Directional Deep Embedding and Appearance Learning for Fast Video Object Segmentation
IEEE Transactions on Neural Networks and Learning Systems(2022)
摘要
Most recent semisupervised video object segmentation (VOS) methods rely on fine-tuning deep convolutional neural networks online using the given mask of the first frame or predicted masks of subsequent frames. However, the online fine-tuning process is usually time-consuming, limiting the practical use of such methods. We propose a directional deep embedding and appearance learning (DDEAL) method, which is free of the online fine-tuning process, for fast VOS. First, a global directional matching module (GDMM), which can be efficiently implemented by parallel convolutional operations, is proposed to learn a semantic pixel-wise embedding as an internal guidance. Second, an effective directional appearance model-based statistics is proposed to represent the target and background on a spherical embedding space for VOS. Equipped with the GDMM and the directional appearance model learning module, DDEAL learns static cues from the labeled first frame and dynamically updates cues of the subsequent frames for object segmentation. Our method exhibits the state-of-the-art VOS performance without using online fine-tuning. Specifically, it achieves a
${\mathcal{ J}}$
&
${\mathcal{ F}}$
mean score of 74.8% on DAVIS 2017 data set and an overall score
${\mathcal{ G}}$
of 71.3% on the large-scale YouTube-VOS data set, while retaining a speed of 25 fps with a single NVIDIA TITAN Xp GPU. Furthermore, our faster version runs 31 fps with only a little accuracy loss.
更多查看译文
关键词
Deep appearance learning,directional deep embedding learning,directional statistics-based learning,video object segmentation (VOS)
AI 理解论文
溯源树
样例
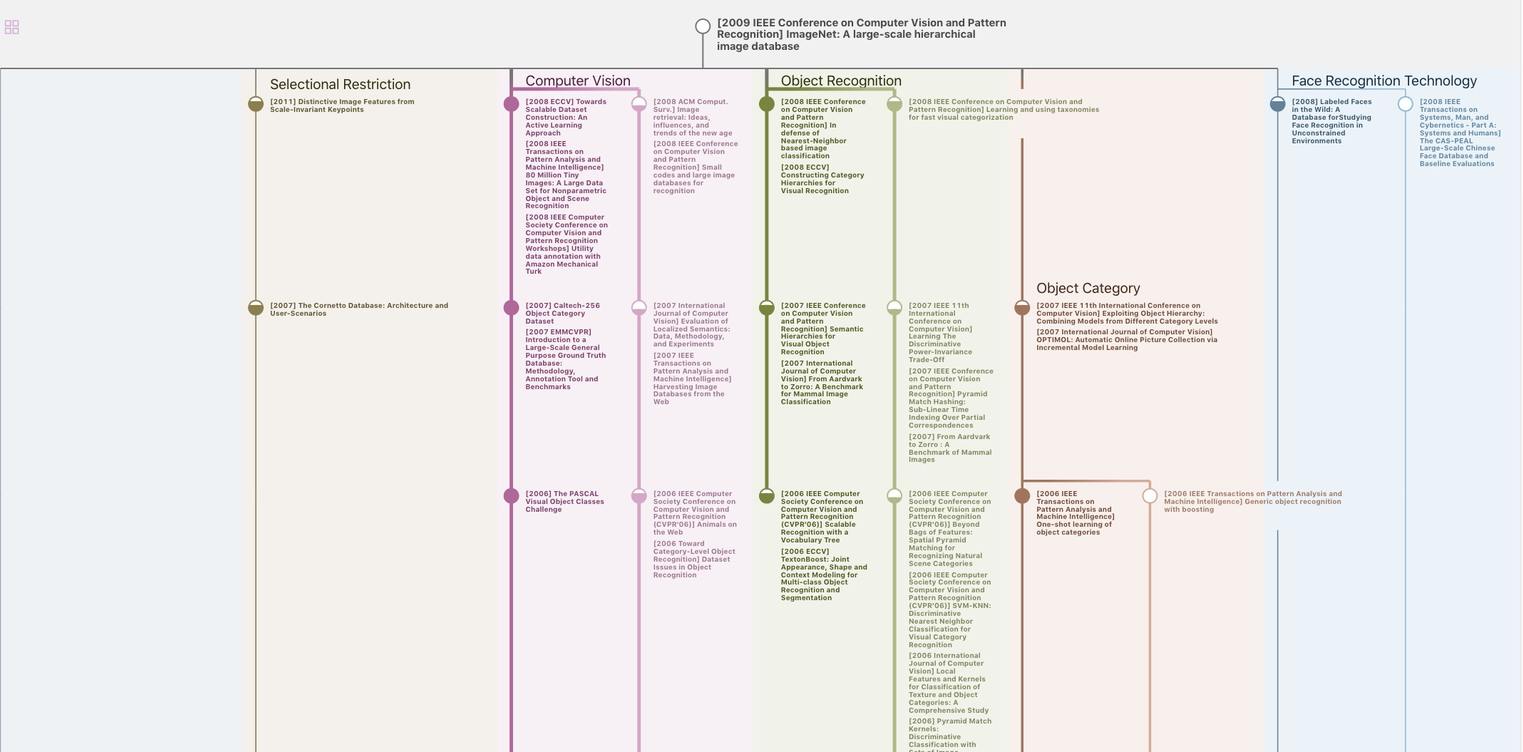
生成溯源树,研究论文发展脉络
Chat Paper
正在生成论文摘要