Cleaning Denial Constraint Violations through Relaxation
SIGMOD/PODS '20: International Conference on Management of Data Portland OR USA June, 2020(2020)
摘要
Data cleaning is a time-consuming process that depends on the data analysis that users perform. Existing solutions treat data cleaning as a separate offline process that takes place before analysis begins. Applying data cleaning before analysis assumes a priori knowledge of the inconsistencies and the query workload, thereby requiring effort on understanding and cleaning the data that is unnecessary for the analysis. We propose an approach that performs probabilistic repair of denial constraint violations on-demand, driven by the exploratory analysis that users perform. We introduce Daisy, a system that seamlessly integrates data cleaning into the analysis by relaxing query results. Daisy executes analytical query-workloads over dirty data by weaving cleaning operators into the query plan. Our evaluation shows that Daisy adapts to the workload and outperforms traditional offline cleaning on both synthetic and real-world workloads.
更多查看译文
关键词
data cleaning, denial constraints, adaptive cleaning
AI 理解论文
溯源树
样例
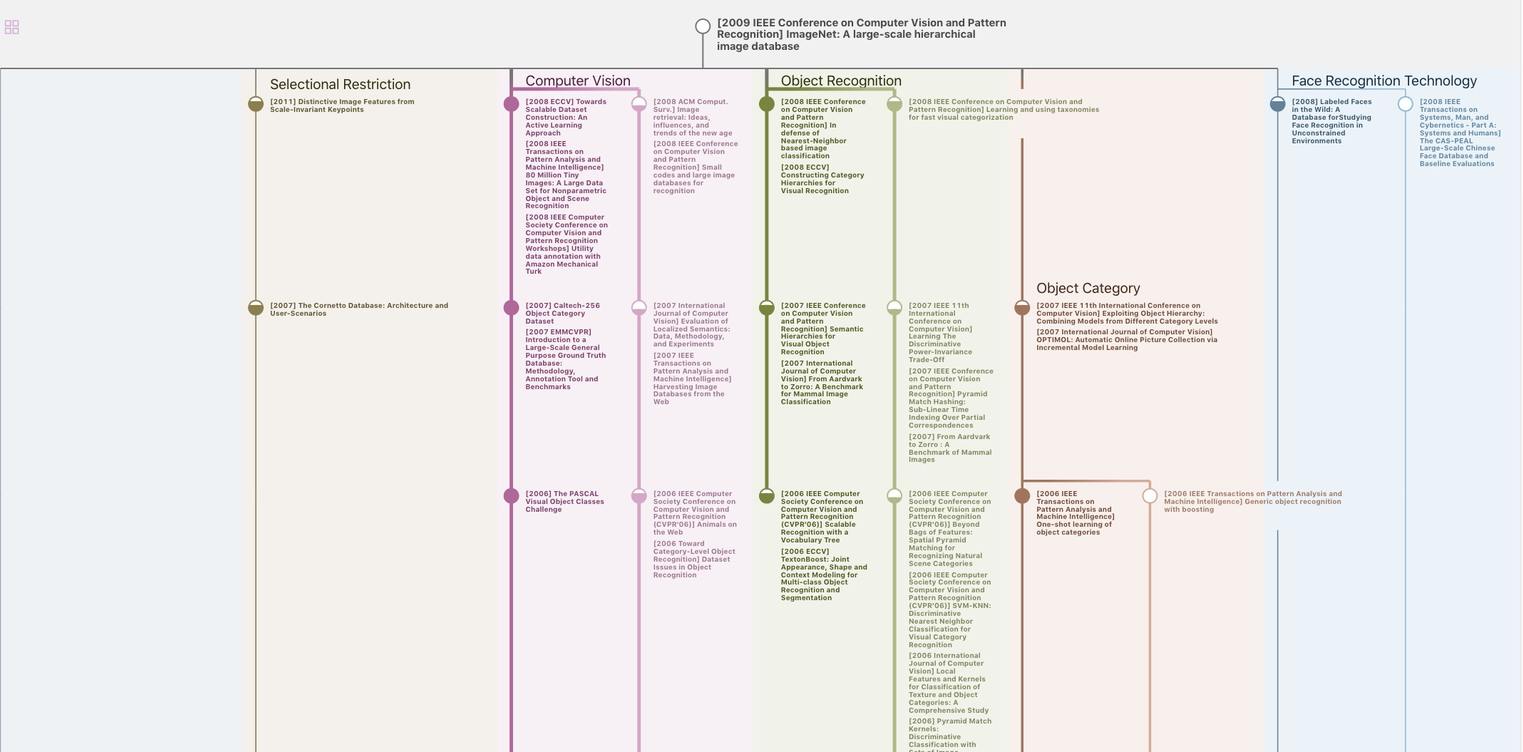
生成溯源树,研究论文发展脉络
Chat Paper
正在生成论文摘要