sKPNSGA-II: Knee point based MOEA with self-adaptive angle for Mission Planning Problems
CoRR(2020)
摘要
Real-world and complex problems have usually many objective functions that have to be optimized all at once. Over the last decades, Multi-Objective Evolutionary Algorithms (MOEAs) are designed to solve this kind of problems. Nevertheless, some problems have many objectives which lead to a large number of non-dominated solutions obtained by the optimization algorithms. The large set of non-dominated solutions hinders the selection of the most appropriate solution by the decision maker. This paper presents a new algorithm that has been designed to obtain the most significant solutions from the Pareto Optimal Frontier (POF). This approach is based on the cone-domination applied to MOEA, which can find the knee point solutions. In order to obtain the best cone angle, we propose a hypervolume-distribution metric, which is used to self-adapt the angle during the evolving process. This new algorithm has been applied to the real world application in Unmanned Air Vehicle (UAV) Mission Planning Problem. The experimental results show a significant improvement of the algorithm performance in terms of hypervolume, number of solutions, and also the required number of generations to converge.
更多查看译文
关键词
mission planning problems,moea,knee point,self-adaptive
AI 理解论文
溯源树
样例
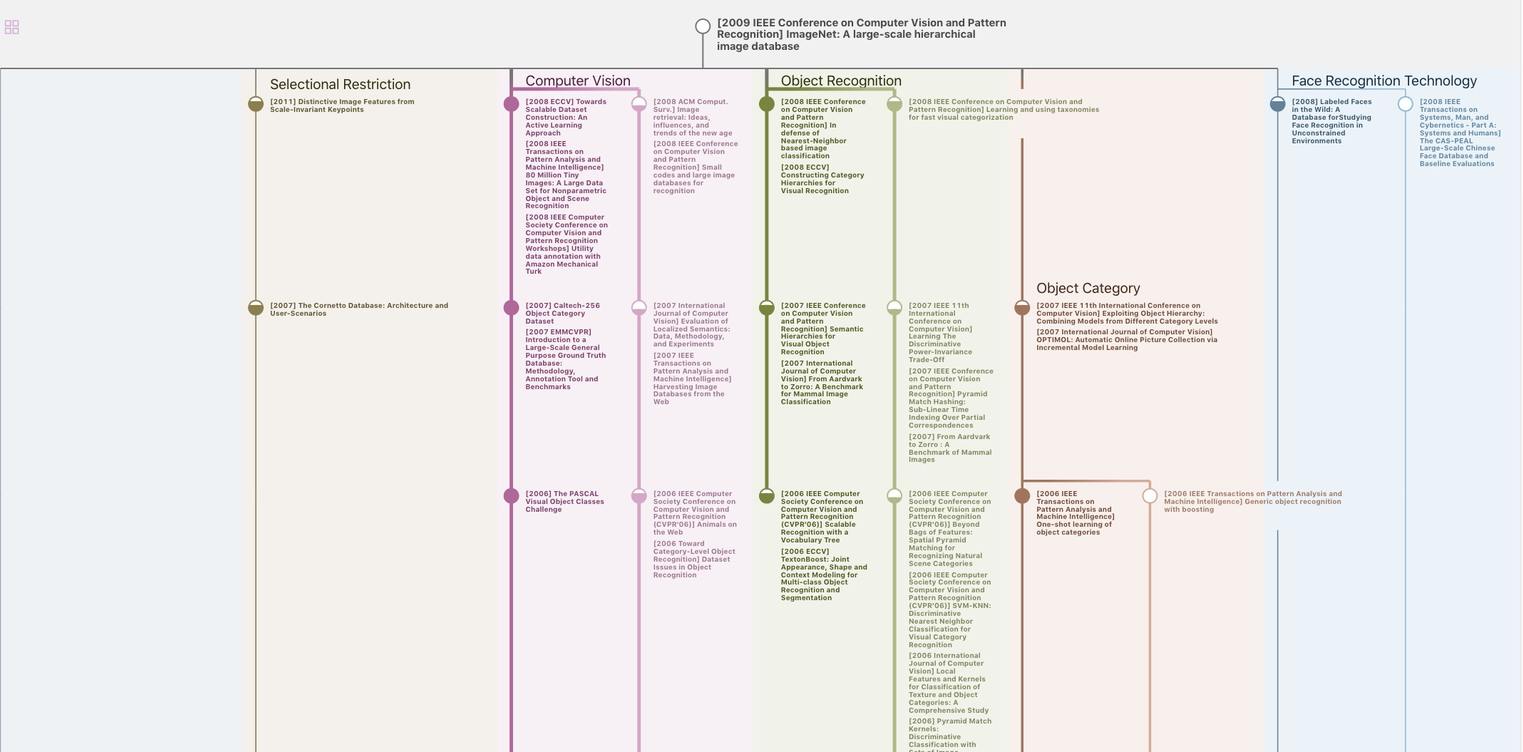
生成溯源树,研究论文发展脉络
Chat Paper
正在生成论文摘要