Dual-Attention GAN for Large-Pose Face Frontalization
2020 15th IEEE International Conference on Automatic Face and Gesture Recognition (FG 2020)(2020)
摘要
Face frontalization provides an effective and efficient way for face data augmentation and further improves the face recognition performance in extreme pose scenario. Despite recent advances in deep learning-based face synthesis approaches, this problem is still challenging due to significant pose and illumination discrepancy. In this paper, we present a novel Dual-Attention Generative Adversarial Network (DA-GAN) for photo-realistic face frontalization by capturing both contextual dependencies and local consistency during GAN training. Specifically, a self-attention-based generator is introduced to integrate local features with their long-range dependencies yielding better feature representations, and hence generate faces that preserves identities better, especially for larger pose angles. Moreover, a novel face-attention-based discriminator is applied to emphasize local features of face regions, and hence reinforce the realism of synthetic frontal faces. Guided by semantic segmentation, four independent discriminators are used to distinguish between different aspects of a face (i.e., skin, keypoints, hairline, and frontalized face). By introducing these two complementary attention mechanisms in generator and discriminator separately, we can learn a richer feature representation and generate identity preserving inference of frontal views with much finer details (i.e., more accurate facial appearance and textures) comparing to the state-of-the-art. Quantitative and qualitative experimental results demonstrate the effectiveness and efficiency of our DA-GAN approach.
更多查看译文
关键词
face frontalization,attention,GAN,face synthesis
AI 理解论文
溯源树
样例
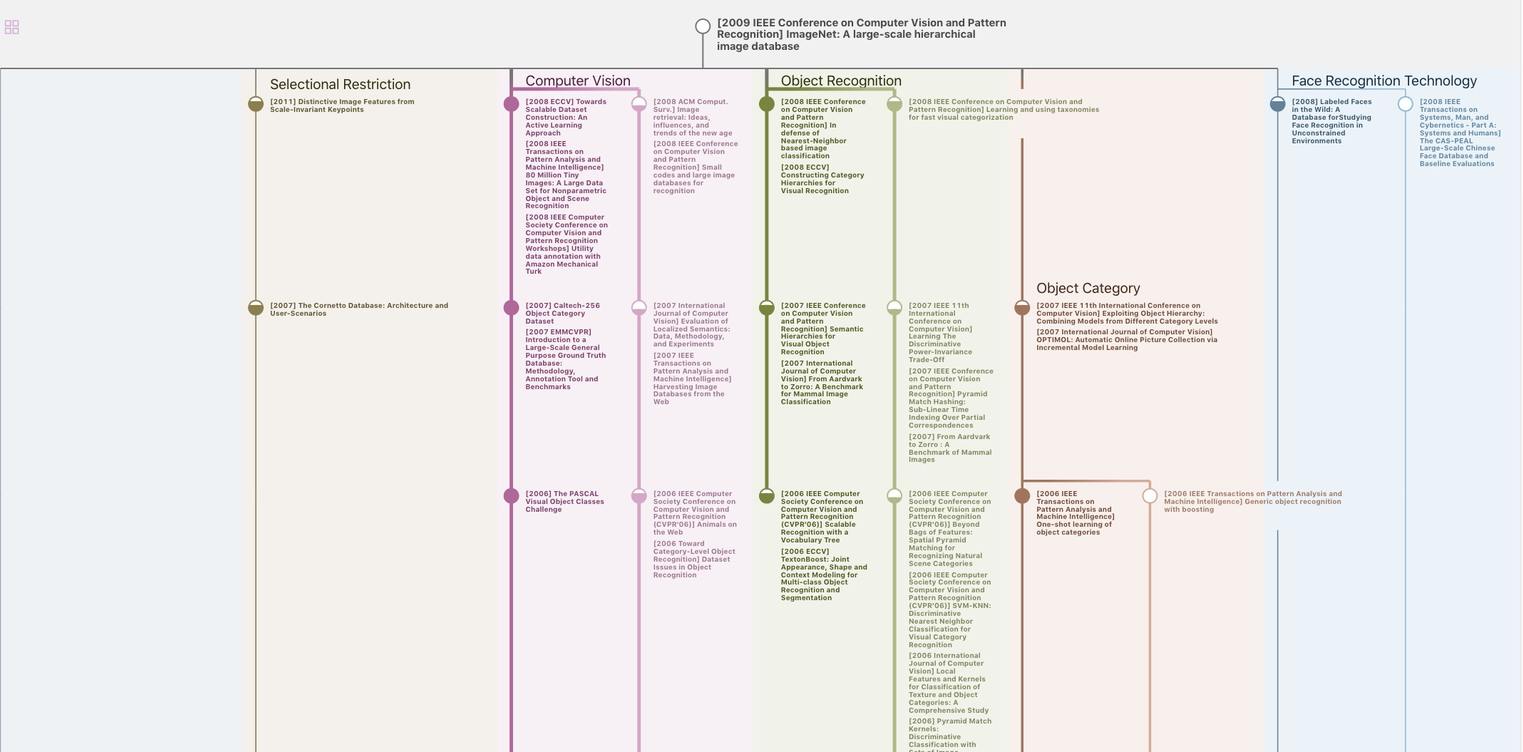
生成溯源树,研究论文发展脉络
Chat Paper
正在生成论文摘要