FrameAxis: characterizing microframe bias and intensity with word embedding
PEERJ COMPUTER SCIENCE(2021)
摘要
Framing is a process of emphasizing a certain aspect of an issue over the others, nudging readers or listeners towards different positions on the issue even without making a biased argument. Here, we propose FrameAxis, a method for characterizing documents by identifying the most relevant semantic axes ("microframes") that are overrepresented in the text using word embedding. Our unsupervised approach can be readily applied to large datasets because it does not require manual annotations. It can also provide nuanced insights by considering a rich set of semantic axes. FrameAxis is designed to quantitatively tease out two important dimensions of how microframes are used in the text. Microframe bias captures how biased the text is on a certain microframe, and microframe intensity shows how prominently a certain microframe is used. Together, they offer a detailed characterization of the text. We demonstrate that microframes with the highest bias and intensity align well with sentiment, topic, and partisan spectrum by applying FrameAxis to multiple datasets from restaurant reviews to political news. The existing domain knowledge can be incorporated into FrameAxis by using custom microframes and by using FrameAxis as an iterative exploratory analysis instrument. Additionally, we propose methods for explaining the results of FrameAxis at the level of individual words and documents. Our method may accelerate scalable and sophisticated computational analyses of framing across disciplines.
更多查看译文
关键词
Framing, Media bias, Microframe, SemAxis, Word embedding, Antonyms, Semantic Axis
AI 理解论文
溯源树
样例
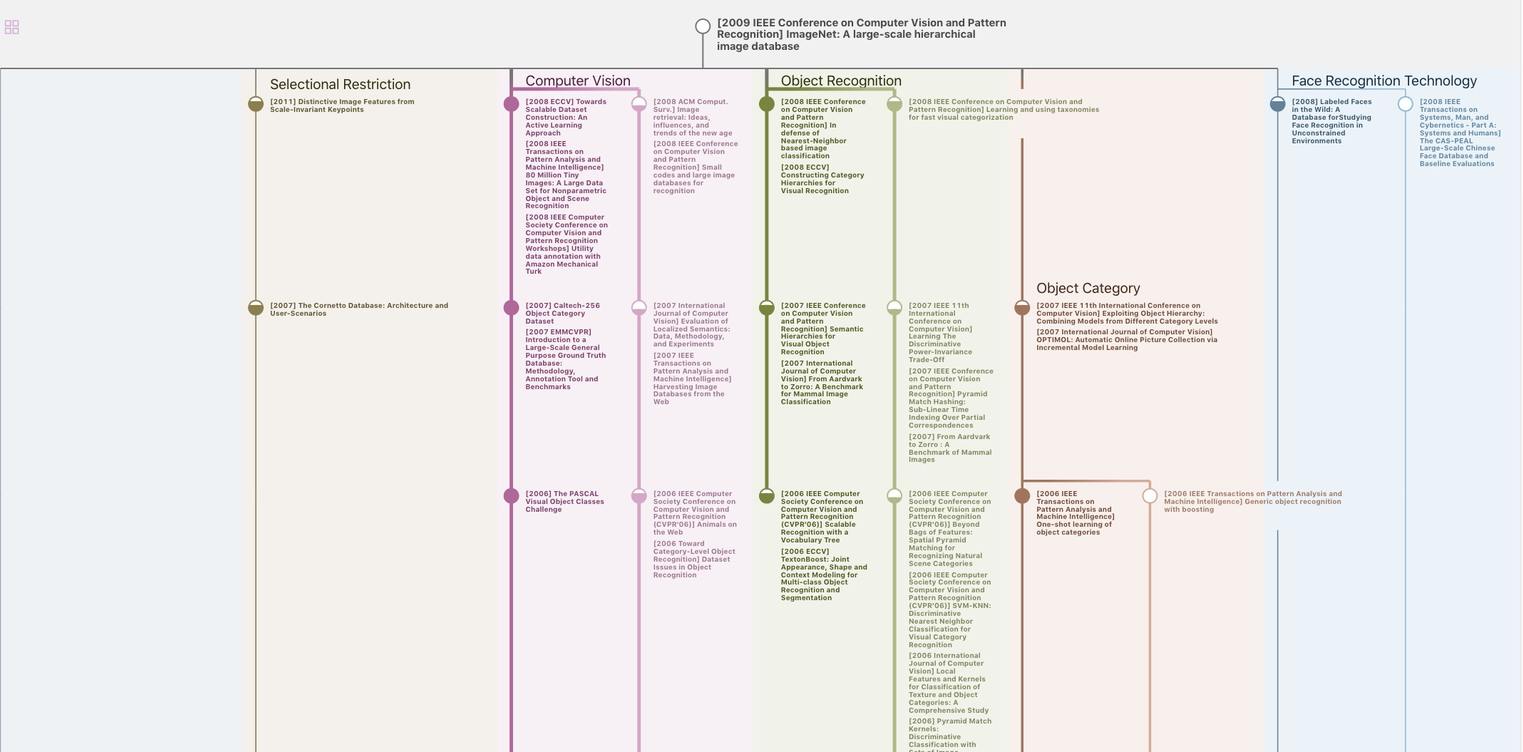
生成溯源树,研究论文发展脉络
Chat Paper
正在生成论文摘要