INTENSITY ESTIMATION ON GEOMETRIC NETWORKS WITH PENALIZED SPLINES
ANNALS OF APPLIED STATISTICS(2022)
摘要
In the past decades the growing amount of network data lead to many novel statistical models. In this paper we consider so-called geometric networks. Typical examples are road networks or other infrastructure networks. Nevertheless, the neurons or the blood vessels in a human body can also be interpreted as a geometric network embedded in a three-dimensional space. A network-specific metric, rather than the Euclidean metric, is usually used in all these applications, making the analyses of network data challenging. We consider network-based point processes, and our task is to estimate the intensity (or density) of the process which allows us to detect high- and low-intensity regions of the underlying stochastic processes. Available routines that tackle this problem are commonly based on kernel smoothing methods. This paper uses penalized spline smoothing and extends this toward smooth intensity estimation on geometric networks. Furthermore, our approach easily allows incorporating covariates, enabling us to respect the network geometry in a regression model framework. Several data examples and a simulation study show that penalized spline-based intensity estimation on geometric networks is a numerically stable and efficient tool. Furthermore, it also allows estimating linear and smooth covariate effects, distinguishing our approach from already existing methodologies.
更多查看译文
关键词
Intensity estimation of stochastic point processes, generalized additive models, geometric networks, penalized splines, poisson regression with offset, spats tat package
AI 理解论文
溯源树
样例
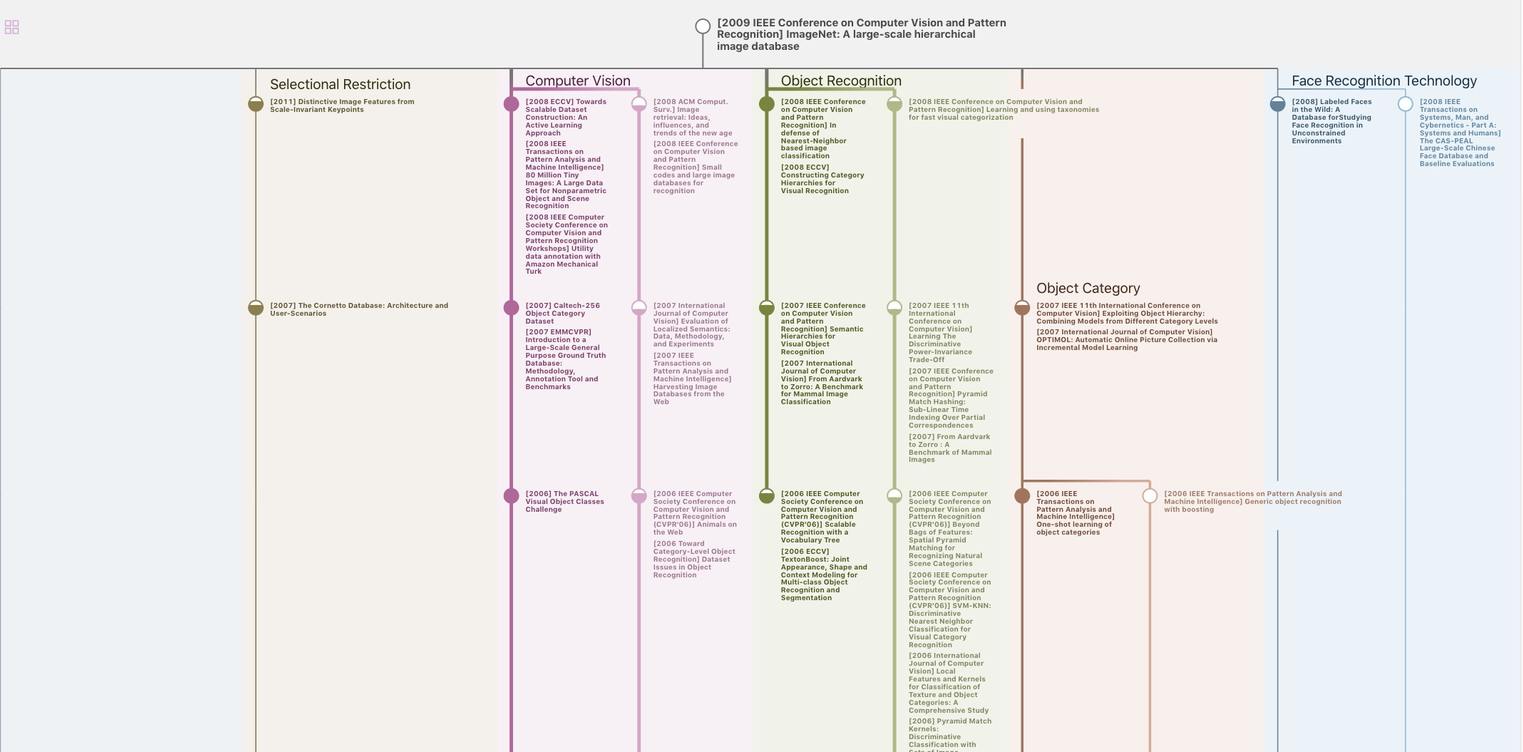
生成溯源树,研究论文发展脉络
Chat Paper
正在生成论文摘要