Hyperparameter optimization through context-based meta-reinforcement learning with task-aware representation
KNOWLEDGE-BASED SYSTEMS(2023)
摘要
In this paper, we combine context-based Meta-Reinforcement Learning with task-aware representation to efficiently overcome data-inefficiency and limited generalization in the hyperparameter optimiza-tion problem. First, we propose a new context-based meta-RL model that disentangles task inference and control, which improves the meta-training efficiency and accelerates the learning process for unseen tasks. Second, the task properties are inferred on-line, which includes not only the dataset representation but also the task-solving experience, thus encouraging the agent to explore in a much smarter fashion. Third, we employ amortized meta-learning to meta-train the agent, which is simple and runs faster than the gradient-based meta-training method. Experimental results suggest that our method can search for the optimal hyperparameter configuration with limited computational cost in a reasonable time.(c) 2022 Elsevier B.V. All rights reserved.
更多查看译文
AI 理解论文
溯源树
样例
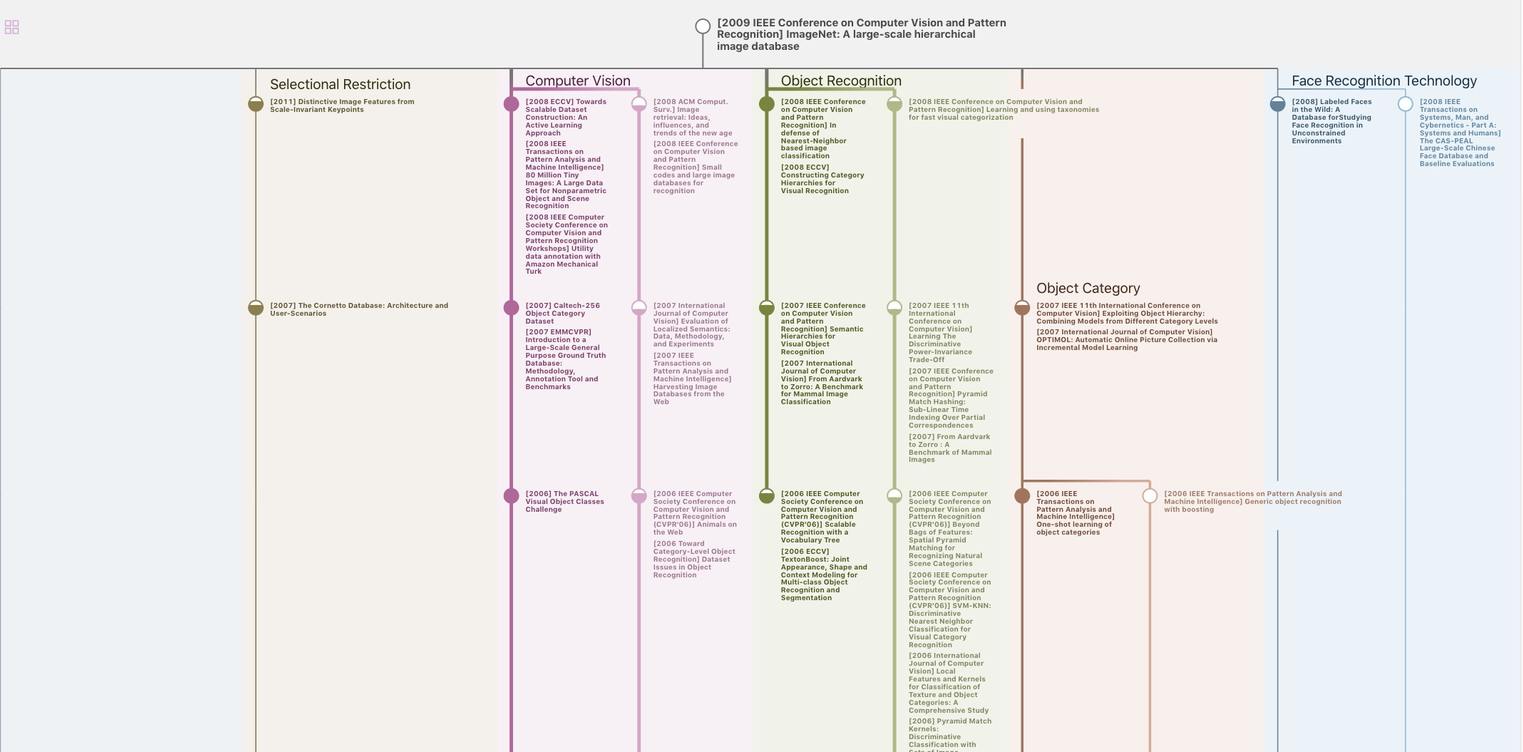
生成溯源树,研究论文发展脉络
Chat Paper
正在生成论文摘要