A general theory of identification
arxiv(2020)
摘要
What does it mean to say that a quantity is identifiable from the data? Statisticians seem to agree on a definition in the context of parametric statistical models --- roughly, a parameter $\theta$ in a model $\mathcal{P} = \{P_\theta: \theta \in \Theta\}$ is identifiable if the mapping $\theta \mapsto P_\theta$ is injective. This definition raises important questions: Are parameters the only quantities that can be identified? Is the concept of identification meaningful outside of parametric statistics? Does it even require the notion of a statistical model? Partial and idiosyncratic answers to these questions have been discussed in econometrics, biological modeling, and in some subfields of statistics like causal inference. This paper proposes a unifying theory of identification that incorporates existing definitions for parametric and nonparametric models and formalizes the process of identification analysis. The applicability of this framework is illustrated through a series of examples and two extended case studies.
更多查看译文
关键词
identification,general theory
AI 理解论文
溯源树
样例
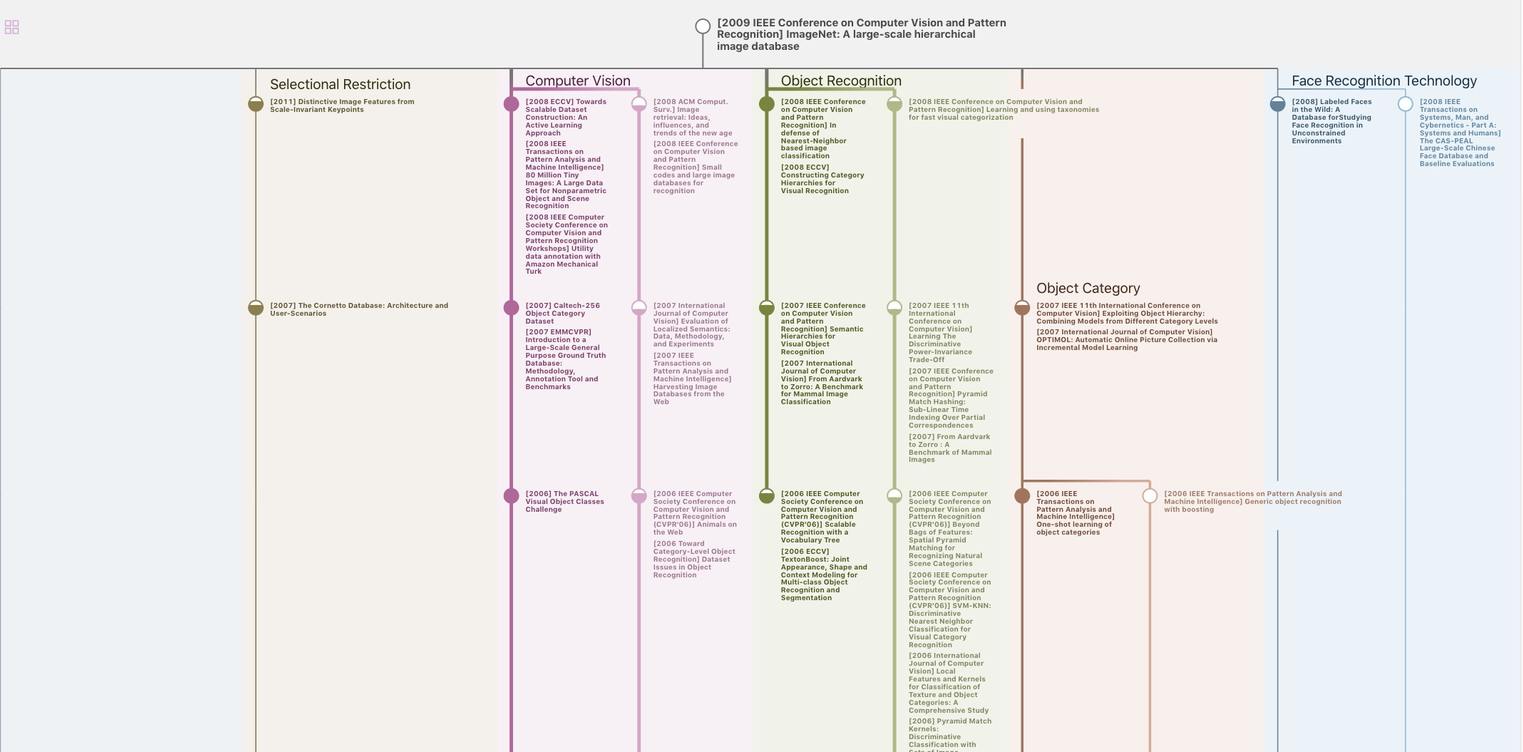
生成溯源树,研究论文发展脉络
Chat Paper
正在生成论文摘要