Scalable Neural Methods for Reasoning With a Symbolic Knowledge Base
ICLR(2020)
摘要
We describe a novel way of representing a symbolic knowledge base (KB) called a sparse-matrix reified KB. This representation enables neural modules that are fully differentiable, faithful to the original semantics of the KB, expressive enough to model multi-hop inferences, and scalable enough to use with realistically large KBs. The sparse-matrix reified KB can be distributed across multiple GPUs, can scale to tens of millions of entities and facts, and is orders of magnitude faster than naive sparse-matrix implementations. The reified KB enables very simple end-to-end architectures to obtain competitive performance on several benchmarks representing two families of tasks: KB completion, and learning semantic parsers from denotations.
更多查看译文
关键词
question-answering, knowledge base completion, neuro-symbolic reasoning, multihop reasoning
AI 理解论文
溯源树
样例
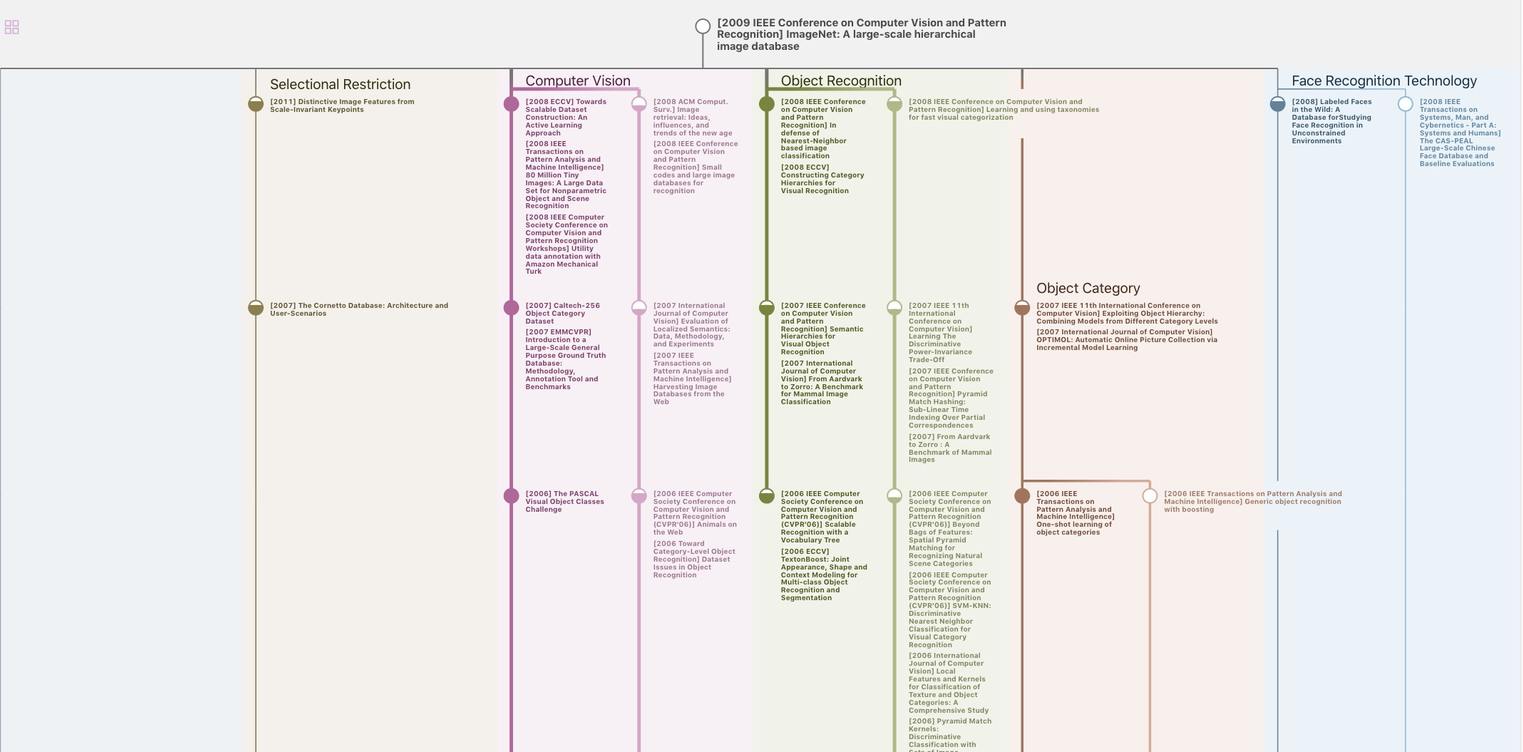
生成溯源树,研究论文发展脉络
Chat Paper
正在生成论文摘要