Privacy-Preserving Power System Obfuscation: A Bilevel Optimization Approach
IEEE Transactions on Power Systems(2020)
摘要
This paper considers the problem of releasing optimal power flow (OPF) test cases that preserve the privacy of customers (loads) using the notion of
Differential Privacy
. It is motivated by the observation that traditional differential privacy algorithms are not suitable for releasing privacy preserving OPF test cases: The added noise fundamentally changes the nature of the underlying optimization and often leads to test cases with no solutions. To remedy this limitation, the paper introduces the
OPF Load Indistinguishability
(OLI) problem, which guarantees load privacy while satisfying the OPF constraints and remaining close to the optimal dispatch cost. The paper introduces an exact mechanism, based on bilevel optimization, as well as three mechanisms that approximate the OLI problem accurately. These mechanisms enjoy desirable theoretical properties, and the computational experiments show that they produce orders of magnitude improvements over standard approaches on an extensive collection of test cases.
更多查看译文
关键词
Privacy,Optimization,Differential privacy,Generators,Load flow,Laplace equations
AI 理解论文
溯源树
样例
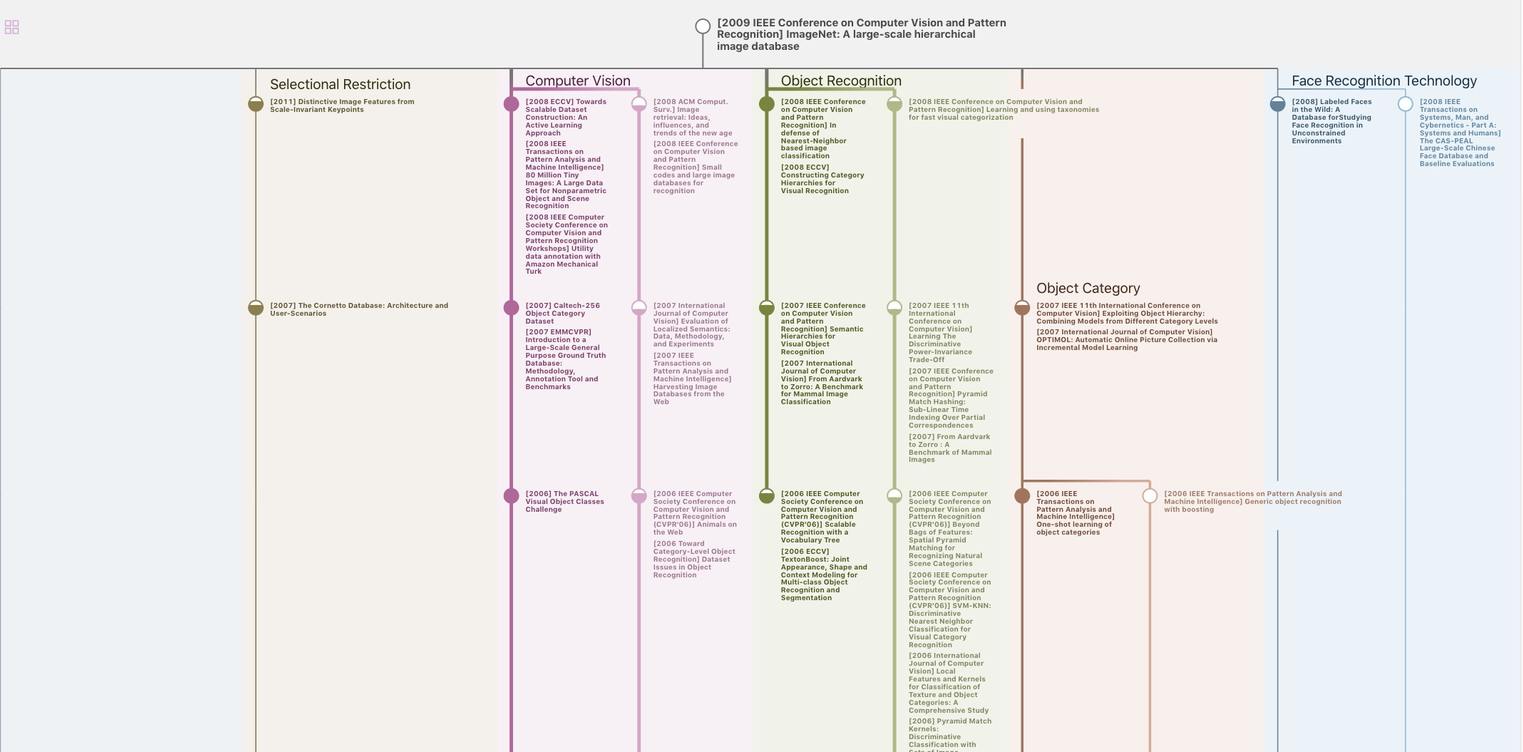
生成溯源树,研究论文发展脉络
Chat Paper
正在生成论文摘要