Hybrid Deep Neural Networks for Detection of Non-Technical Losses in Electricity Smart Meters
IEEE Transactions on Power Systems(2020)
摘要
Non-technical losses (NTL) in electricity utilities are responsible for major revenue losses. In this paper, we propose a novel end-to-end solution to self-learn the features for detecting anomalies and frauds in smart meters using a hybrid deep neural network. The network is fed with simple raw data, removing the need of handcrafted feature engineering. The proposed architecture consists of a long short-term memory network and a multi-layer perceptrons network. The first network analyses the raw daily energy consumption history whilst the second one integrates non-sequential data such as its contracted power or geographical information. The results show that the hybrid neural network significantly outperforms state-of-the-art classifiers as well as previous deep learning models used in NTL detection. The model has been trained and tested with real smart meter data of Endesa, the largest electricity utility in Spain.
更多查看译文
关键词
Inspection,Neural networks,Smart meters,History,Deep learning,Energy consumption,Data models
AI 理解论文
溯源树
样例
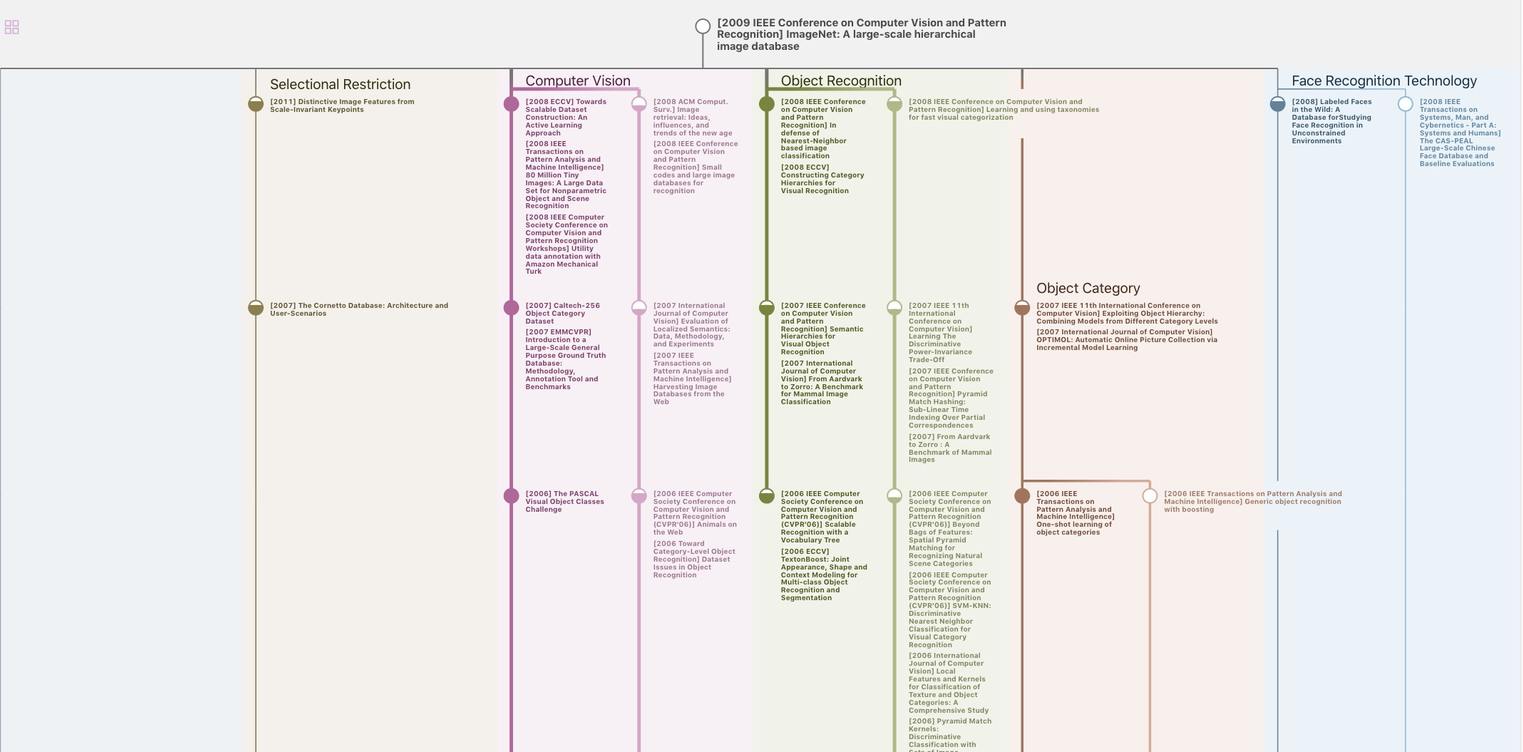
生成溯源树,研究论文发展脉络
Chat Paper
正在生成论文摘要