AdvMS: A Multi-source Multi-cost Defense Against Adversarial Attacks
ICASSP(2020)
摘要
Designing effective defense against adversarial attacks is a crucial topic as deep neural networks have been proliferated rapidly in many security-critical domains such as malware detection and self-driving cars. Conventional defense methods, although shown to be promising, are largely limited by their single-source single-cost nature: The robustness promotion tends to plateau when the defenses are made increasingly stronger while the cost tends to amplify. In this paper, we study principles of designing multi-source and multi-cost schemes where defense performance is boosted from multiple defending components. Based on this motivation, we propose a multi-source and multi-cost defense scheme, Adversarially Trained Model Switching (AdvMS), that inherits advantages from two leading schemes: adversarial training and random model switching. We show that the multi-source nature of AdvMS mitigates the performance plateauing issue and the multi-cost nature enables improving robustness at a flexible and adjustable combination of costs over different factors which can better suit specific restrictions and needs in practice.
更多查看译文
关键词
Adversarial attack, adversarial robustness, stochastic defense, adversarial training
AI 理解论文
溯源树
样例
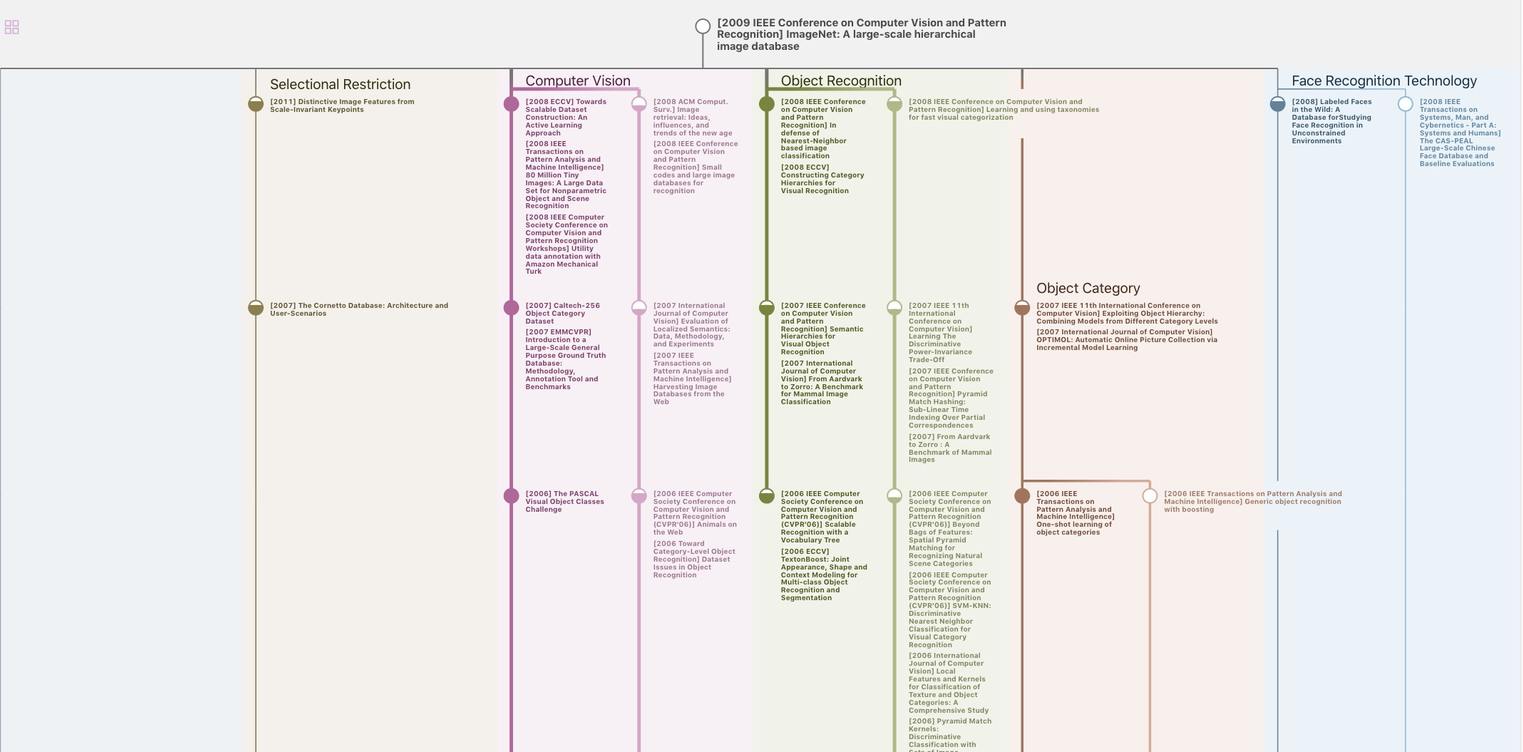
生成溯源树,研究论文发展脉络
Chat Paper
正在生成论文摘要