Optimization of Linearized Belief Propagation for Distributed Detection
IEEE Transactions on Communications(2020)
摘要
In this paper, we investigate distributed inference schemes, over binary-valued Markov random fields, which are realized by the belief propagation (BP) algorithm. We first show that a decision variable obtained by the BP algorithm in a network of distributed agents can be approximated by a linear fusion of all the local log-likelihood ratios. The proposed approach clarifies how the BP algorithm works, simplifies the statistical analysis of its behavior, and enables us to develop a performance optimization framework for the BP-based distributed inference systems. Next, we propose a blind learning-adaptation scheme to optimize the system performance when there is no information available a priori describing the statistical behavior of the wireless environment concerned. In addition, we propose a blind threshold adaptation method to guarantee a certain performance level in a BP-based distributed detection system. To clarify the points discussed, we design a novel linear-BP-based distributed spectrum sensing scheme for cognitive radio networks and illustrate the performance improvement obtained, over an existing BP-based detection method, via computer simulations.
更多查看译文
关键词
Approximation algorithms,Inference algorithms,Signal processing algorithms,Sensors,Optimization,Wireless sensor networks,Random variables
AI 理解论文
溯源树
样例
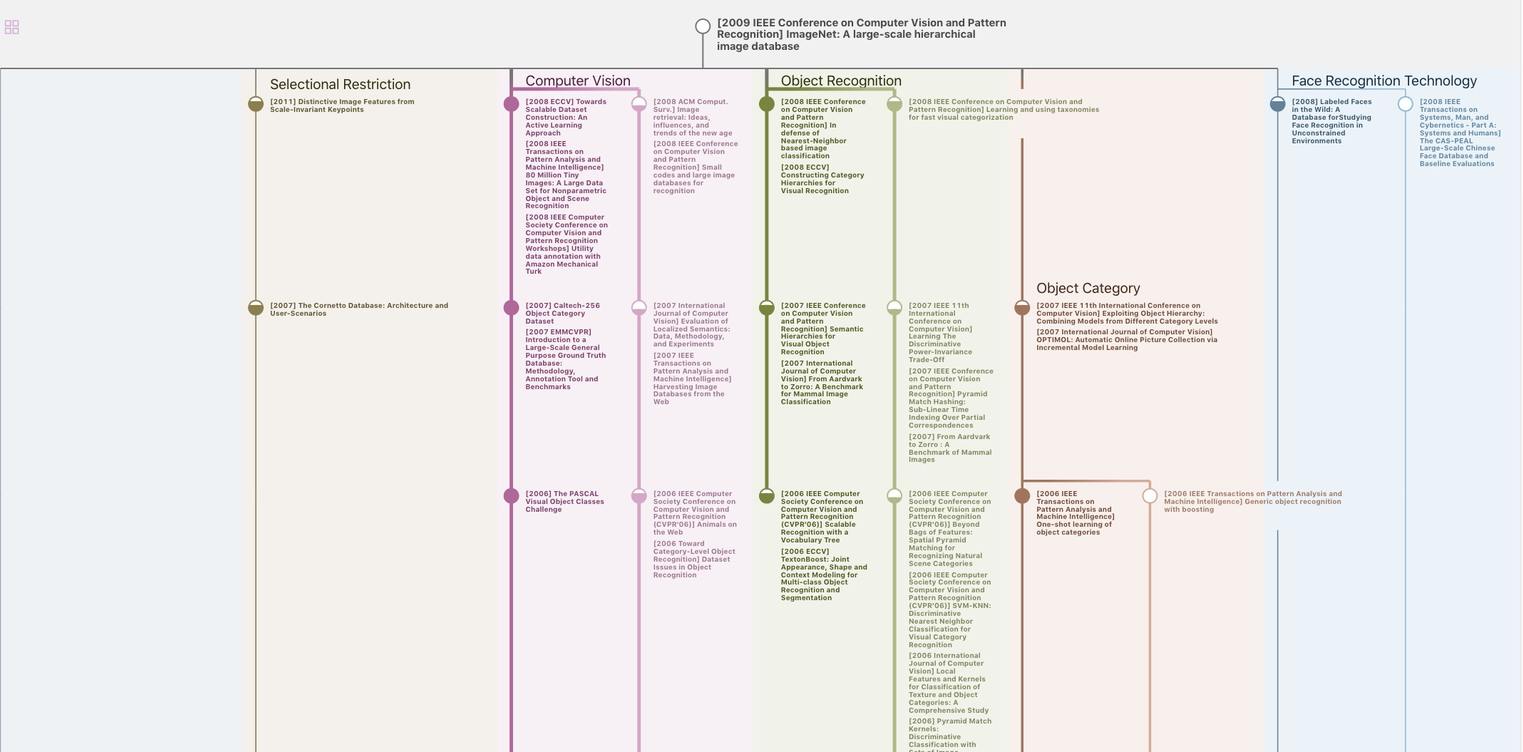
生成溯源树,研究论文发展脉络
Chat Paper
正在生成论文摘要