Sparse Super-Regular Networks.
ICMLA(2019)
摘要
It has been argued by Thom and Palm that sparsely-connected neural networks (SCNs) show improved performance over fully-connected networks (FCNs). Super-regular networks (SRNs) are neural networks composed of a set of stacked sparse layers of (epsilon, delta)-super-regular pairs, and randomly permuted node order. Using the Blow-up Lemma, we prove that as a result of the individual super-regularity of each pair of layers, SRNs guarantee a number of properties that make them suitable replacements for FCNs for many tasks. These guarantees include edge uniformity across all large-enough subsets, minimum node in- and out-degree, input-output sensitivity, and the ability to embed pre-trained constructs. Indeed, SRNs have the capacity to act like FCNs, and eliminate the need for costly regularization schemes like Dropout. We show that SRNs perform similarly to X-Nets via readily reproducible experiments, and offer far greater guarantees and control over network structure.
更多查看译文
关键词
sparse-neural networks,graph theory,super regularity,expander graphs,x-nets,deep learning
AI 理解论文
溯源树
样例
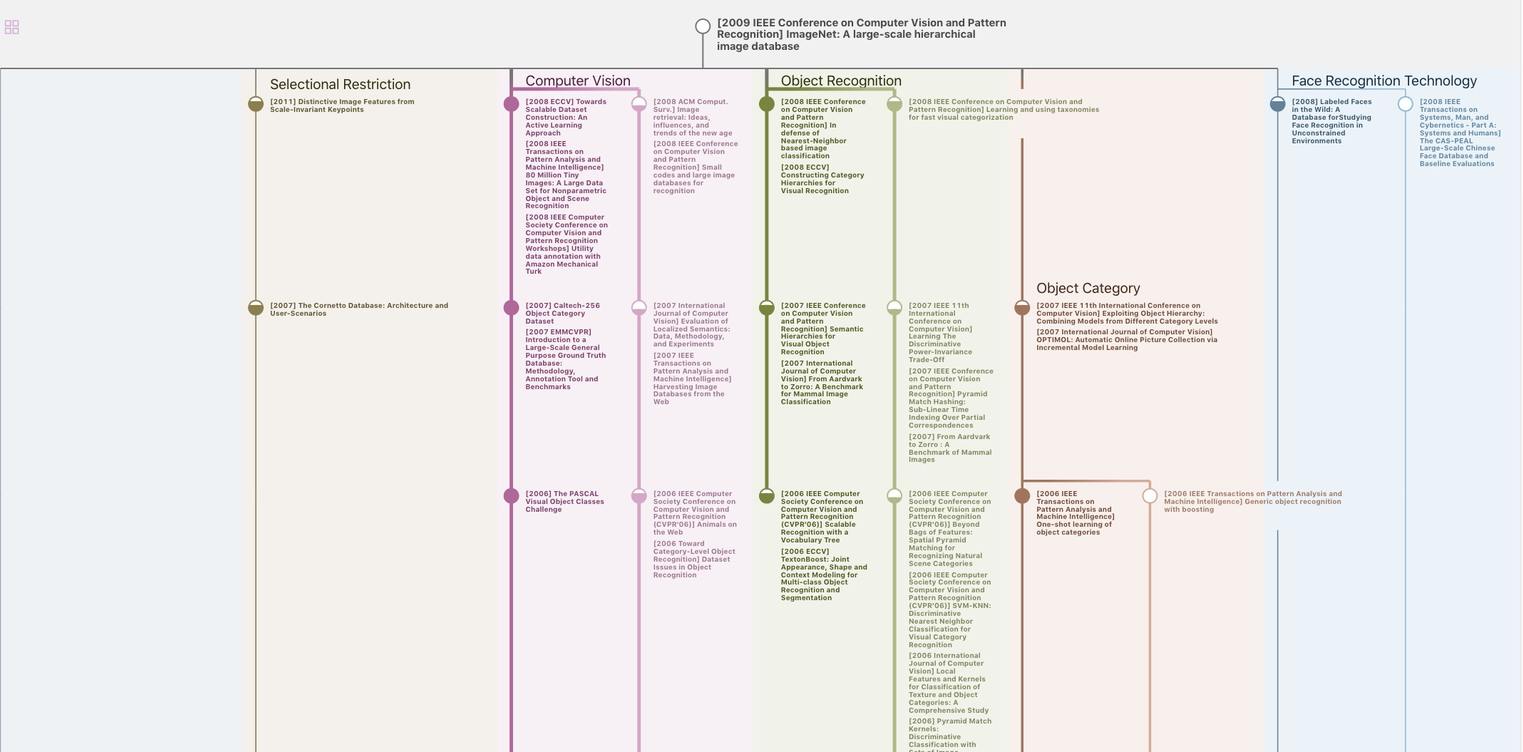
生成溯源树,研究论文发展脉络
Chat Paper
正在生成论文摘要