SYMOG: Learning symmetric mixture of Gaussian modes for improved fixed-point quantization
Neurocomputing(2020)
摘要
Deep neural networks (DNNs) have been proven to outperform classical methods on several machine learning benchmarks. However, they have high computational complexity and require powerful processing units. Especially when deployed on embedded systems, model size and inference time must be significantly reduced. We propose SYMOG (symmetric mixture of Gaussian modes), which significantly decreases the complexity of DNNs through low-bit fixed-point quantization. SYMOG is a novel soft quantization method such that the learning task and the quantization are solved simultaneously. During training the weight distribution changes from an unimodal Gaussian distribution to a symmetric mixture of Gaussians, where each mean value belongs to a particular fixed-point mode. We evaluate our approach with different architectures (LeNet5, VGG7, VGG11, DenseNet) on common benchmark data sets (MNIST, CIFAR-10, CIFAR-100) and we compare with state-of-the-art quantization approaches. We achieve excellent results and outperform 2-bit state-of-the-art performance with an error rate of only 5.71% on CIFAR-10 and 27.65% on CIFAR-100.
更多查看译文
关键词
Deep neural network,Model reduction,Fixed-point quantization,Gradient descent,Weight clipping
AI 理解论文
溯源树
样例
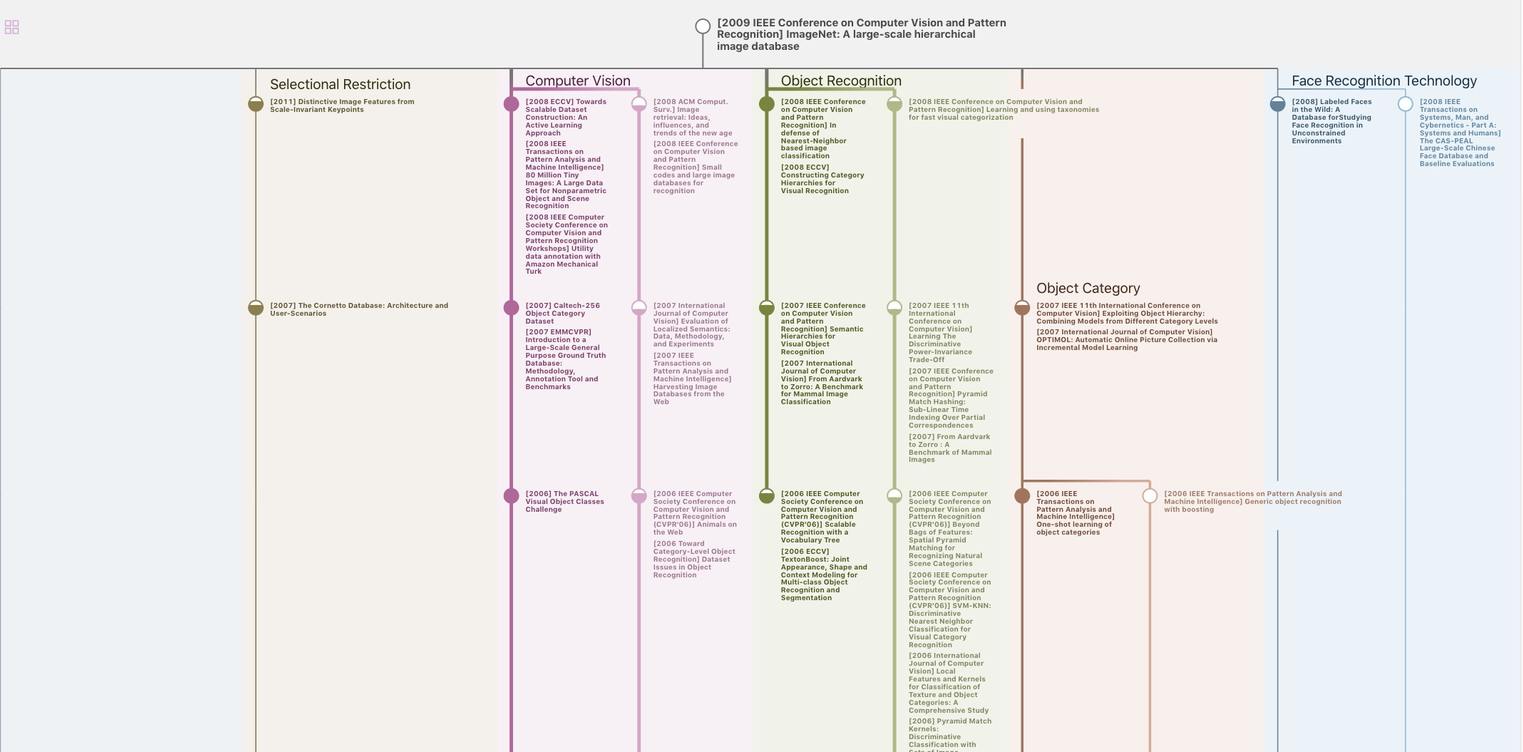
生成溯源树,研究论文发展脉络
Chat Paper
正在生成论文摘要