Self-Enhanced GNN: Improving Graph Neural Networks Using Model Outputs
2021 INTERNATIONAL JOINT CONFERENCE ON NEURAL NETWORKS (IJCNN)(2021)
摘要
Graph neural networks (GNNs) have received much attention recently because of their excellent performance on graph-based tasks. However, existing research on GNNs focuses on designing more effective models without considering much about the quality of the input data. In this paper, we propose self-enhanced GNN (SEG), which improves the quality of the input data using the outputs of existing GNN models for better performance on semi-supervised node classification. As graph data consist of both topology and node labels, we improve input data quality from both perspectives. For topology, we observe that higher classification accuracy can be achieved when the ratio of inter-class edges (connecting nodes from different classes) is low and propose topology update to remove inter-class edges and add intra-class edges. For node labels, we propose training node augmentation, which enlarges the training set using the labels predicted by existing GNN models. SEG is a general framework that can be easily combined with existing GNN models. Experimental results validate that SEG consistently improves the performance of well-known GNN models such as GCN, GAT and SGC across different datasets.
更多查看译文
关键词
graph neural networks, graph representation learning, semi-supervised node classification
AI 理解论文
溯源树
样例
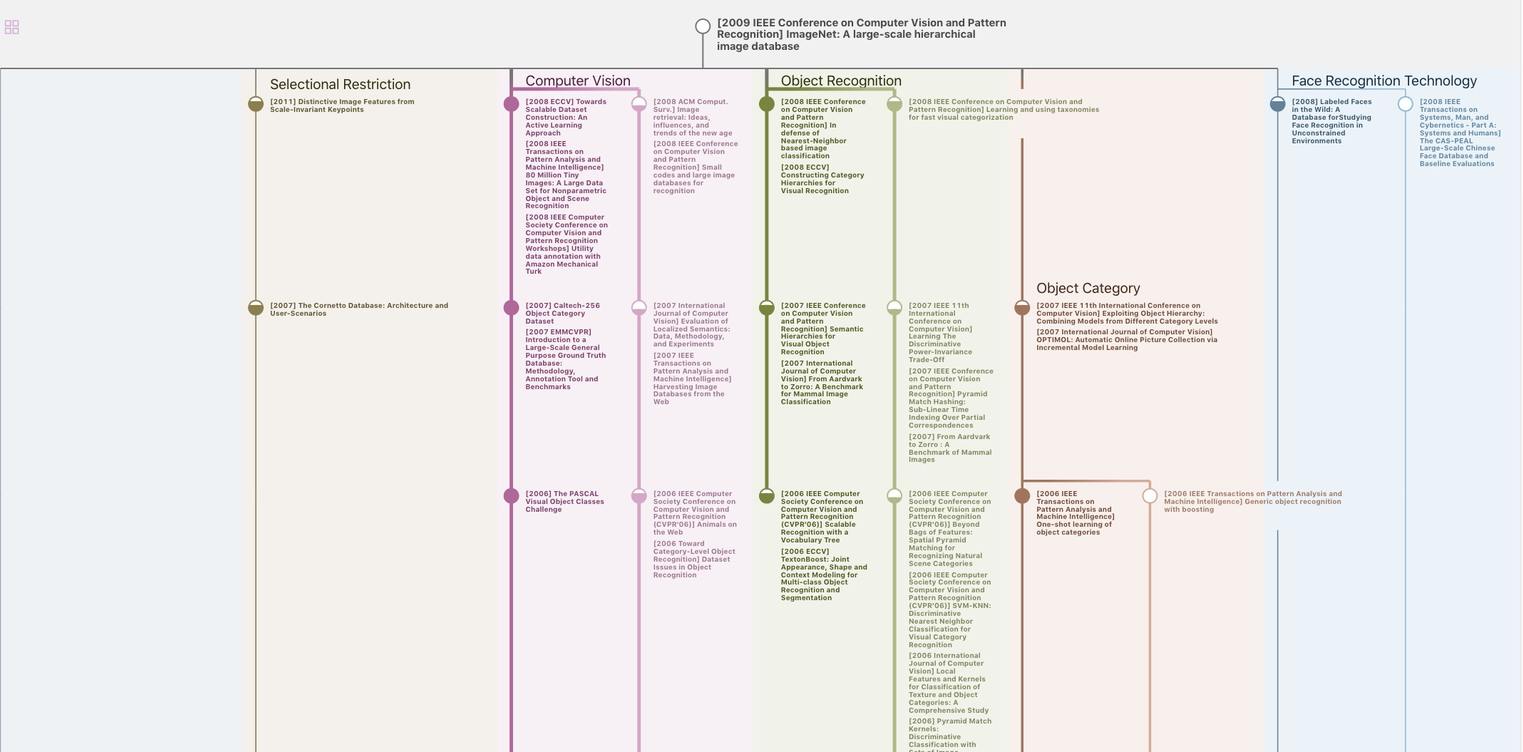
生成溯源树,研究论文发展脉络
Chat Paper
正在生成论文摘要