A New Approach to Design Symmetry Invariant Neural Networks
2021 INTERNATIONAL JOINT CONFERENCE ON NEURAL NETWORKS (IJCNN)(2021)
摘要
We investigate a new method to design G-invariant neural networks that approximate functions invariant to the action of a given permutation subgroup G of the symmetric group on input data. The key element of the new network architecture is a G-invariant transformation module, which produces a G-invariant latent representation of the input data. This latent representation is then processed with a multi-layer perceptron in the network. We prove the universality of the new architecture, discuss its properties and highlight its computational and memory efficiency. Theoretical considerations are supported by numerical experiments involving different network configurations, which demonstrate the efficiency and strong generalization properties of the new approach to design symmetry invariant neural networks, in comparison to other G-invariant neural architectures.
更多查看译文
关键词
Machine learning, neural networks, group invariance, G-invariance, geometric deep learning
AI 理解论文
溯源树
样例
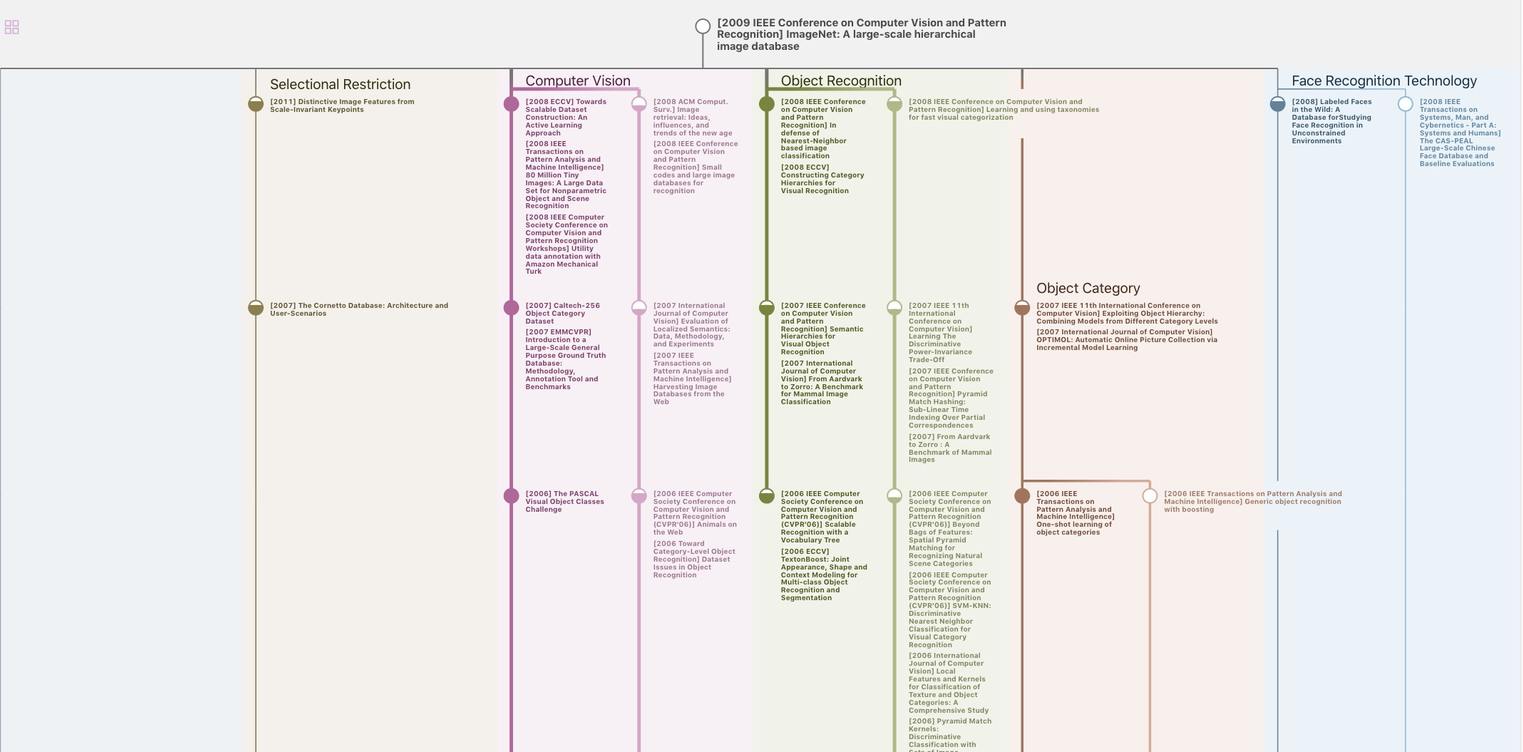
生成溯源树,研究论文发展脉络
Chat Paper
正在生成论文摘要