Learning Functionally Decomposed Hierarchies For Continuous Control Tasks With Path Planning
IEEE ROBOTICS AND AUTOMATION LETTERS(2021)
摘要
We present HiDe, a novel hierarchical reinforcement learning architecture that successfully solves long horizon control tasks and generalizes to unseen test scenarios. Functional decomposition between planning and low-level control is achieved by explicitly separating the state-action spaces across the hierarchy, which allows the integration of task-relevant knowledge per layer. We propose an RL-based planner to efficiently leverage the information in the planning layer of the hierarchy, while the control layer learns a goal-conditioned control policy. The hierarchy is trained jointly but allows for the modular transfer of policy layers across hierarchies of different agents. We experimentally show that our method generalizes across unseen test environments and can scale to 3x horizon length compared to both learning and non-learning based methods. We evaluate on complex continuous control tasks with sparse rewards, including navigation and robot manipulation.
更多查看译文
关键词
Hierarchical reinforcement learning, motion and path planning, reinforcement learning, transfer learning
AI 理解论文
溯源树
样例
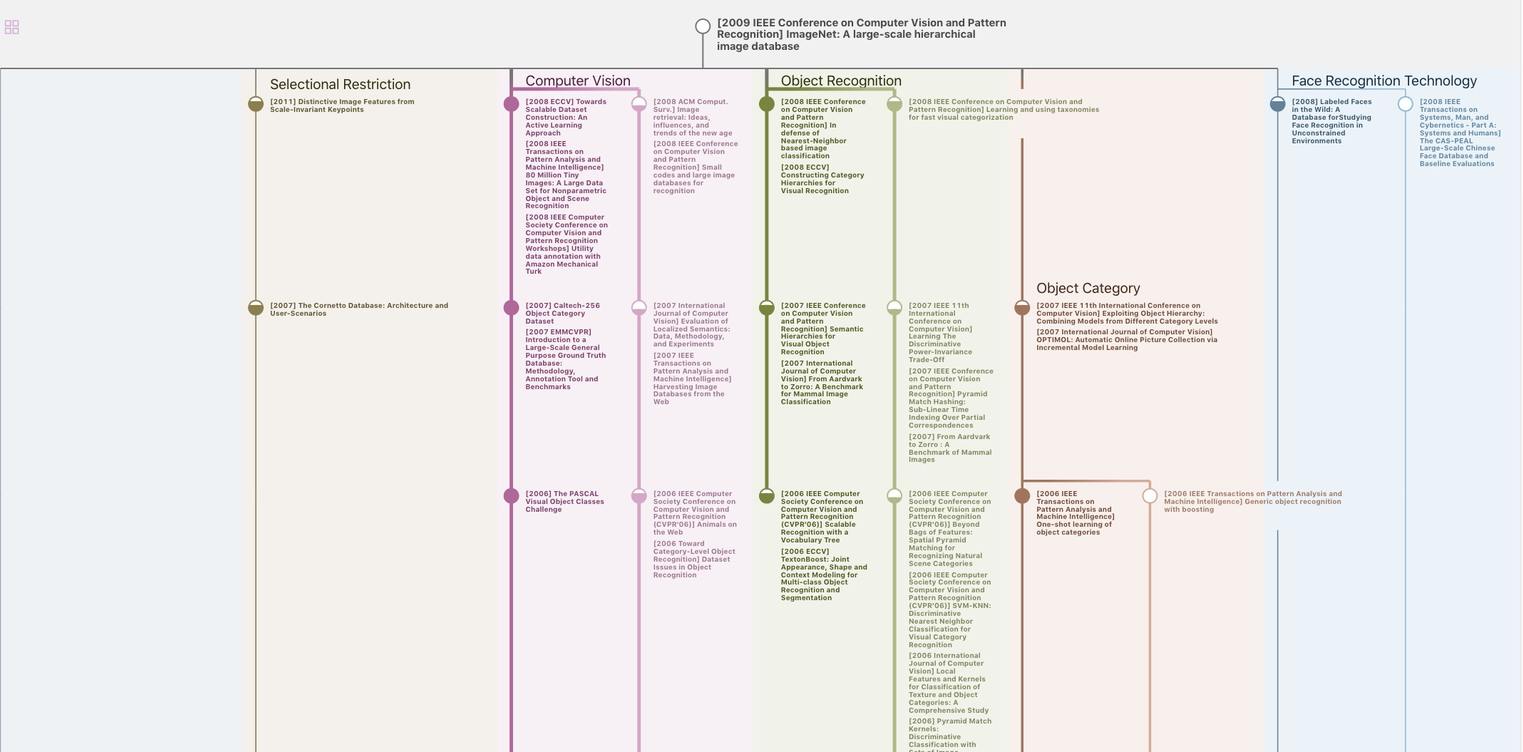
生成溯源树,研究论文发展脉络
Chat Paper
正在生成论文摘要