When Relation Networks meet GANs: Relation GANs with Triplet Loss
arxiv(2020)
摘要
Though recent research has achieved remarkable progress in generating realistic images with generative adversarial networks (GANs), the lack of training stability is still a lingering concern of most GANs, especially on high-resolution inputs and complex datasets. Since the randomly generated distribution can hardly overlap with the real distribution, training GANs often suffers from the gradient vanishing problem. A number of approaches have been proposed to address this issue by constraining the discriminator's capabilities using empirical techniques, like weight clipping, gradient penalty, spectral normalization etc. In this paper, we provide a more principled approach as an alternative solution to this issue. Instead of training the discriminator to distinguish real and fake input samples, we investigate the relationship between paired samples by training the discriminator to separate paired samples from the same distribution and those from different distributions. To this end, we explore a relation network architecture for the discriminator and design a triplet loss which performs better generalization and stability. Extensive experiments on benchmark datasets show that the proposed relation discriminator and new loss can provide significant improvement on variable vision tasks including unconditional and conditional image generation and image translation. Our source codes are available on the website: \url{https://github.com/JosephineRabbit/Relation-GAN}
更多查看译文
关键词
relation gans,relation networks
AI 理解论文
溯源树
样例
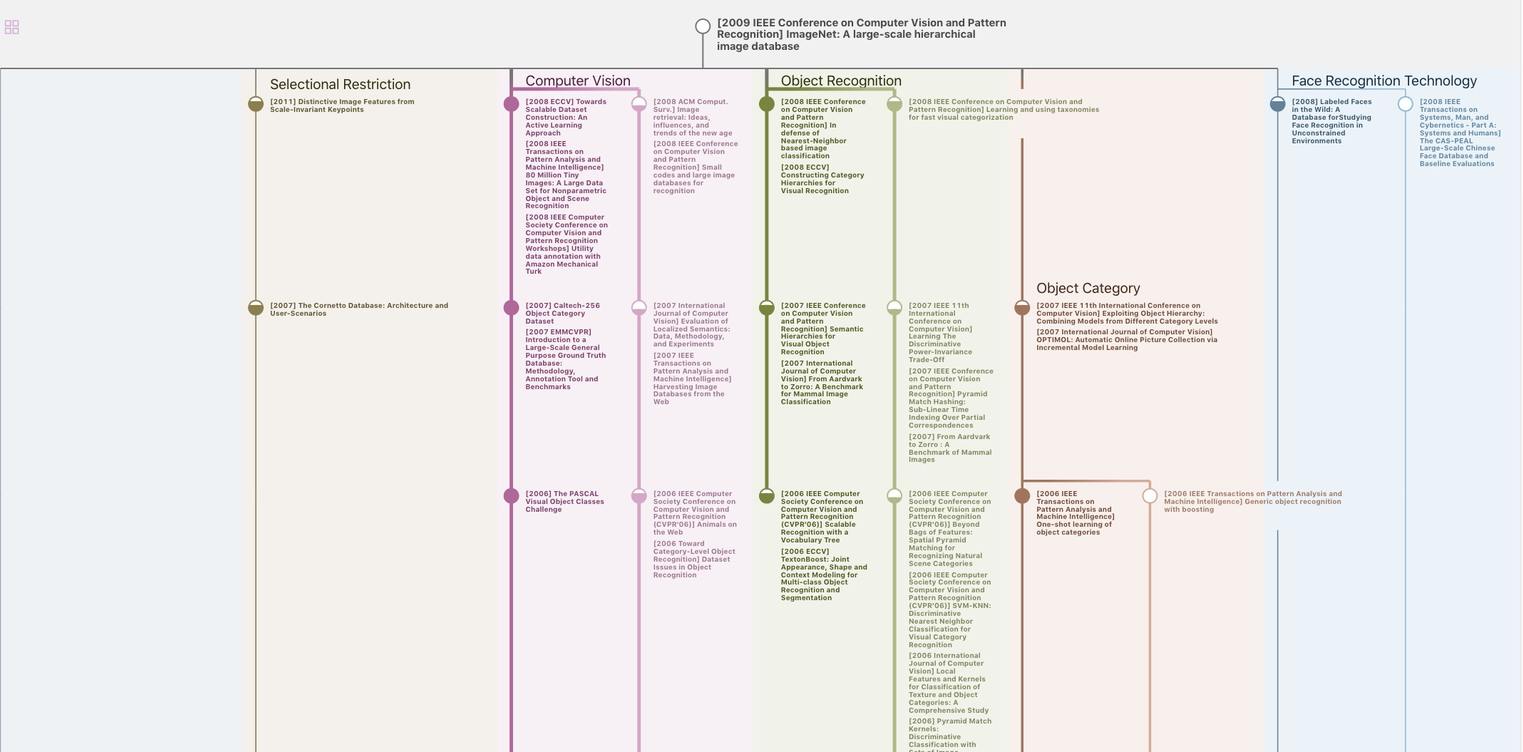
生成溯源树,研究论文发展脉络
Chat Paper
正在生成论文摘要