated text data sets are utilized. The speech-based end-of-turn detection estimates whether a target speaker's utterance is ended or not using speech information. In previous studies, the speech-based end-of-turn detection models were trained using only speech data sets that contained manually annotated end-of-turn labels. However, since the amounts of annotated speech data sets are often limited, the end-of-turn detection models were unable to correctly handle a wide variety of speech patterns. In order to mitigate the data scarcity problem, our key idea is to leverage punctuated text data sets for building more effective speech-based end-of-turn detection. Therefore, the proposed method introduces cross-modal representation learning to construct a speech encoder and a text encoder that can map speech and text with the same lexical information into similar vector representations. This enables us to train speech-based end-ofturn detection models from the punctuated text data sets by tackling text-based sentence boundary detection. In experiments on contact center calls, we show that speech-based end-of-turn detection models using hierarchical recurrent neural networks can be improved through the use of punctuated text data sets.
更多查看译文
关键词
Speech-based End-of-turn detection, punctuated text data, cross-modal representation learning, hierarchical recurrent neural networks
AI 理解论文
溯源树
样例
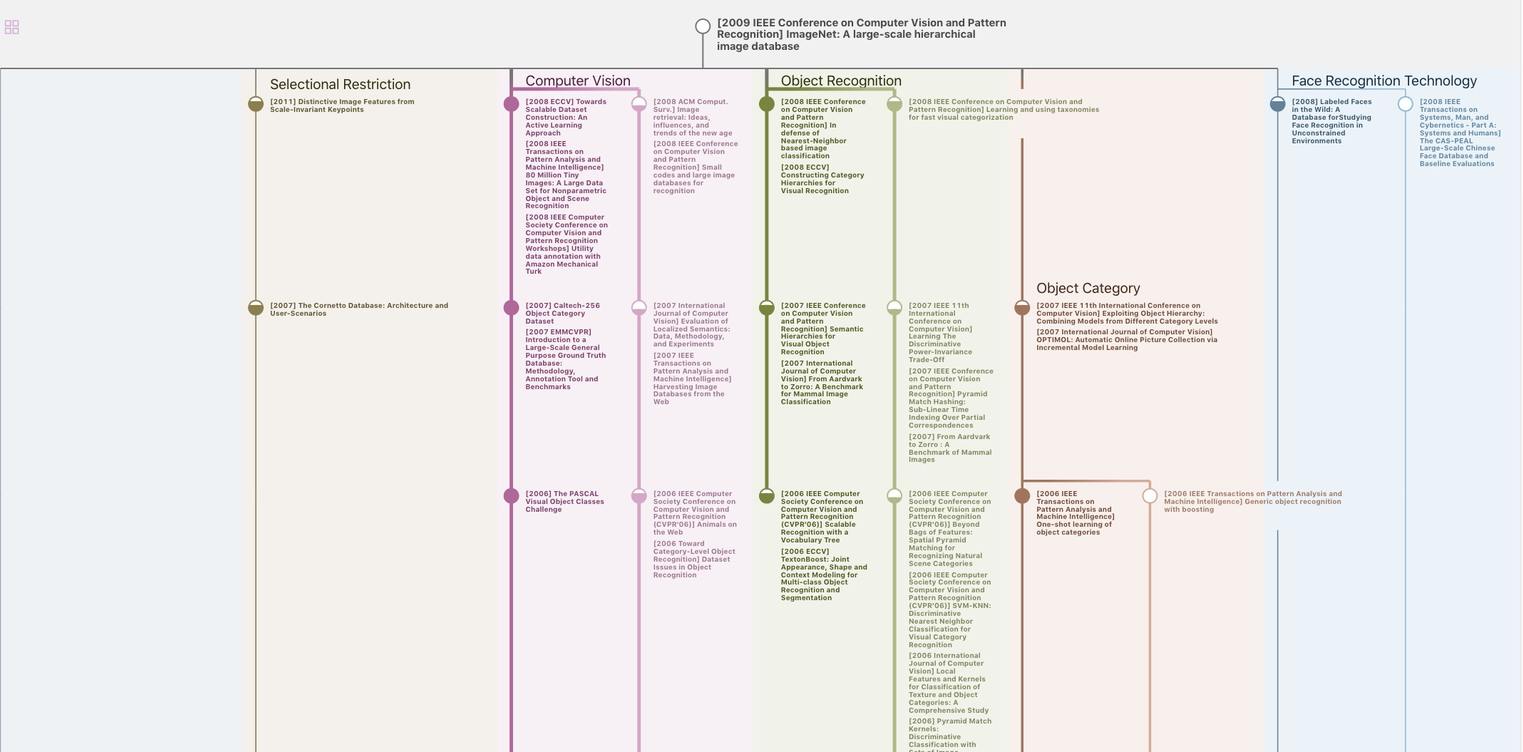
生成溯源树,研究论文发展脉络
Chat Paper
正在生成论文摘要