Transfer Learning On Decision Tree With Class Imbalance
2019 IEEE 31ST INTERNATIONAL CONFERENCE ON TOOLS WITH ARTIFICIAL INTELLIGENCE (ICTAI 2019)(2019)
摘要
Transfer learning has attracted growing attention from the machine learning community. It addresses real-world issues that were not considered in the classical methods, especially with model-based methods when source data is not available. Recent work on transfer learning for Decision Trees has been proposed in the form of two algorithms named SER and STRUT. These methods tackle with success potential changes between source and target data, however several classification tasks may face imbalance data, hence degrading the performance of such transfer methods. In this paper, we study the impact of class imbalance on these algorithms and propose an adaptation for each of them, named SER* and STRUT*. These variants have been tested on real and synthetic data, these latter revealing to be of great use to control at the same time various transformations between source and target along with imbalance conditions. Results on both types of data show the benefits of our approach and suggest several perspectives for further research.
更多查看译文
关键词
transfer learning, decision tree, random forests, class imbalance
AI 理解论文
溯源树
样例
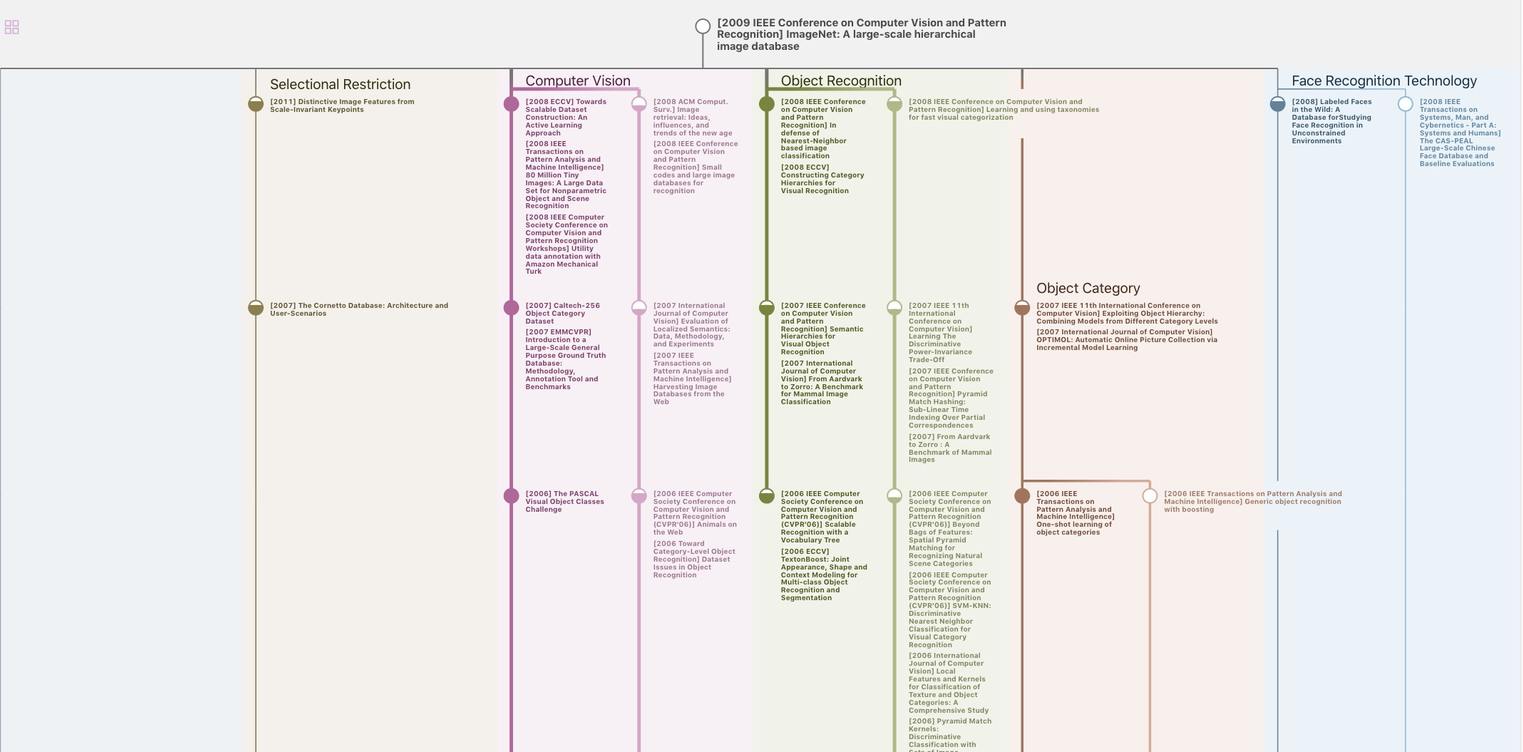
生成溯源树,研究论文发展脉络
Chat Paper
正在生成论文摘要