Classification of Kidney Cancer Data Using Cost-Sensitive Hybrid Deep Learning Approach.
SYMMETRY-BASEL(2020)
摘要
Recently, large-scale bioinformatics and genomic data have been generated using advanced biotechnology methods, thus increasing the importance of analyzing such data. Numerous data mining methods have been developed to process genomic data in the field of bioinformatics. We extracted significant genes for the prognosis prediction of 1157 patients using gene expression data from patients with kidney cancer. We then proposed an end-to-end, cost-sensitive hybrid deep learning (COST-HDL) approach with a cost-sensitive loss function for classification tasks on imbalanced kidney cancer data. Here, we combined the deep symmetric auto encoder; the decoder is symmetric to the encoder in terms of layer structure, with reconstruction loss for non-linear feature extraction and neural network with balanced classification loss for prognosis prediction to address data imbalance problems. Combined clinical data from patients with kidney cancer and gene data were used to determine the optimal classification model and estimate classification accuracy by sample type, primary diagnosis, tumor stage, and vital status as risk factors representing the state of patients. Experimental results showed that the COST-HDL approach was more efficient with gene expression data for kidney cancer prognosis than other conventional machine learning and data mining techniques. These results could be applied to extract features from gene biomarkers for prognosis prediction of kidney cancer and prevention and early diagnosis.
更多查看译文
关键词
data mining,machine learning,kidney cancer,bioinformatics,autoencoder,neural network,cost-sensitive,hybrid deep learning,cancer classification
AI 理解论文
溯源树
样例
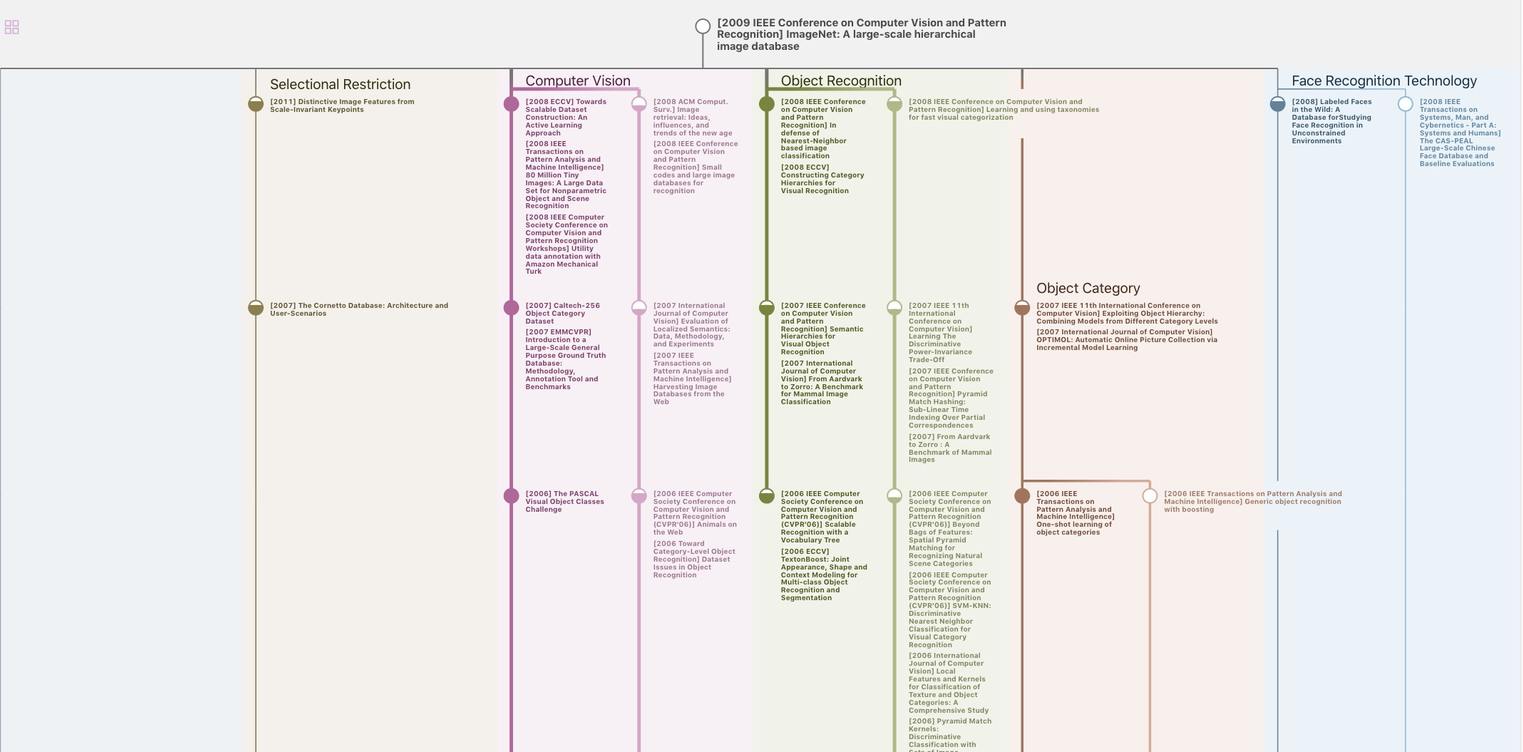
生成溯源树,研究论文发展脉络
Chat Paper
正在生成论文摘要