Combining Multiple one-class Classifiers for Anomaly based Face Spoofing Attack Detection
2019 International Conference on Biometrics (ICB)(2019)
摘要
One-class spoofing detection approaches have been an effective alternative to the two-class learners in the face presentation attack detection particularly in unseen attack scenarios. We propose an ensemble based anomaly detection approach applicable to one-class classifiers. A new score normalisation method is proposed to normalise the output of individual outlier detectors before fusion. To comply with the accuracy and diversity objectives for the component classifiers, three different strategies are utilised to build a pool of anomaly experts. To boost the performance, we also make use of the client-specific information both in the design of individual experts as well as in setting a distinct threshold for each client. We carry out extensive experiments on three face anti-spoofing datasets and show that the proposed ensemble approaches are comparable superior to the techniques based on the two-class formulation or class-independent settings.
更多查看译文
关键词
deep convolutional neural networks,pretrained neural networks,CNN architectures,score normalisation,one-class spoofing detection,face antispoofing datasets,multiple one-class classifiers,face presentation attack detection,anomaly based face spoofing attack detection
AI 理解论文
溯源树
样例
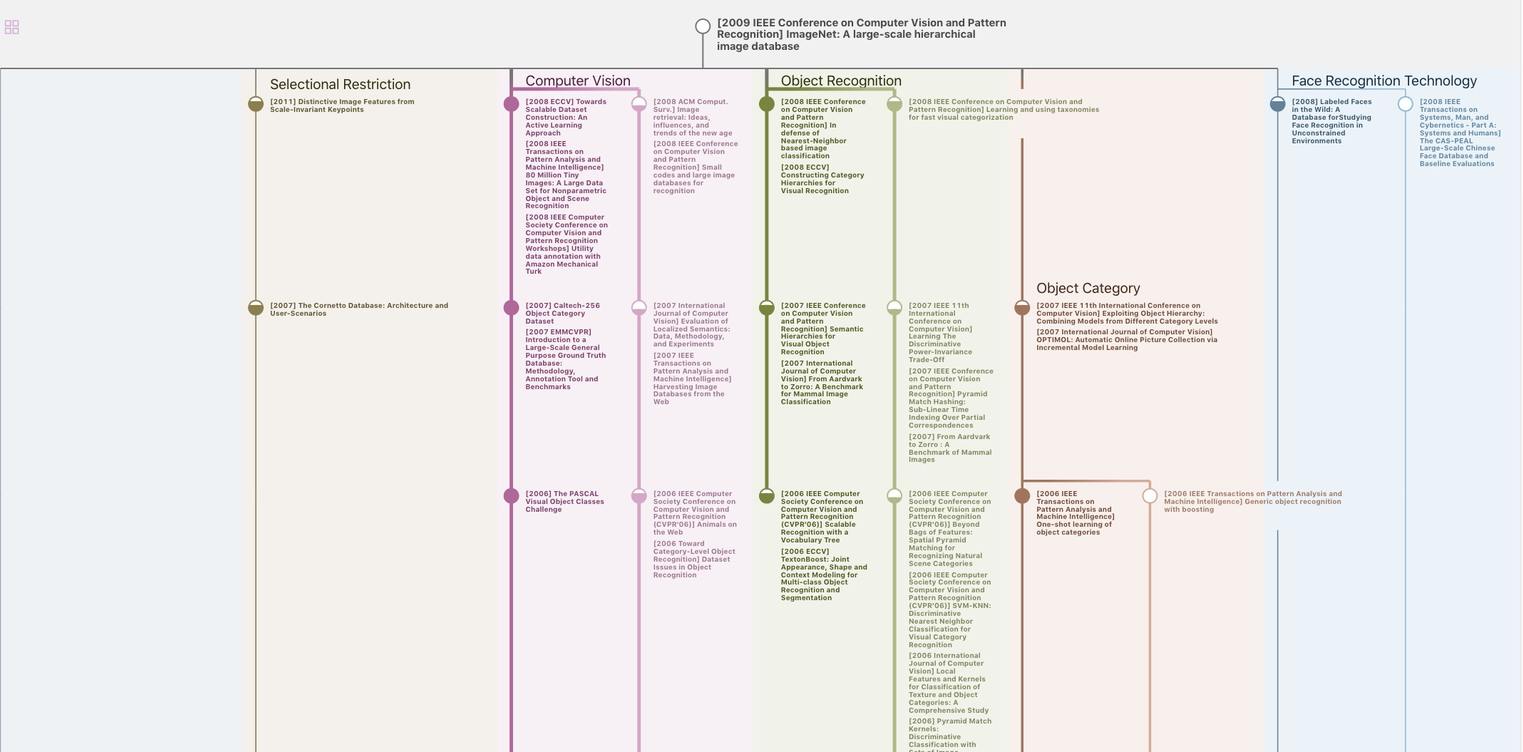
生成溯源树,研究论文发展脉络
Chat Paper
正在生成论文摘要