Mast: A Memory-Augmented Self-Supervised Tracker
2020 IEEE/CVF CONFERENCE ON COMPUTER VISION AND PATTERN RECOGNITION (CVPR)(2020)
摘要
Recent interest in self-supervised dense tracking has yielded rapid progress, but performance still remains far from supervised methods. We propose a dense tracking model trained on videos without any annotations that surpasses previous self-supervised methods on existing benchmarks by a significant margin (+15%), and achieves performance comparable to supervised methods. In this paper, we first reassess the traditional choices used for self-supervised training and reconstruction loss by conducting thorough experiments that finally elucidate the optimal choices. Second, we further improve on existing methods by augmenting our architecture with a crucial memory component. Third, we benchmark on large-scale semi-supervised video object segmentation (aka. dense tracking), and propose a new metric: generalizability. Our first two contributions yield a self-supervised network that for the first time is competitive with supervised methods on standard evaluation metrics of dense tracking. When measuring generalizability, we show self-supervised approaches are actually superior to the majority of supervised methods. We believe this new generalizability metric can better capture the real-world use-cases for dense tracking, and will spur new interest in this research direction. Code will be released at https://github.com/z1ai0/MAST.
更多查看译文
关键词
memory-augmented,self-supervised
AI 理解论文
溯源树
样例
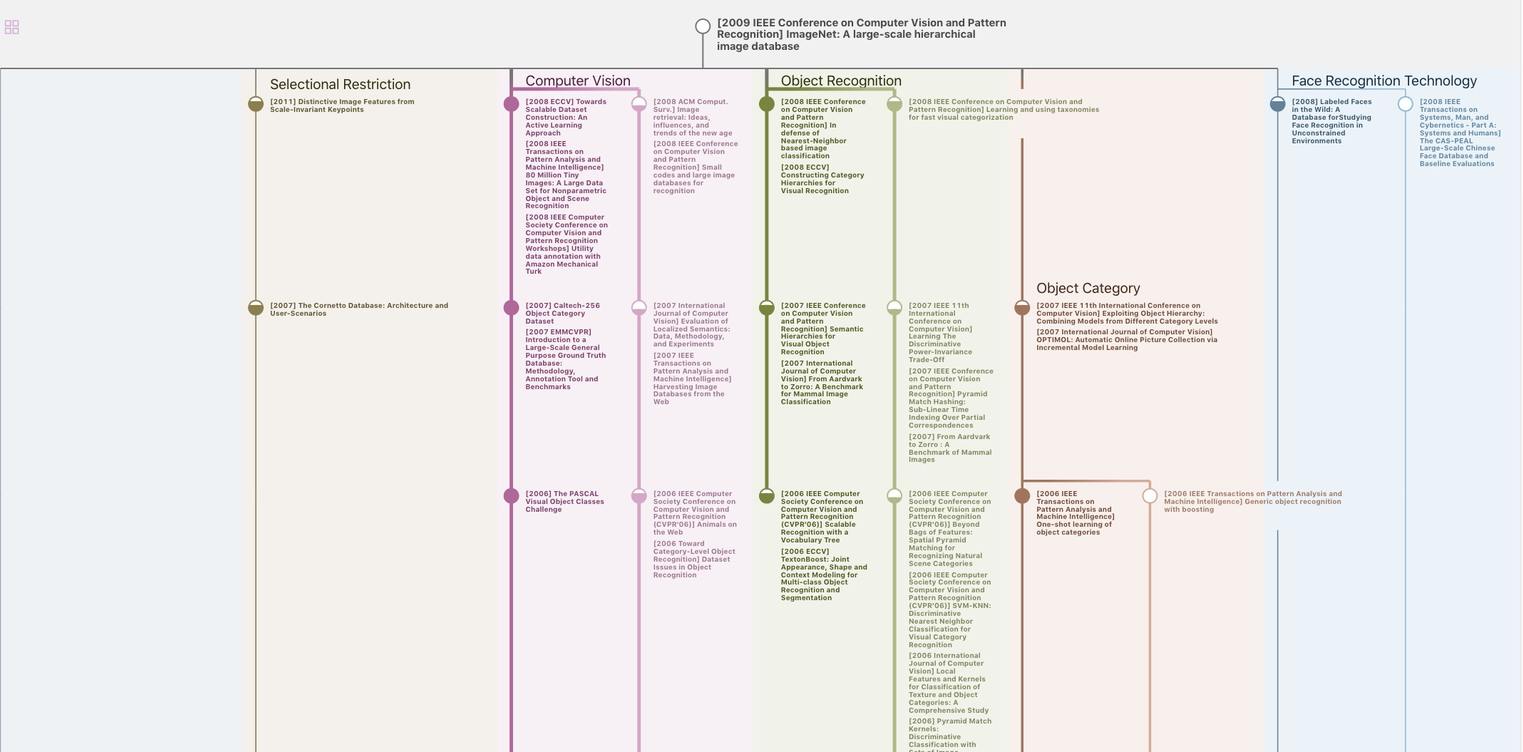
生成溯源树,研究论文发展脉络
Chat Paper
正在生成论文摘要