I-SPEC: An End-to-End Framework for Learning Transportable, Shift-Stable Models
Unknown Journal(2020)
摘要
Shifts in environment between development and deployment cause classical supervised learning to produce models that fail to generalize well to new target distributions. Recently, many solutions which find invariant predictive distributions have been developed. Among these, graph-based approaches do not require data from the target environment and can capture more stable information than alternative methods which find stable feature sets. However, these approaches assume that the data generating process is known in the form of a full causal graph, which is generally not the case. In this paper, we propose I-SPEC, an end-to-end framework that addresses this shortcoming by using data to learn a partial ancestral graph (PAG). Using the PAG we develop an algorithm that determines an interventional distribution that is stable to the declared shifts; this subsumes existing approaches which find stable feature sets that are less accurate. We apply I-SPEC to a mortality prediction problem to show it can learn a model that is robust to shifts without needing upfront knowledge of the full causal DAG.
更多查看译文
关键词
learning transportable,models,i-spec,end-to-end,shift-stable
AI 理解论文
溯源树
样例
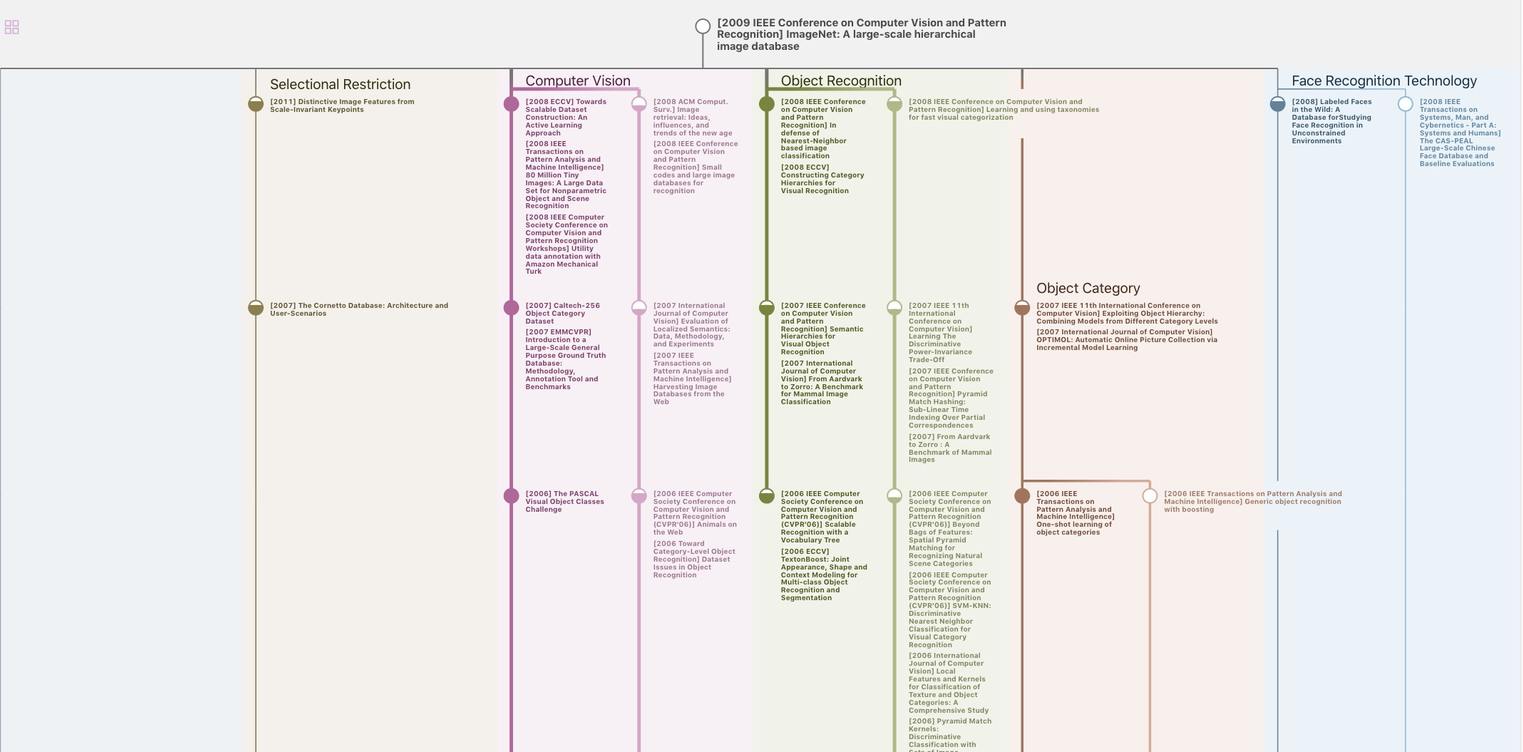
生成溯源树,研究论文发展脉络
Chat Paper
正在生成论文摘要