Time-Series Information and Unsupervised Learning of Representations
IEEE Transactions on Information Theory(2020)
摘要
Numerous control and learning problems face the situation where sequences of high-dimensional highly dependent data are available but no or little feedback is provided to the learner, which makes any inference rather challenging. To address this challenge, we formulate the following problem. Given a series of observations
$X_{0}, \ldots,X_{n}$
coming from a large (high-dimensional) space
$\mathcal X$
, find a representation function
$f$
mapping
$\mathcal X$
to a finite space
$\mathcal Y$
such that the series
$f(X_{0}), \ldots,f(X_{n})$
preserves as much information as possible about the original time-series dependence in
$X_{0}, \ldots,X_{n}$
. We show that, for stationary time series, the function
$f$
can be selected as the one maximizing a certain information criterion that we call time-series information. Some properties of this functions are investigated, including its uniqueness and consistency of its empirical estimates. Implications for the problem of optimal control are presented.
更多查看译文
关键词
Learning (artificial intelligence),unsupervised learning,time series analysis,mutual information
AI 理解论文
溯源树
样例
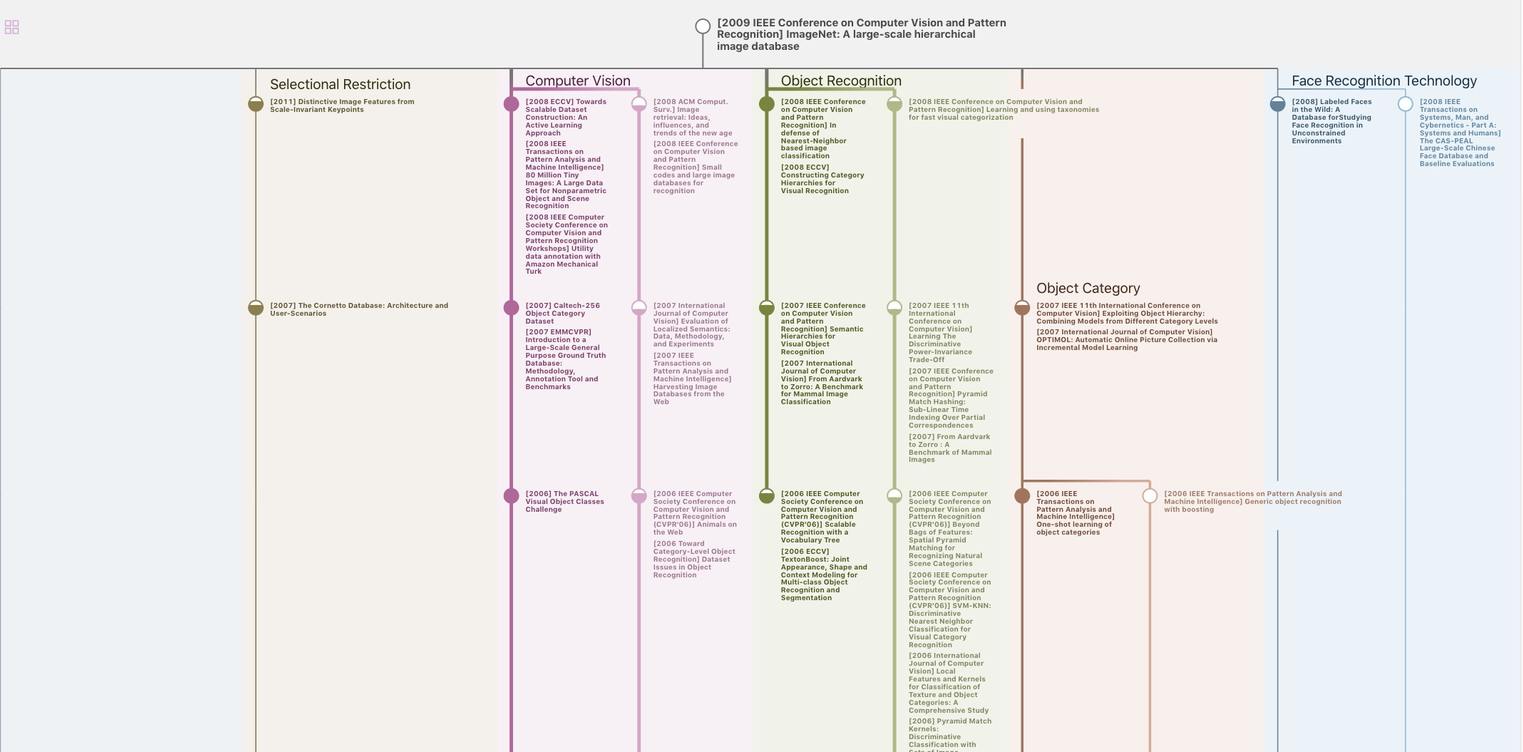
生成溯源树,研究论文发展脉络
Chat Paper
正在生成论文摘要