Deep Domain Adaptive Object Detection: a Survey
SSCI(2020)
摘要
Deep learning (DL) based object detection has achieved great progress. These methods typically assume that large amount of labeled training data is available, and training and test data are drawn from an identical distribution. However, the two assumptions are not always hold in practice. Deep domain adaptive object detection (DDAOD) has emerged as a new learning paradigm to address the above mentioned challenges. This paper aims to review the state-of-the-art progress on deep domain adaptive object detection approaches. Firstly, we introduce briefly the basic concepts of deep domain adaptation. Secondly, the deep domain adaptive detectors are classified into four categories and detailed descriptions of representative methods in each category are provided. Finally, insights for future research trend are presented.
更多查看译文
关键词
object detection,deep domain adaptation,and adaptive object detection
AI 理解论文
溯源树
样例
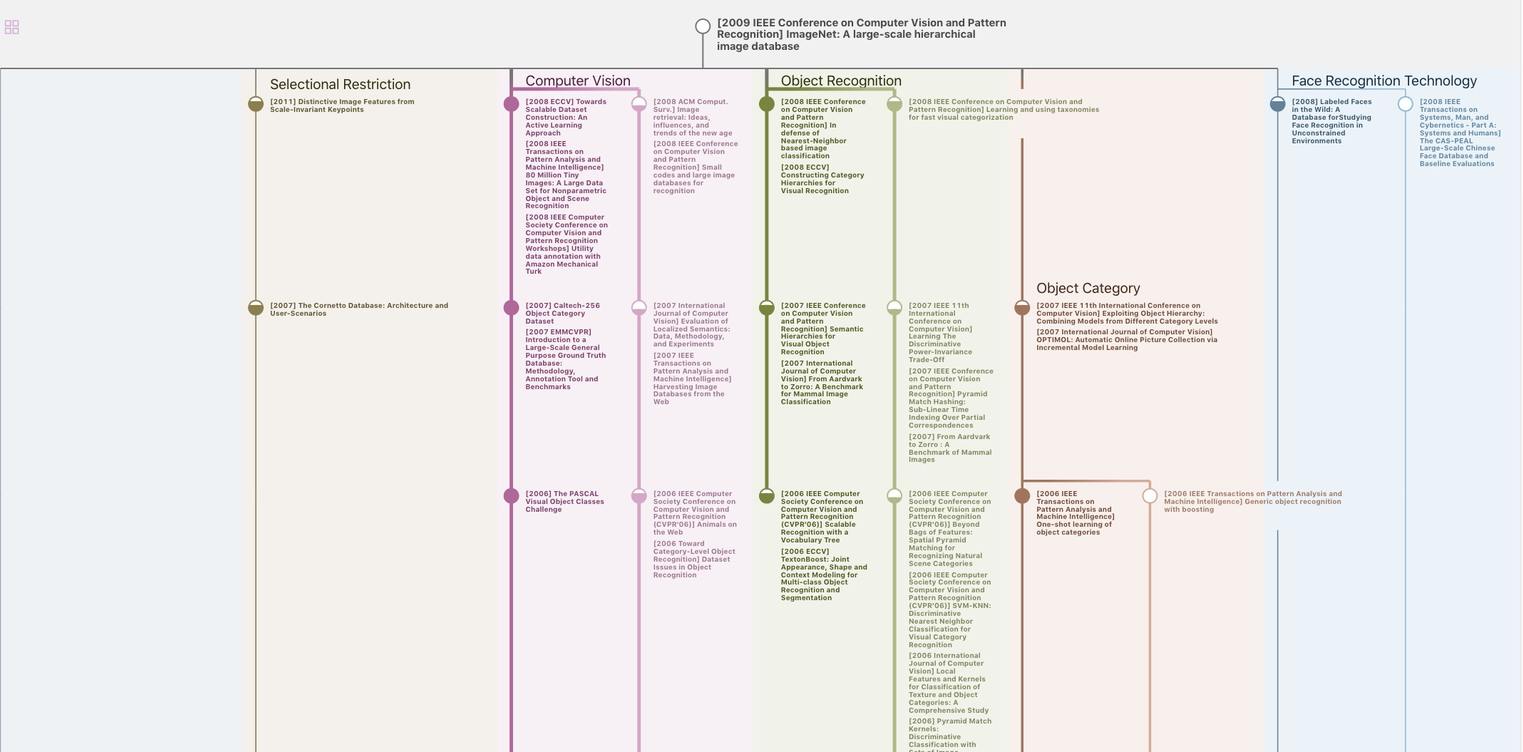
生成溯源树,研究论文发展脉络
Chat Paper
正在生成论文摘要